Decomposed Deep Q-Network for Coherent Task-Oriented Dialogue Policy Learning
IEEE-ACM TRANSACTIONS ON AUDIO SPEECH AND LANGUAGE PROCESSING(2024)
摘要
Reinforcement learning (RL) has emerged as a key technique for designing dialogue policies. However, action space inflation in dialogue tasks has led to a heavy decision burden and incoherence problems for dialogue policies. In this paper, we propose a novel decomposed deep Q-network (D2Q) that exploits the natural structure of dialogue actions to perform decomposition on Q-function, realizing efficient and coherent dialogue policy learning. Instead of directly evaluating the Q-function, it consists of two separate estimators, one for the abstract action-value functions and the other for the specific action-value functions, both sharing a common feature layer. The abstract action-value function determines the speech act of the system action, while the specific action-value function focuses on the concrete action. This structure establishes a logical relationship between the user and the system on speech actions, avoiding the problem of incoherence. Moreover, the abstract action-value function shields unreasonable specific actions in the inflated action space, reducing the decision complexity. Our results show that the problem of incoherence is prevalent in existing approaches, which significantly impacts the efficiency and quality of dialogue policy learning. Our D2Q architecture alleviates this problem and performs significantly better than competitive baselines in both evaluated and human experiments. Further experiments validate the generality of our method. It can be easily extended to other RL-based dialogue policy approaches.
更多查看译文
关键词
Reinforcement learning,Periodic structures,dialogue policy,action space inflation,incoherence problem
AI 理解论文
溯源树
样例
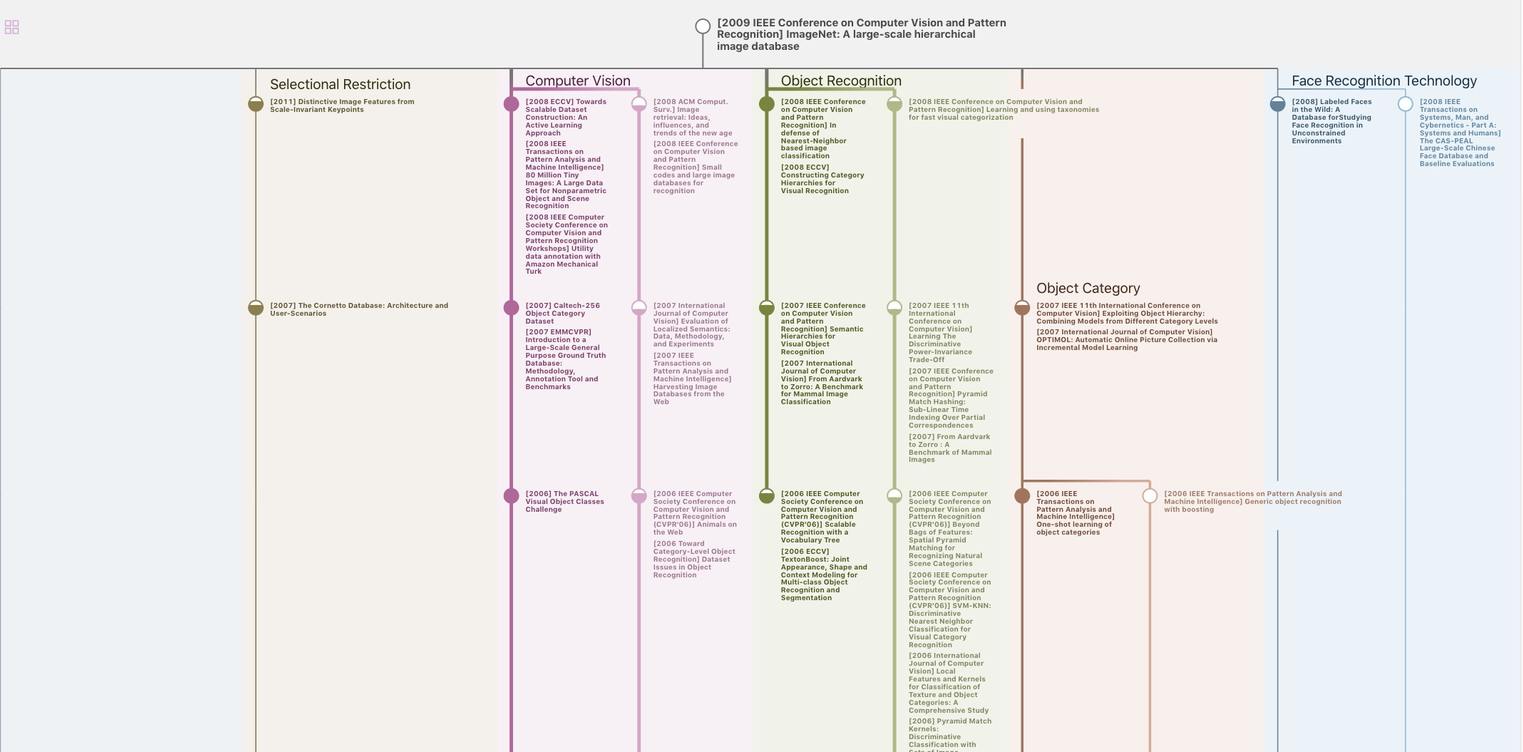
生成溯源树,研究论文发展脉络
Chat Paper
正在生成论文摘要