MRM-BERT: a novel deep neural network predictor of multiple RNA modifications by fusing BERT representation and sequence features
RNA BIOLOGY(2024)
摘要
RNA modifications play crucial roles in various biological processes and diseases. Accurate prediction of RNA modification sites is essential for understanding their functions. In this study, we propose a hybrid approach that fuses a pre-trained sequence representation with various sequence features to predict multiple types of RNA modifications in one combined prediction framework. We developed MRM-BERT, a deep learning method that combined the pre-trained DNABERT deep sequence representation module and the convolutional neural network (CNN) exploiting four traditional sequence feature encodings to improve the prediction performance. MRM-BERT was evaluated on multiple datasets of 12 commonly occurring RNA modifications, including m6A, m5C, m1A and so on. The results demonstrate that our hybrid model outperforms other models in terms of area under receiver operating characteristic curve (AUC) for all 12 types of RNA modifications. MRM-BERT is available as an online tool (http://117.122.208.21:8501) or source code (https://github.com/abhhba999/MRM-BERT), which allows users to predict RNA modification sites and visualize the results. Overall, our study provides an effective and efficient approach to predict multiple RNA modifications, contributing to the understanding of RNA biology and the development of therapeutic strategies.
更多查看译文
关键词
RNA modifications,deep learning,sequence features,Bidirectional encoder representations from transformers (BERT),convolutional neural network,web server
AI 理解论文
溯源树
样例
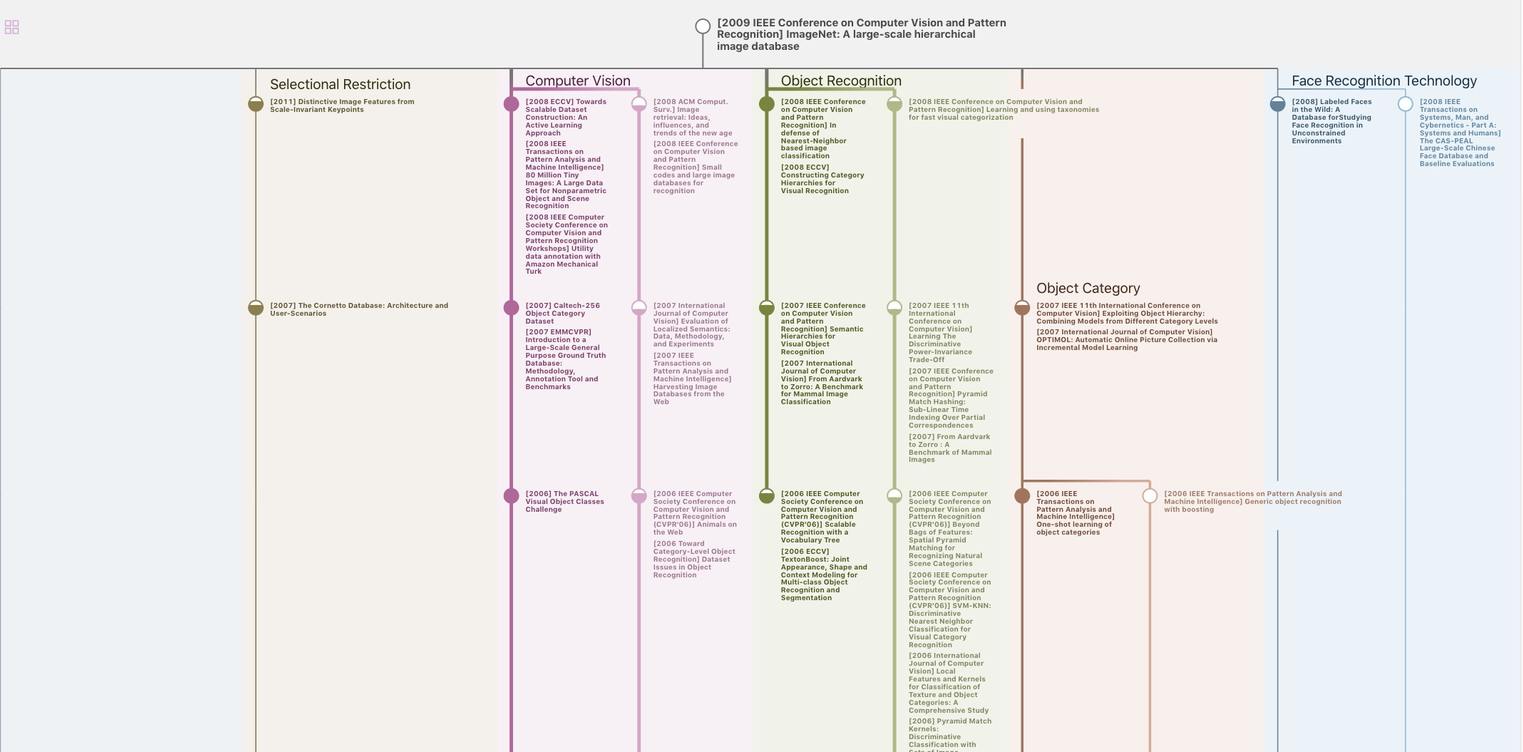
生成溯源树,研究论文发展脉络
Chat Paper
正在生成论文摘要