State of health estimation of LIB based on discharge section with multi-model combined
HELIYON(2024)
摘要
Accurate estimation of a battery's state of health (SOH) is essential in battery management systems (BMS). This study considers a complete analysis of combining incremental capacity (IC), differential thermal voltammetry (DTV), and differential temperature (DT) for SOH prediction in cases of discharge. Initially, the IC, DTV, and DT curves were derived from the current, voltage, and temperature datasets, and these curves underwent smoothing through the application of Lowess and Gaussian techniques. Subsequently, discerning healthy features were identified within the domains where the curve exhibited substantial phase transitions. Utilizing Pearson correlation analysis, features exhibiting the utmost correlation with battery capacity degradation were singled out. Finally, the state-of-health (SOH) prediction model was constructed using a bidirectional long short-term memory (BILSTM) neural network. Two datasets were used to validate the model, and the experimental results demonstrated that the SOH prediction had a root mean square error (RMSE) below 1.2% and mean absolute error (MAE) below 1%, which verified the feasibility and accuracy. This approach quantifies the internal electrochemical reactions of a battery using externally measured data, further enabling early SOH predictions.
更多查看译文
关键词
Lithium -ion battery,State of health,Bidirectional long short -term memory network,Incremental capacity analysis,Differential thermal voltammetry analysis,Differential temperature analysis
AI 理解论文
溯源树
样例
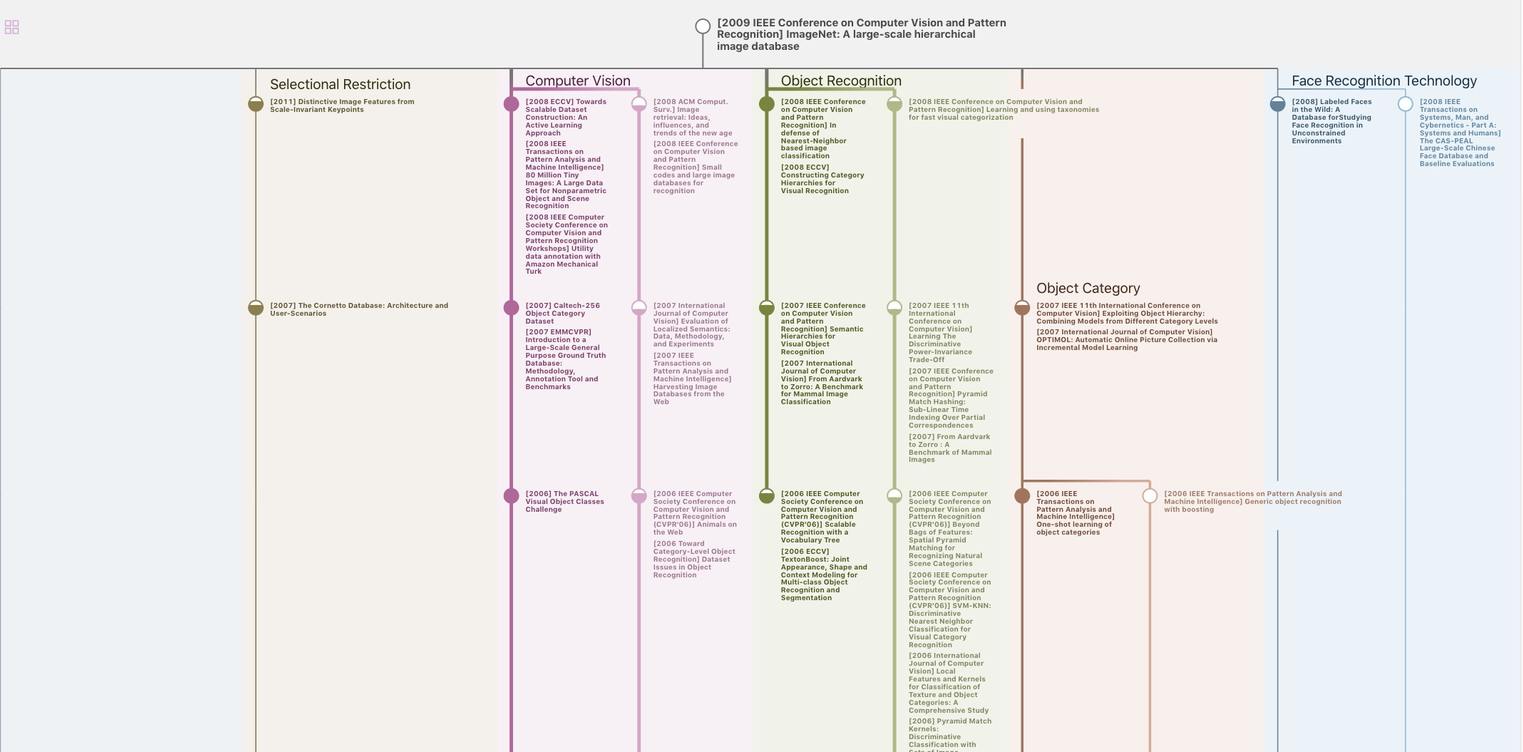
生成溯源树,研究论文发展脉络
Chat Paper
正在生成论文摘要