DoorINet: A Deep-Learning Inertial Framework for Door-Mounted IoT Applications
CoRR(2024)
摘要
Many Internet of Things applications utilize low-cost, micro,
electro-mechanical inertial sensors. A common task is orientation estimation.
To tackle such a task, attitude and heading reference system algorithms are
applied. Relying on the gyroscope readings, the accelerometer readings are used
to update the attitude angles, and magnetometer measurements are utilized to
update the heading angle. In indoor environments, magnetometers suffer from
interference that degrades their performance. This mainly influences
applications focused on estimating the heading angle like finding the heading
angle of a closet or fridge door. To circumvent such situations, we propose
DoorINet, an end-to-end deep-learning framework to calculate the heading angle
from door-mounted, low-cost inertial sensors without using magnetometers. To
evaluate our approach, we record a unique dataset containing 391 minutes of
accelerometer and gyroscope measurements and corresponding ground-truth heading
angle. We show that our proposed approach outperforms commonly used, model
based approaches and data-driven methods.
更多查看译文
AI 理解论文
溯源树
样例
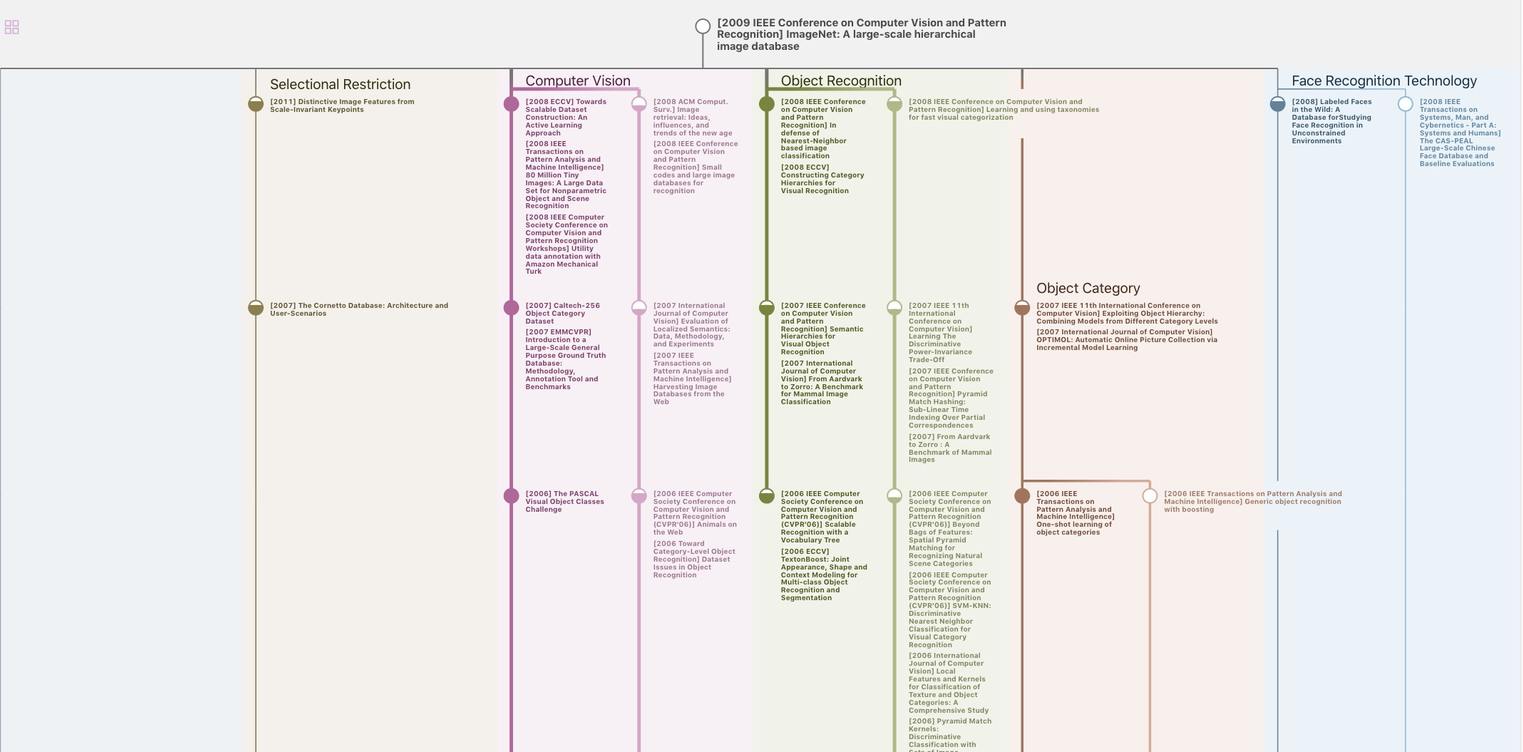
生成溯源树,研究论文发展脉络
Chat Paper
正在生成论文摘要