Dataset Clustering for Improved Offline Policy Learning
CoRR(2024)
摘要
Offline policy learning aims to discover decision-making policies from
previously-collected datasets without additional online interactions with the
environment. As the training dataset is fixed, its quality becomes a crucial
determining factor in the performance of the learned policy. This paper studies
a dataset characteristic that we refer to as multi-behavior, indicating that
the dataset is collected using multiple policies that exhibit distinct
behaviors. In contrast, a uni-behavior dataset would be collected solely using
one policy. We observed that policies learned from a uni-behavior dataset
typically outperform those learned from multi-behavior datasets, despite the
uni-behavior dataset having fewer examples and less diversity. Therefore, we
propose a behavior-aware deep clustering approach that partitions
multi-behavior datasets into several uni-behavior subsets, thereby benefiting
downstream policy learning. Our approach is flexible and effective; it can
adaptively estimate the number of clusters while demonstrating high clustering
accuracy, achieving an average Adjusted Rand Index of 0.987 across various
continuous control task datasets. Finally, we present improved policy learning
examples using dataset clustering and discuss several potential scenarios where
our approach might benefit the offline policy learning community.
更多查看译文
AI 理解论文
溯源树
样例
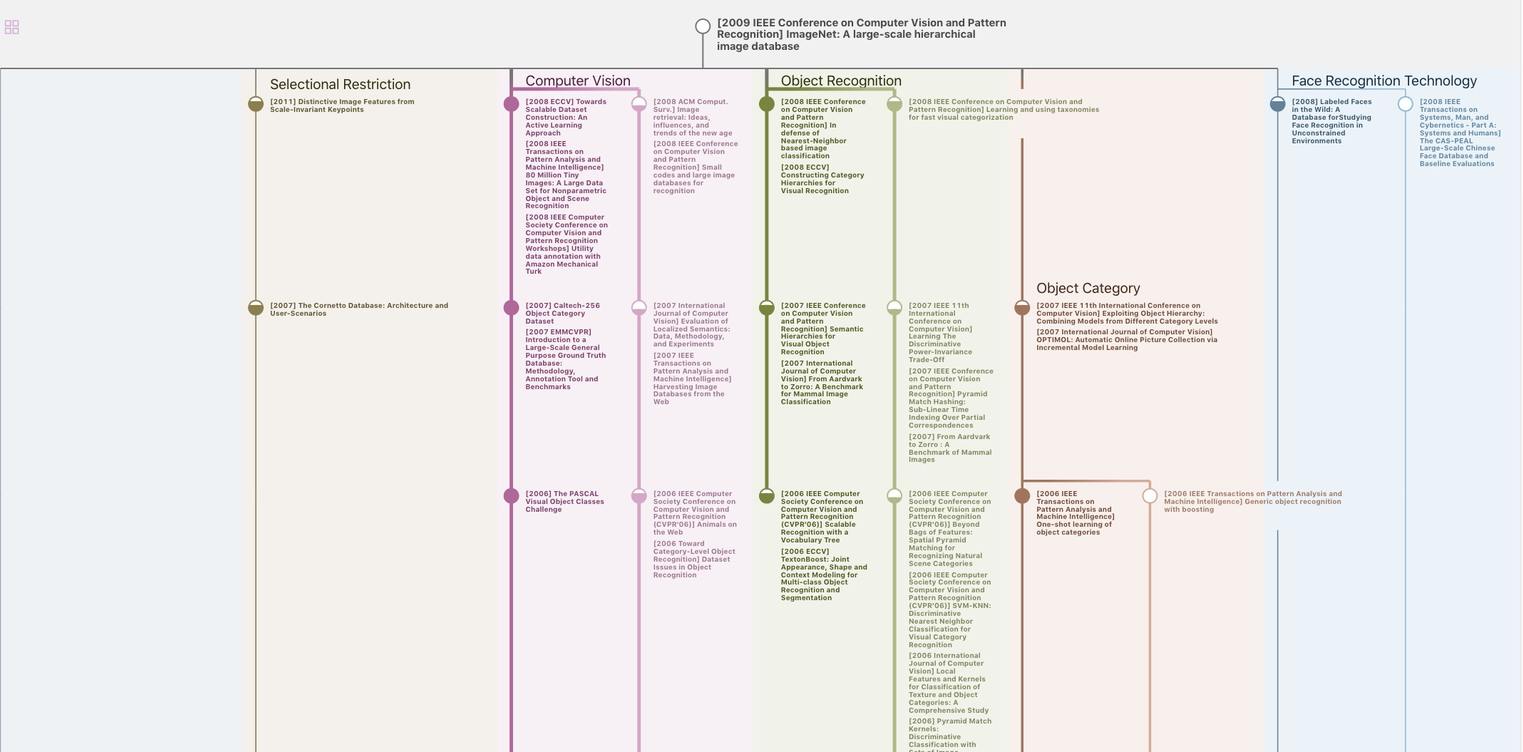
生成溯源树,研究论文发展脉络
Chat Paper
正在生成论文摘要