Applicability Evaluation of Deep Learning-Based Object Detection for Coastal Debris Monitoring: A Comparative Study of YOLOv8 and RT-DETR
KOREAN JOURNAL OF REMOTE SENSING(2023)
摘要
Coastal debris has emerged as a salient issue due to its adverse effects on coastal aesthetics, ecological systems, and human health. In pursuit of effective countermeasures, the present study delineated the construction of a specialized image dataset for coastal debris detection and embarked on a comparative analysis between two paramount real-time object detection algorithms, YOLOv8 and RTDETR. Rigorous assessments of robustness under multifarious conditions were instituted, subjecting the models to assorted distortion paradigms. YOLOv8 manifested a detection accuracy with a mean Average Precision (mAP) value ranging from 0.927 to 0.945 and an operational speed between 65 and 135 Frames Per Second (FPS). Conversely, RT-DETR yielded an mAP value bracket of 0.917 to 0.918 with a detection velocity spanning 40 to 53 FPS. While RT-DETR exhibited enhanced robustness against color distortions, YOLOv8 surpassed resilience under other evaluative criteria. The implications derived from this investigation are poised to furnish pivotal directives for algorithmic selection in the practical deployment of marine debris monitoring systems.
更多查看译文
关键词
Coastal debris,Object detection,Drone,Deep learning
AI 理解论文
溯源树
样例
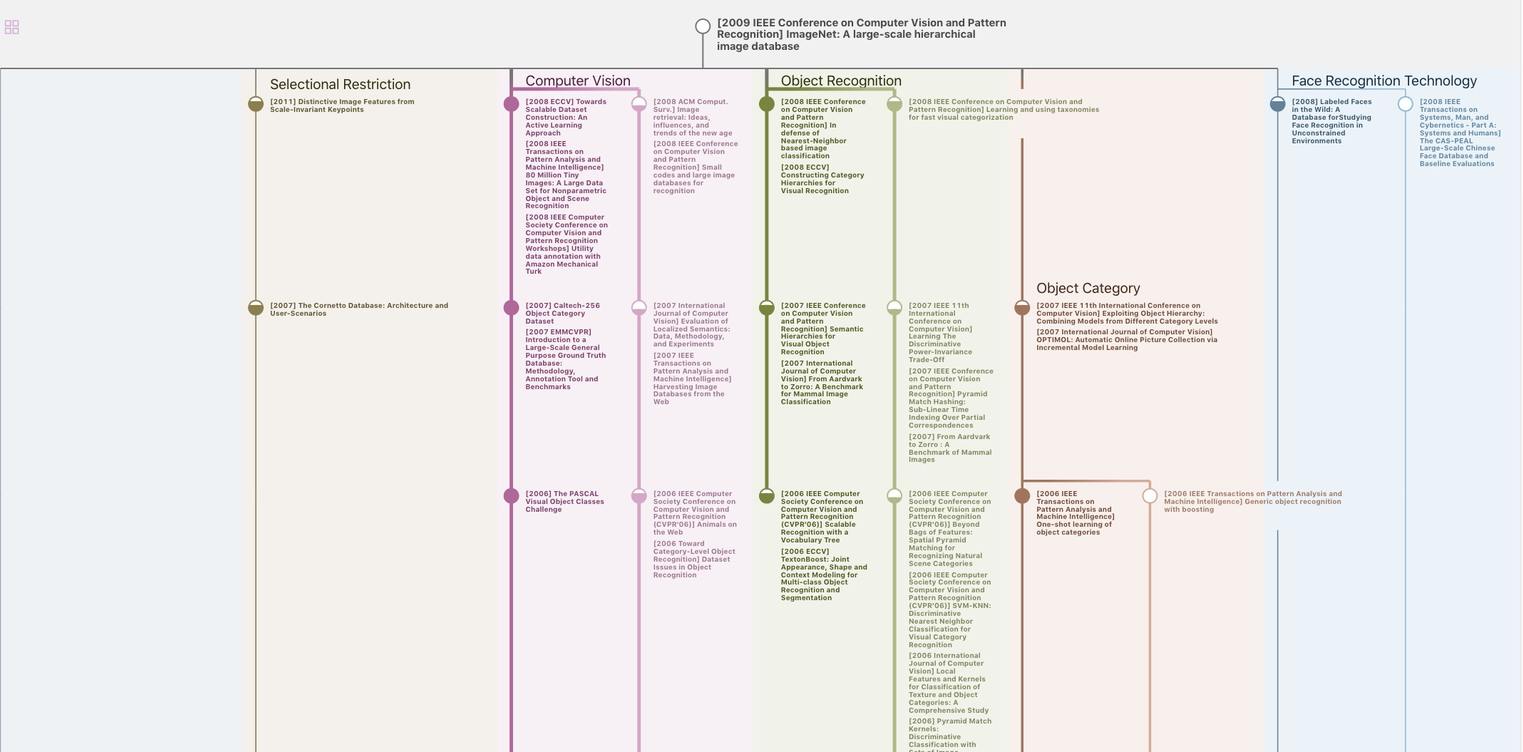
生成溯源树,研究论文发展脉络
Chat Paper
正在生成论文摘要