Accelerating FEM-Based Corrosion Predictions Using Machine Learning
JOURNAL OF THE ELECTROCHEMICAL SOCIETY(2024)
摘要
Atmospheric corrosion of metallic parts is a widespread materials degradation phenomena that is challenging to predict given its dependence on many factors (e.g. environmental, physiochemical, and part geometry). For materials with long expected service lives, accurately predicting the degree to which corrosion will degrade part performance is especially difficult due to the stochastic nature of corrosion damage spread across years or decades of service. The Finite Element Method (FEM) is a computational technique capable of providing accurate estimates of corrosion rate by numerically solving complex differential Eqs. characterizing this phenomena. Nevertheless, given the iterative nature of FEM and the computational expense required to solve these complex equations, FEM is ill-equipped for an efficient exploration of the design space to identify factors that accelerate or deter corrosion, despite its accuracy. In this work, a machine learning based surrogate model capable of providing accurate predictions of corrosion with significant computational savings is introduced. Specifically, this work leverages AdaBoosted Decision trees to provide an accurate estimate of corrosion current per width given different values of temperature, water layer thickness, molarity of the solution, and the length of the cathode for a galvanic couple of aluminum and stainless steel. Novel protocol for extracting knowledge from previously performed Finite Element corrosion simulations using machine learning.Obtain accurate predictions for corrosion current 5 orders of magnitude faster than Finite Element simulations.Accurate machine learning based model capable of performing an effective and efficient search over the multi-dimensional input space to identify areas/zones where corrosion is more (or less) noticeable.
更多查看译文
关键词
Machine Learning,Galvanic corrosion,Marine Corrosion,Atmospheric corrosion
AI 理解论文
溯源树
样例
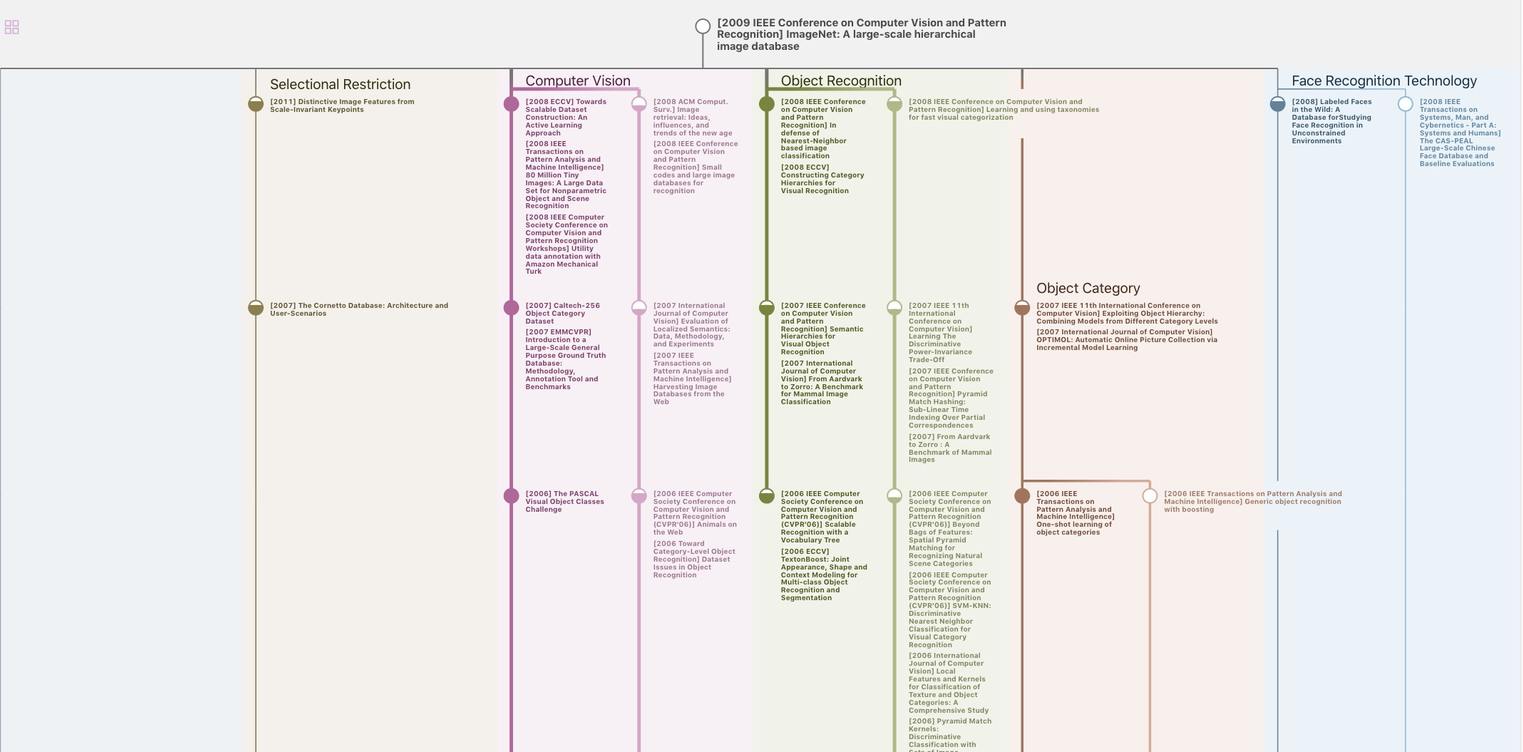
生成溯源树,研究论文发展脉络
Chat Paper
正在生成论文摘要