f-MICL: Understanding and Generalizing InfoNCE-based Contrastive Learning
CoRR(2024)
摘要
In self-supervised contrastive learning, a widely-adopted objective function
is InfoNCE, which uses the heuristic cosine similarity for the representation
comparison, and is closely related to maximizing the Kullback-Leibler
(KL)-based mutual information. In this paper, we aim at answering two
intriguing questions: (1) Can we go beyond the KL-based objective? (2) Besides
the popular cosine similarity, can we design a better similarity function? We
provide answers to both questions by generalizing the KL-based mutual
information to the f-Mutual Information in Contrastive Learning (f-MICL)
using the f-divergences. To answer the first question, we provide a wide
range of f-MICL objectives which share the nice properties of InfoNCE (e.g.,
alignment and uniformity), and meanwhile result in similar or even superior
performance. For the second question, assuming that the joint feature
distribution is proportional to the Gaussian kernel, we derive an f-Gaussian
similarity with better interpretability and empirical performance. Finally, we
identify close relationships between the f-MICL objective and several popular
InfoNCE-based objectives. Using benchmark tasks from both vision and natural
language, we empirically evaluate f-MICL with different f-divergences on
various architectures (SimCLR, MoCo, and MoCo v3) and datasets. We observe that
f-MICL generally outperforms the benchmarks and the best-performing
f-divergence is task and dataset dependent.
更多查看译文
AI 理解论文
溯源树
样例
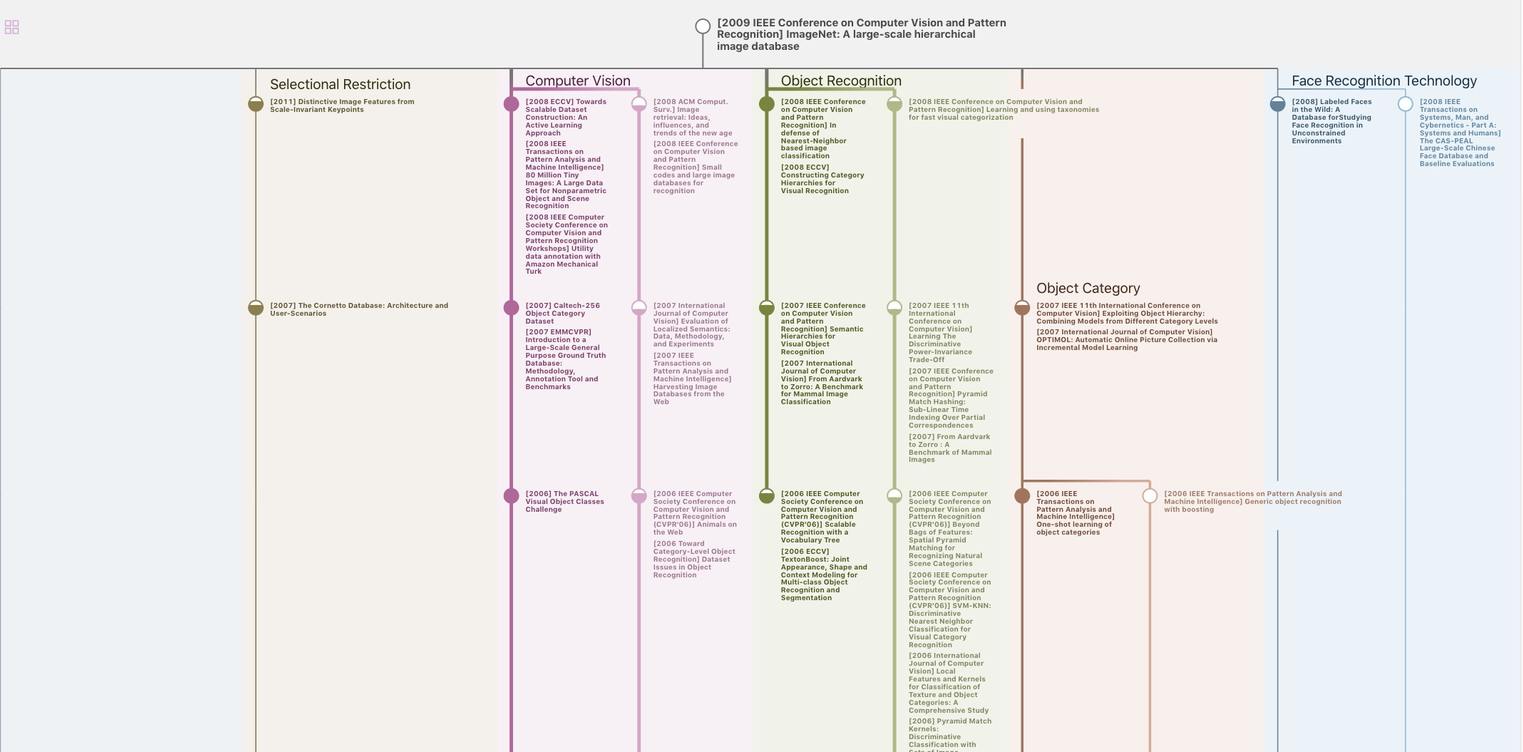
生成溯源树,研究论文发展脉络
Chat Paper
正在生成论文摘要