Machine learning for hypothesis generation in biology and medicine: exploring the latent space of neuroscience and developmental bioelectricity
DIGITAL DISCOVERY(2024)
摘要
Artificial intelligence is a powerful tool that could be deployed to accelerate the scientific enterprise. Here we address a major unmet need: use of existing scientific literature to generate novel hypotheses. We use a deep symmetry between the fields of neuroscience and developmental bioelectricity to evaluate a new tool, FieldSHIFT. FieldSHIFT is an in-context learning framework using a large language model to facilitate candidate scientific research from existing published studies, serving as a tool to generate hypotheses at scale. We release a new dataset for translating between the neuroscience and developmental bioelectricity domains and show how FieldSHIFT helps human scientists explore a latent space of papers that could exist, providing a rich field of suggested future research. We demonstrate the performance of FieldSHIFT for hypothesis generation relative to human-generated developmental biology research directions then test a key prediction of this model using bioinformatics, showing a surprising conservation of molecular mechanisms involved in cognitive behavior and developmental morphogenesis. By allowing scientists to rapidly explore symmetries and meta-parameters that exist in a corpus of scientific papers, we show how machine learning can potentiate human creativity and assist with one of the most interesting and crucial aspects of research: identifying insights from data and generating potential candidates for research agendas. FieldSHIFT uses in-context learning to translate neuroscience abstracts into developmental biology abstracts based on example concept mappings, creating new research hypotheses at scale.
更多查看译文
AI 理解论文
溯源树
样例
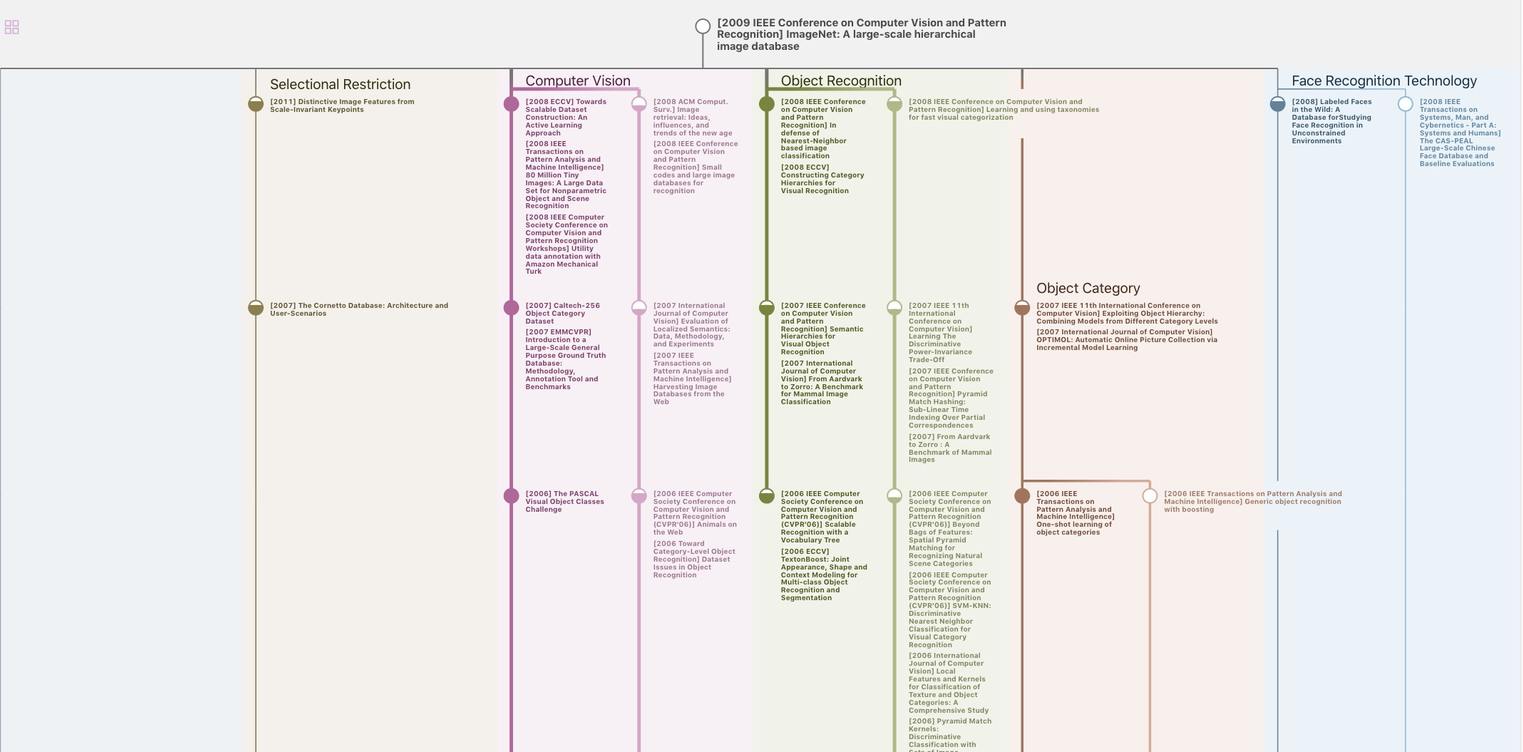
生成溯源树,研究论文发展脉络
Chat Paper
正在生成论文摘要