StarkML: application of machine learning to overcome lack of data on electron-impact broadening parameters
MONTHLY NOTICES OF THE ROYAL ASTRONOMICAL SOCIETY(2023)
Abstract
Parameters of electron-impact (Stark) broadening and shift of spectral lines are of key importance in various studies of plasma spectroscopy and astrophysics. To overcome the lack of accurately known Stark parameters, we developed a machine learning approach for predicting Stark parameters of neutral atoms' lines. By implementing a data pre-processing routine and explicitly testing models' predictive ability and generalizability, we achieve a high level of accuracy in parameters prediction as well as physically meaningful temperature dependence. The applicability of the results is demonstrated by the case of low-temperature plasma diagnostics. The developed model is readily accessible for predicting desired Stark parameters.
MoreTranslated text
Key words
atomic data,line: profiles,plasmas,software: data analysis
AI Read Science
Must-Reading Tree
Example
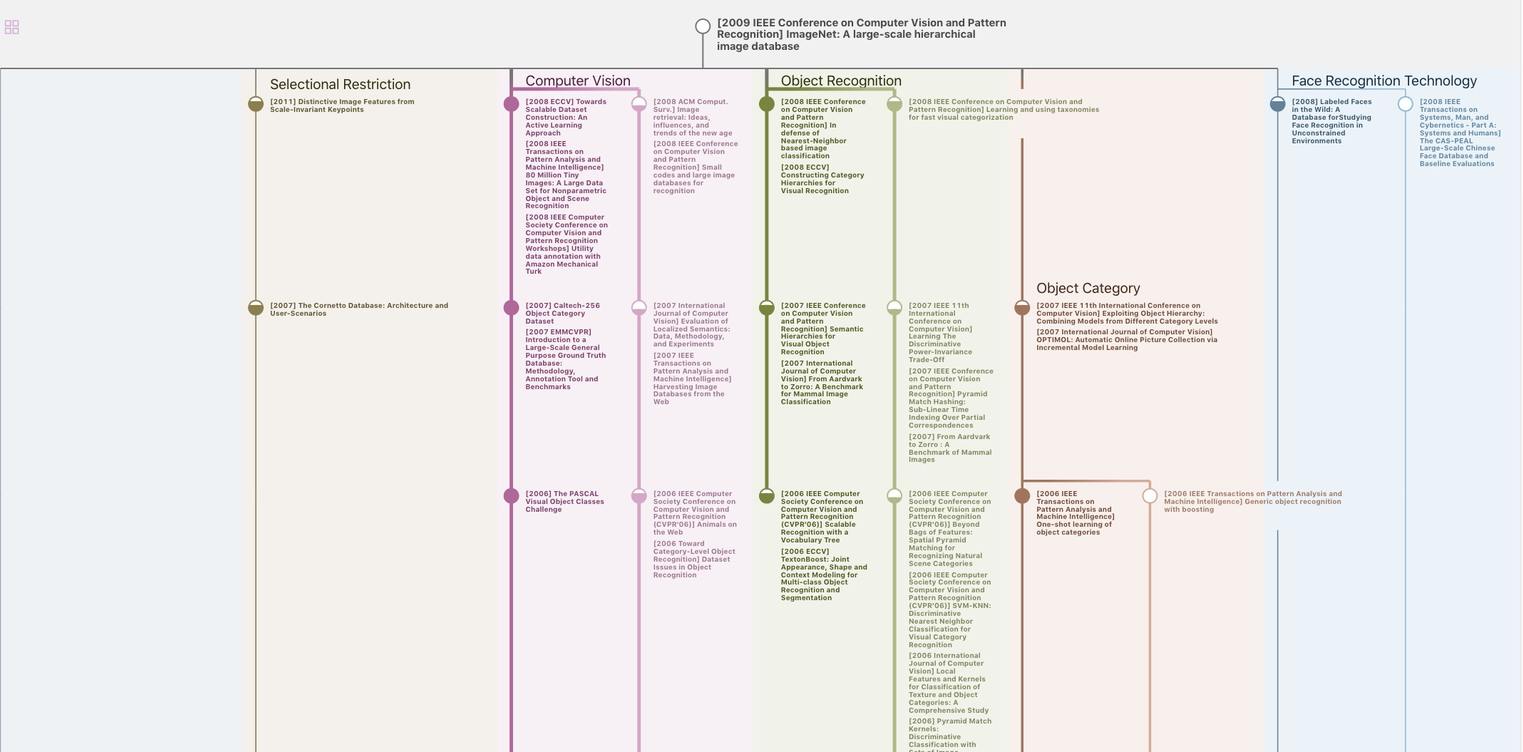
Generate MRT to find the research sequence of this paper
Chat Paper
Summary is being generated by the instructions you defined