FedFit: Server Aggregation Through Linear Regression in Federated Learning
IEEE ACCESS(2024)
摘要
We present a conceptually novel framework for Federated Learning (FL) called FedFit for a flexible solver to address FL problems. The FedFit framework consists of two components: model compression to upload a local model from a client to the server and the reconstruction of the compressed local model in the server. Clients upload a compressed local model using a "key" shared with the server to formulate the server aggregation in the FL as linear regression. Therefore, the parameters of the global model are updated through a linear regression solver in the server while naturally contributing to reducing upload costs from clients to the server. Thanks to our framework design, the server can flexibly utilize various established linear regression techniques to address some open problems of FL by considering server aggregation from a different perspective-linear regression. As an example of the broad applicability of our concept, we demonstrate the effectiveness of robust regression and LASSO regression implemented on FedFit, which can alleviate vulnerability issues against attacks on the global model from collapsed clients and introduce sparsity to the global model toward the reduction in model size, respectively.
更多查看译文
关键词
Federated learning,linear regression,communication protocol
AI 理解论文
溯源树
样例
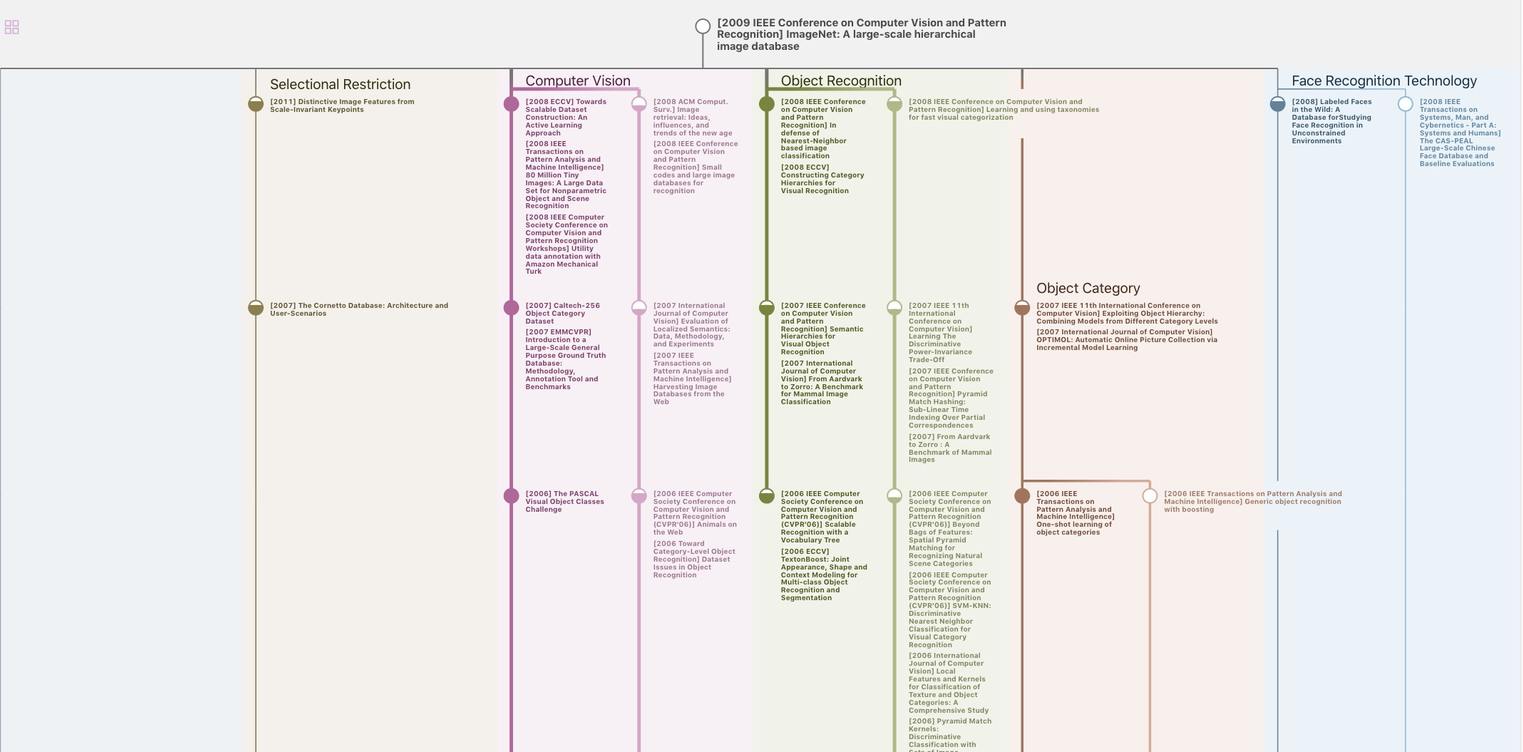
生成溯源树,研究论文发展脉络
Chat Paper
正在生成论文摘要