Multi-Viscosity Physics-Informed Neural Networks for Generating Ultra High Resolution Flow Field Data
INTERNATIONAL JOURNAL OF COMPUTATIONAL FLUID DYNAMICS(2023)
摘要
To address the limited generalisation ability issue of physics-informed neural networks, we propose a multi viscosity physics-informed neural networks (mu-PINNs), along with two tailored training strategies. By using mu-PINNs, we train the model once for a specific scenario and obtain flow field data with varying fluid viscosity (mu). To validate mu-PINNs, we conduct experiments on three 2D fluid flow scenarios, comparing the results to those computed by OpenFOAM and providing their relative $ \mathbb {L}_2 $ L2 error. The experiments demonstrate that mu-PINNs possess the capability of capturing the influence of viscosity on the output flow field data. Additionally, we compare the traditional scheme with the mixed-variable scheme. The memory usage and training time in mixed-variable scheme are 52.5% and 53.6% of that in traditional scheme, at the expense of lower accuracy. This comparison offers guidance for researchers in selecting an appropriate scheme. All code and data-sets are available on GitHub at https://github.com/Jensen1997/mu-PINNs.
更多查看译文
关键词
Physics-informed neural networks,deep learning,unsupervised learning,AI for science,fluid dynamics,incompressible laminar flows
AI 理解论文
溯源树
样例
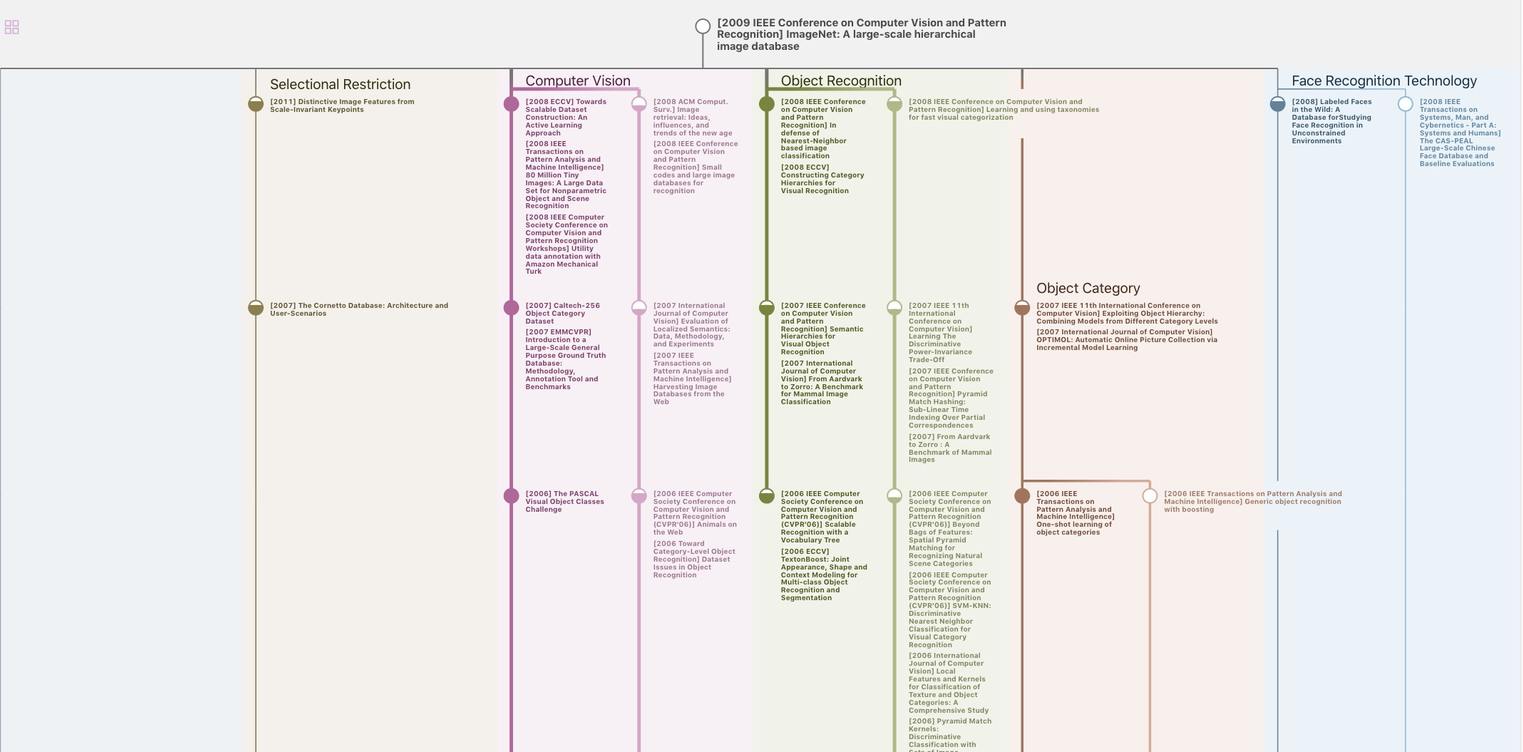
生成溯源树,研究论文发展脉络
Chat Paper
正在生成论文摘要