Full-Reference Image Quality Assessment Using Self-Attention and Multiscale Features
JOURNAL OF CIRCUITS SYSTEMS AND COMPUTERS(2024)
Abstract
In this paper, we construct a deep framework for full-reference image quality assessment (FR-IQA) by combining convolution and self-attention features; this approach effectively uses multiscale features to mimic the image evaluation in human eyes. We achieve the integration of information from local to global. First, convolutional neural network (CNN) and encoder of Swin transformer are used to extract multiscale paired features. Second, for each type of features, we fuse them after converting them to a fixed number of channels. For the fused features, we use the square of the difference between two pixel values at the corresponding channel positions to represent the features of the distortion degree. Then, we introduce a recurrent neural network (RNN) to capture the global features. Finally, for the two types of features, we use the full connection (FC) layer to regress two scores and then add the weights to compute the ultimate perceived score. By training and testing on publicly available FR-IQA datasets, experimental results further validate the superiority of our approach.
MoreTranslated text
Key words
Full-reference image quality assessment,convolution and self-attention features,recurrent neural network
AI Read Science
Must-Reading Tree
Example
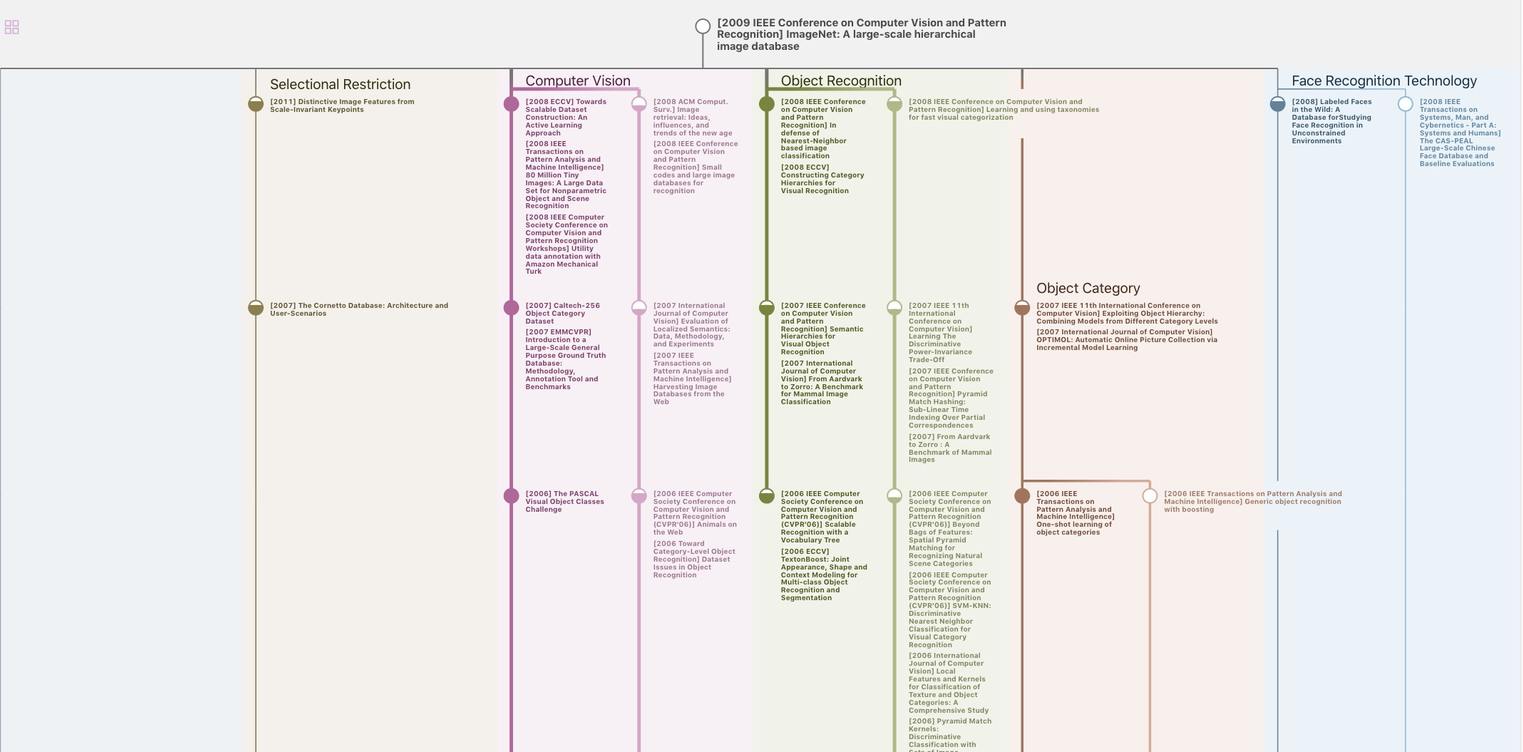
Generate MRT to find the research sequence of this paper
Chat Paper
Summary is being generated by the instructions you defined