Short-Form Videos and Mental Health: A Knowledge-Guided Neural Topic Model
arxiv(2024)
Abstract
While short-form videos head to reshape the entire social media landscape,
experts are exceedingly worried about their depressive impacts on viewers, as
evidenced by medical studies. To prevent widespread consequences, platforms are
eager to predict these videos' impact on viewers' mental health. Subsequently,
they can take intervention measures, such as revising recommendation algorithms
and displaying viewer discretion. Nevertheless, applicable predictive methods
lack relevance to well-established medical knowledge, which outlines clinically
proven external and environmental factors of depression. To account for such
medical knowledge, we resort to an emergent methodological discipline, seeded
Neural Topic Models (NTMs). However, existing seeded NTMs suffer from the
limitations of single-origin topics, unknown topic sources, unclear seed
supervision, and suboptimal convergence. To address those challenges, we
develop a novel Knowledge-guided Multimodal NTM to predict a short-form video's
depressive impact on viewers. Extensive empirical analyses using TikTok and
Douyin datasets prove that our method outperforms state-of-the-art benchmarks.
Our method also discovers medically relevant topics from videos that are linked
to depressive impact. We contribute to IS with a novel video analytics method
that is generalizable to other video classification problems. Practically, our
method can help platforms understand videos' mental impacts, thus adjusting
recommendations and video topic disclosure.
MoreTranslated text
AI Read Science
Must-Reading Tree
Example
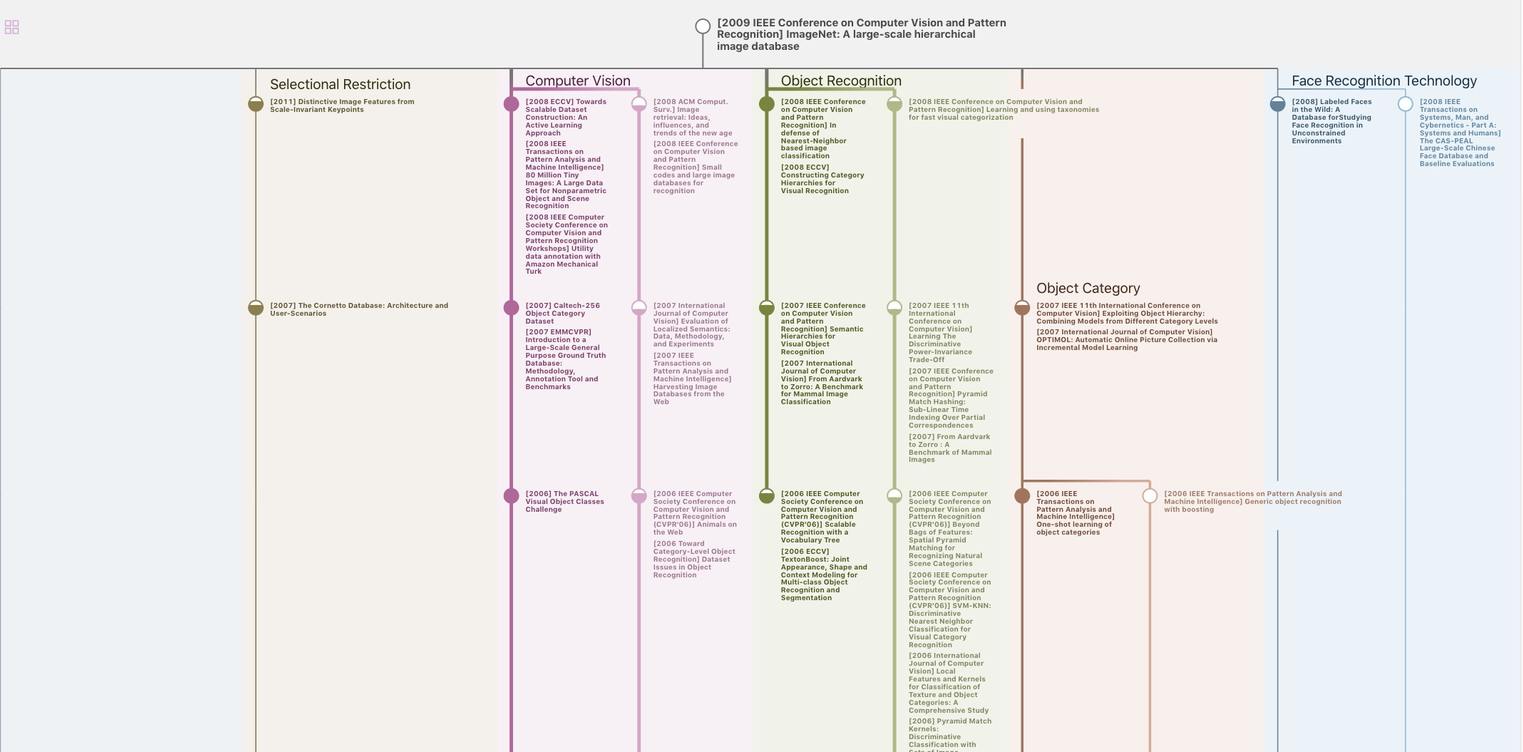
Generate MRT to find the research sequence of this paper
Chat Paper
Summary is being generated by the instructions you defined