Machine learning developed a PI3K/Akt pathway-related signature for predicting prognosis and drug sensitivity in ovarian cancer
AGING-US(2023)
摘要
Background: Ovarian cancer is one of the deadliest malignancies among females, generally having a poor prognosis. The PI3K/Akt pathway plays a vital role in the oncogenesis and progression of many types of cancer. Limited studies have fully clarified the role of PI3K/Akt pathway in the prognosis of ovarian cancer and its correlation with drug sensitivity. Methods: A prognostic PI3K/Akt pathway related signature (PRS) was constructed with 10 machine learning algorithms using TCGA, GSE14764, GSE26193, GSE26712, GSE63885 and GSE140082 datasets. Gaussian mixture and logistic regression were performed to identify the optimal models for classifying lymphatic and venous invasion. Results: The optimal prognostic PRS developed by Lasso + survivalSVM algorithm acted as an independent risk factor for overall survival (OS) of ovarian cancer patients and had a good performance in evaluating OS rate of ovarian cancer patients. Significant correlation was obtained between PRS-based risk score and Immune score, ESTIMATE score, immune cells and cancer-related hallmarks. Low risk score indicated a lower immune escape score, TIDE score, and higher PD1&CTLA4 immunophenoscore in ovarian cancer. Moreover, PRS-based risk score acted as an indicator for drug sensitivity in the immunotherapy and chemotherapy of ovarian cancer patients. Conclusions: All in all, our study developed a prognostic PRS showing powerful and good performance in predicting clinical outcome of ovarian cancer patients. PRS could serve as an indicator for drug sensitivity in the chemotherapy and immunotherapy.
更多查看译文
关键词
immunotherapy,prognostic signature,ovarian cancer,machine learning,PI3K/Akt pathway
AI 理解论文
溯源树
样例
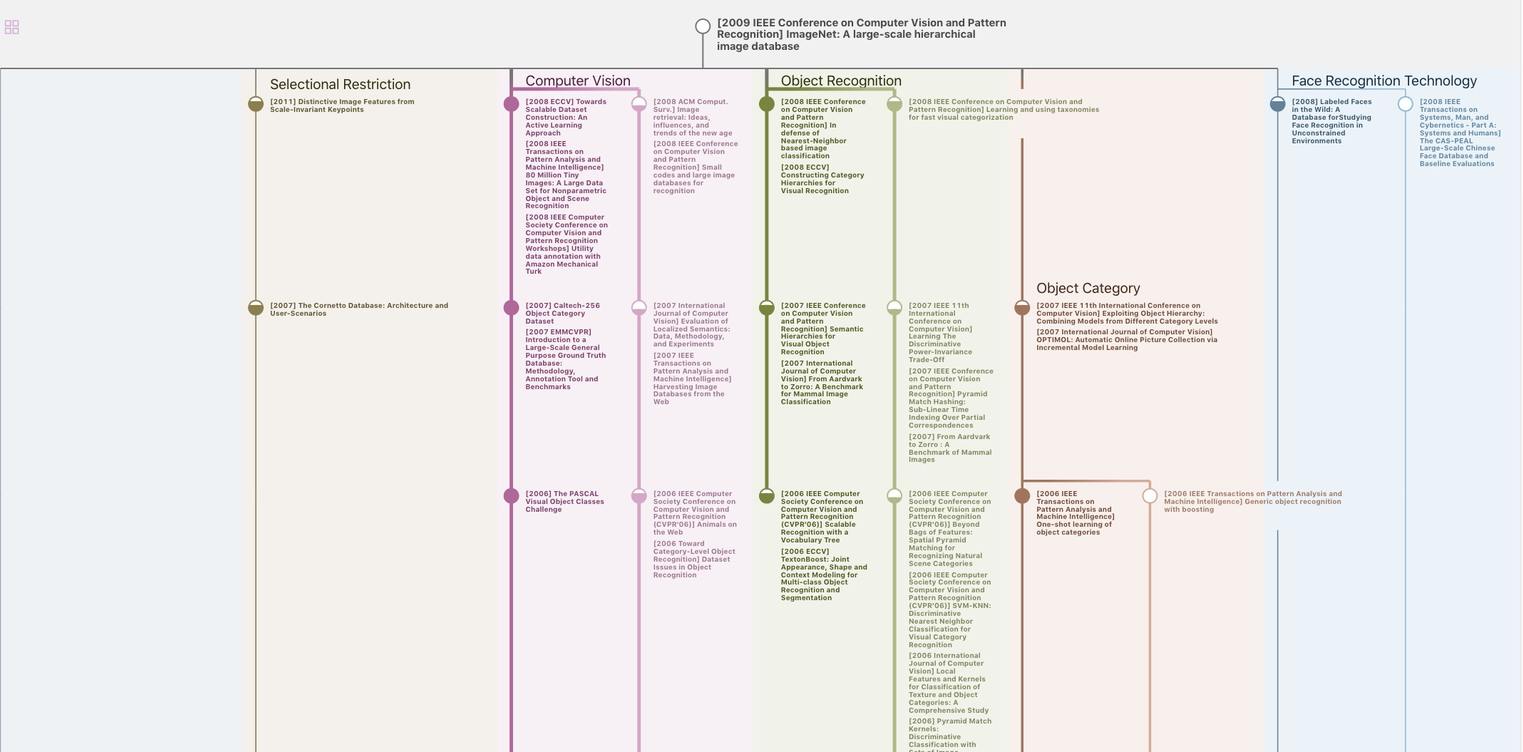
生成溯源树,研究论文发展脉络
Chat Paper
正在生成论文摘要