Domain Adaptation for Contrastive Audio-Language Models
CoRR(2024)
摘要
Audio-Language Models (ALM) aim to be general-purpose audio models by
providing zero-shot capabilities at test time. The zero-shot performance of ALM
improves by using suitable text prompts for each domain. The text prompts are
usually hand-crafted through an ad-hoc process and lead to a drop in ALM
generalization and out-of-distribution performance. Existing approaches to
improve domain performance, like few-shot learning or fine-tuning, require
access to annotated data and iterations of training. Therefore, we propose a
test-time domain adaptation method for ALMs that does not require access to
annotations. Our method learns a domain vector by enforcing consistency across
augmented views of the testing audio. We extensively evaluate our approach on
12 downstream tasks across domains. With just one example, our domain
adaptation method leads to 3.2
improvement. After adaptation, the model still retains the generalization
property of ALMs.
更多查看译文
AI 理解论文
溯源树
样例
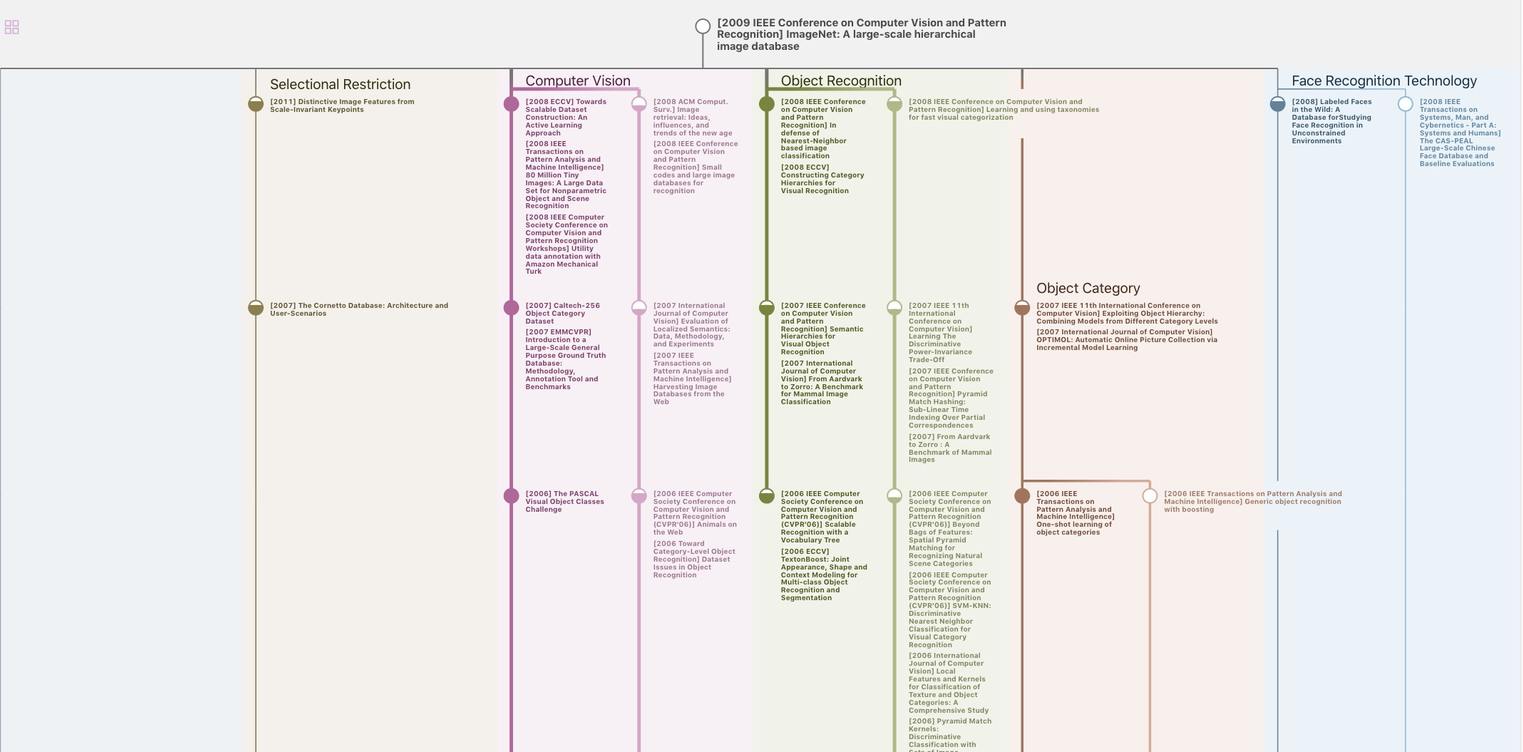
生成溯源树,研究论文发展脉络
Chat Paper
正在生成论文摘要