Employing MS-UNets Networks for Multiscale 3-D Gravity Data Inversion: A Case Study in the Nordkapp Basin, Barents Sea
IEEE TRANSACTIONS ON GEOSCIENCE AND REMOTE SENSING(2024)
摘要
Salt domes are very important in hydrocarbon exploration and identification of potential drilling hazards. While seismic data are indispensable for detailed subsurface imaging, especially in delineating the geometry and properties of salt bodies and their boundaries, gravity inversion provides an additional layer of data by exploiting the density differential. However, traditional methodologies for tackling this problem are complicated by the ill-posedness of the inverse problems. The alternative approach to gravity image is based on machine learning (ML) algorithms. Despite the appealing attributes of convolutional neural networks (CNNs), they are not exempt from limitations, including diminished precision in pinpointing geological features, complications in managing the varying scales of geological structures, and inefficiencies in processing voluminous, high-dimensional data. These deficits can be mitigated by the proposed multiscale functional multiscale UNets (MS-UNets) network, which, through integration with squeeze-and-excitation (S-E) and strip pooling (S-P) modules, are designed to enhance the capture of detailed information about salt domes. These networks were subjected to rigorous testing using both synthetic and real gravity data, showcasing their robustness across diverse scenarios. This testing highlighted their significant potential for applications in geophysical data interpretation, structural modeling, and inversion processes.
更多查看译文
关键词
3-D inversion,gravity and gravity gradiometry,multiscale UNets (MS-UNets)
AI 理解论文
溯源树
样例
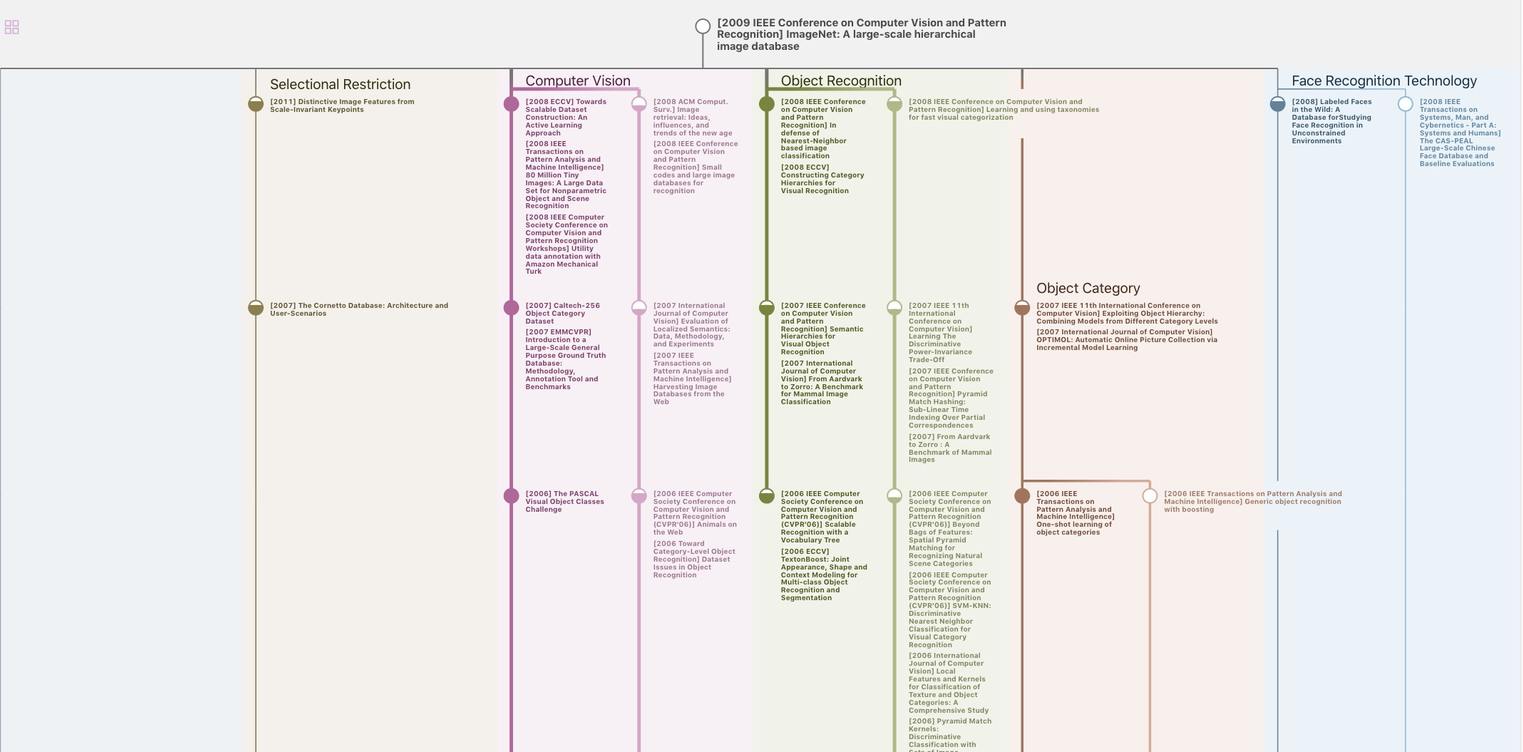
生成溯源树,研究论文发展脉络
Chat Paper
正在生成论文摘要