Information-Minimizing Generative Adversarial Network for Fair Generation and Classification
Neural Processing Letters(2024)
摘要
Studies show that machine learning models trained from biased data can discriminate against groups with certain sensitive attributes. This problem can be mitigated by cleaning the original data or learning fair representations. However, collecting real data in real-life is extremely time and resource-consuming, whereas generative models (e.g., GANs) can create new data that enable more application scenarios. Therefore, utilizing fair data generated by generative models can benefit various downstream tasks. In this paper, we propose a information-minimizing generative adversarial network to improve the fairness of machine learning by generating fair data. An ANOVA-based latent factor is constructed in the input for reducing the accuracy loss, and the joint adversarial training between the generator and classifier can better solve the indirect discrimination and achieve fair classification. Extensive experiments on various environments show the effectiveness of the proposed method.
更多查看译文
关键词
Generative adversarial network,Adversarial training,Indirect discrimination,Fair representation,Fair classification
AI 理解论文
溯源树
样例
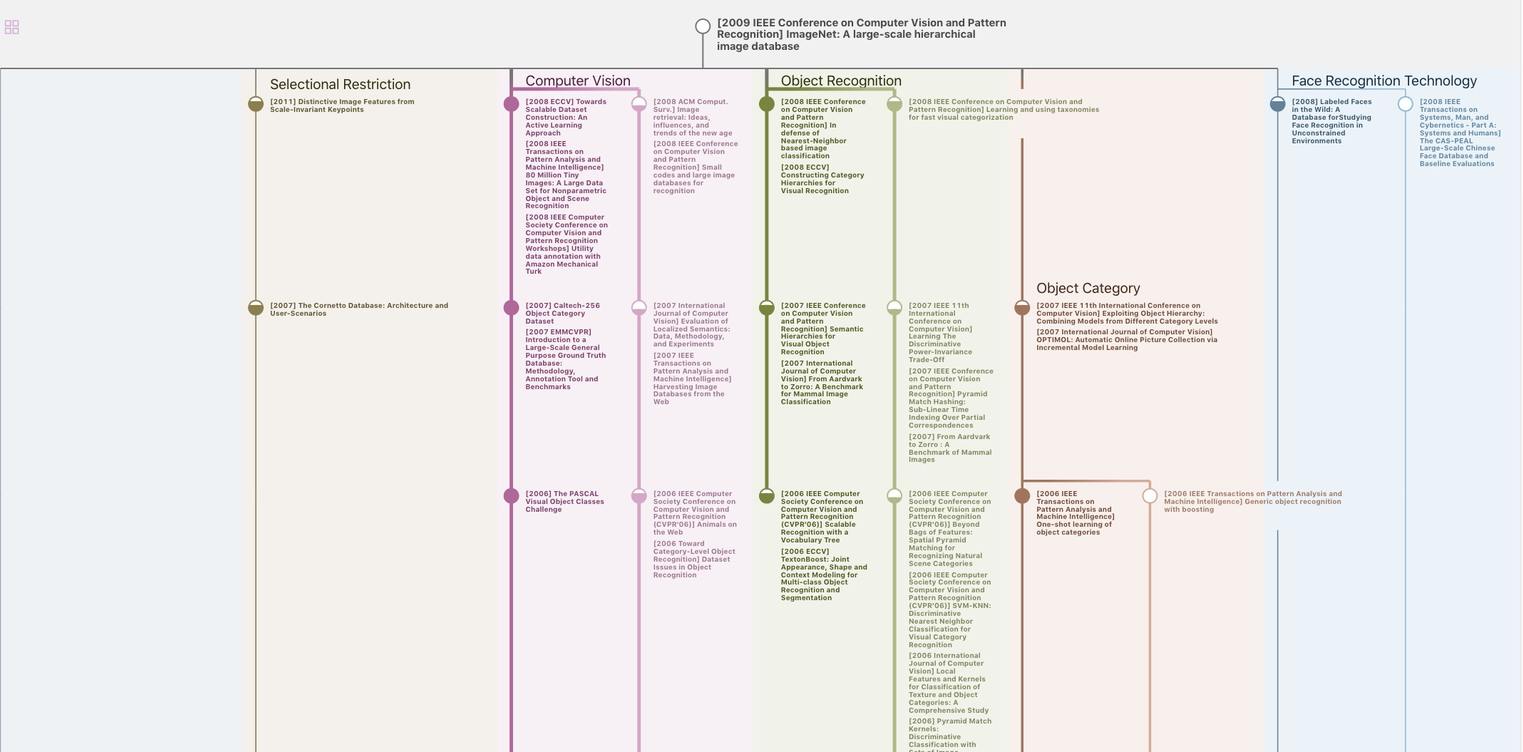
生成溯源树,研究论文发展脉络
Chat Paper
正在生成论文摘要