CLOVER: Contrastive Learning for Onboard Vision-Enabled Robotics
JOURNAL OF SPACECRAFT AND ROCKETS(2023)
Abstract
Current deep-learning models employed by the planetary science community are constrained by a dearth of annotated training data for planetary images. Current models also frequently suffer from inductive bias due to domain shifts when using the same model on data obtained from different spacecraft or different time periods. Moreover, power and compute constraints preclude state-of-the-art vision models from being implemented on robotic spacecraft. In this research, we propose a self-supervised learning (SSL) framework that leverages contrastive learning techniques to improve upon state-of-the-art performance on several published Mars computer vision benchmarks. Our SSL framework enables models to be trained using fewer labels, generalize well to different tasks, and achieve higher computational efficiency. Results on published Mars computer vision benchmarks show that contrastive pretraining outperforms plain supervised learning by 2-10%. We further investigate the importance of dataset heterogeneity in mixed-domain contrastive pretraining. Using self-supervised distillation, we were also able to train a compact ResNet-18 student model to achieve better accuracy than its ResNet-152 teacher model while having 5.2 times fewer parameters. We expect that these SSL techniques will be relevant to the planning of future robotic missions, and remote sensing identification of target destinations with high scientific value.
MoreTranslated text
Key words
Robotics,Planets,Convolutional Neural Network,Computer Vision,Planetary Science and Exploration,Uncrewed Spacecraft,Remote Sensing and Applications,Representation Learning,Mars Science Laboratory,Mars Exploration Rover
AI Read Science
Must-Reading Tree
Example
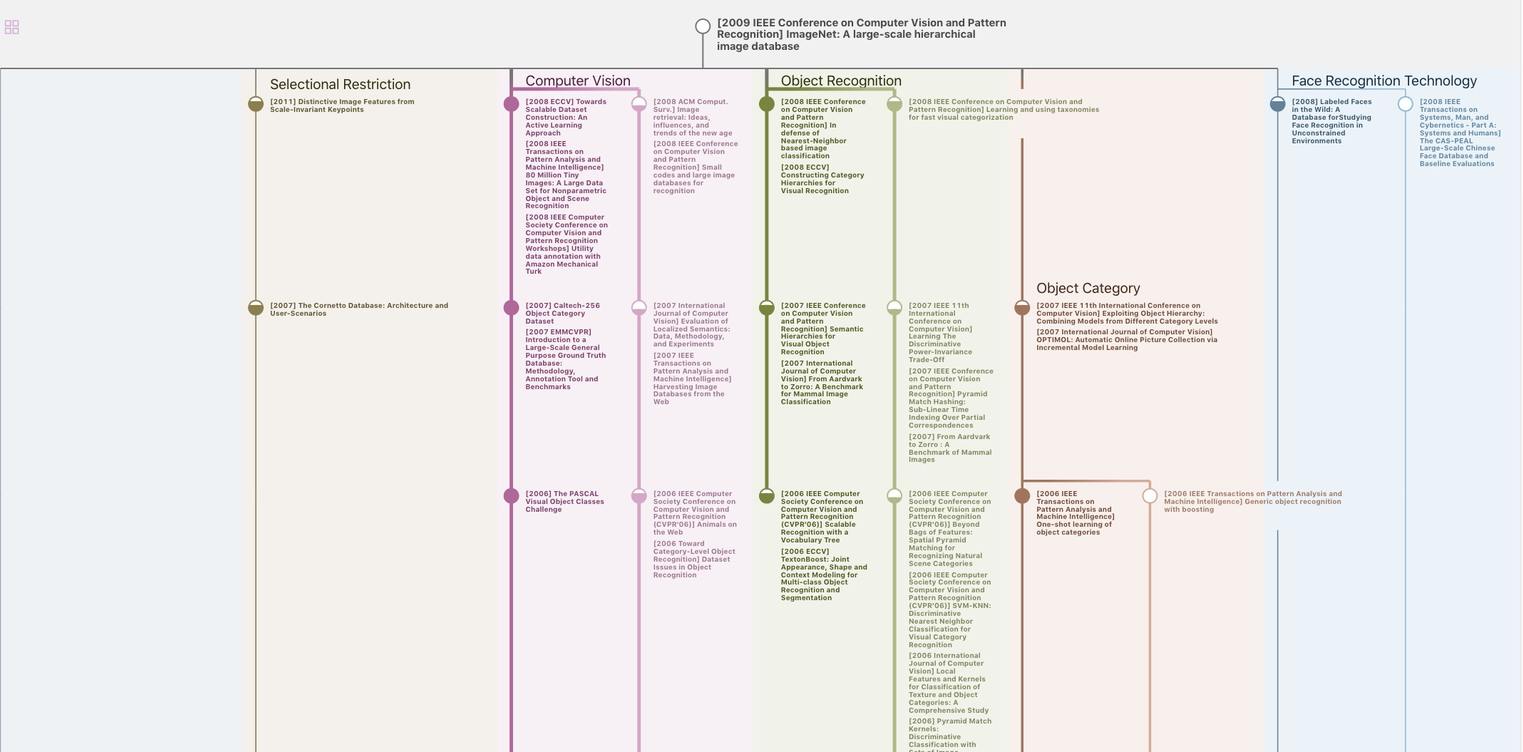
Generate MRT to find the research sequence of this paper
Chat Paper
Summary is being generated by the instructions you defined