Unbiased characterization of atrial fibrillation phenotypic architecture provides insight to genetic liability and clinically relevant outcomes.
medRxiv : the preprint server for health sciences(2024)
Abstract
Background:Atrial Fibrillation (AF) is a common and clinically heterogeneous arrythmia. Machine learning (ML) algorithms can define data-driven disease subtypes in an unbiased fashion, but whether the AF subgroups defined in this way align with underlying mechanisms, such as high polygenic liability to AF or inflammation, and associate with clinical outcomes is unclear.
Methods:We identified individuals with AF in a large biobank linked to electronic health records (EHR) and genome-wide genotyping. The phenotypic architecture in the AF cohort was defined using principal component analysis of 35 expertly curated and uncorrelated clinical features. We applied an unsupervised co-clustering machine learning algorithm to the 35 features to identify distinct phenotypic AF clusters. The clinical inflammatory status of the clusters was defined using measured biomarkers (CRP, ESR, WBC, Neutrophil %, Platelet count, RDW) within 6 months of first AF mention in the EHR. Polygenic risk scores (PRS) for AF and cytokine levels were used to assess genetic liability of clusters to AF and inflammation, respectively. Clinical outcomes were collected from EHR up to the last medical contact.
Results:The analysis included 23,271 subjects with AF, of which 6,023 had available genome-wide genotyping. The machine learning algorithm identified 3 phenotypic clusters that were distinguished by increasing prevalence of comorbidities, particularly renal dysfunction, and coronary artery disease. Polygenic liability to AF across clusters was highest in the low comorbidity cluster. Clinically measured inflammatory biomarkers were highest in the high comorbid cluster, while there was no difference between groups in genetically predicted levels of inflammatory biomarkers. Subgroup assignment was associated with multiple clinical outcomes including mortality, stroke, bleeding, and use of cardiac implantable electronic devices after AF diagnosis.
Conclusion:Patient subgroups identified by unsupervised clustering were distinguished by comorbidity burden and associated with risk of clinically important outcomes. Polygenic liability to AF across clusters was greatest in the low comorbidity subgroup. Clinical inflammation, as reflected by measured biomarkers, was lowest in the subgroup with lowest comorbidities. However, there were no differences in genetically predicted levels of inflammatory biomarkers, suggesting associations between AF and inflammation is driven by acquired comorbidities rather than genetic predisposition.
MoreTranslated text
AI Read Science
Must-Reading Tree
Example
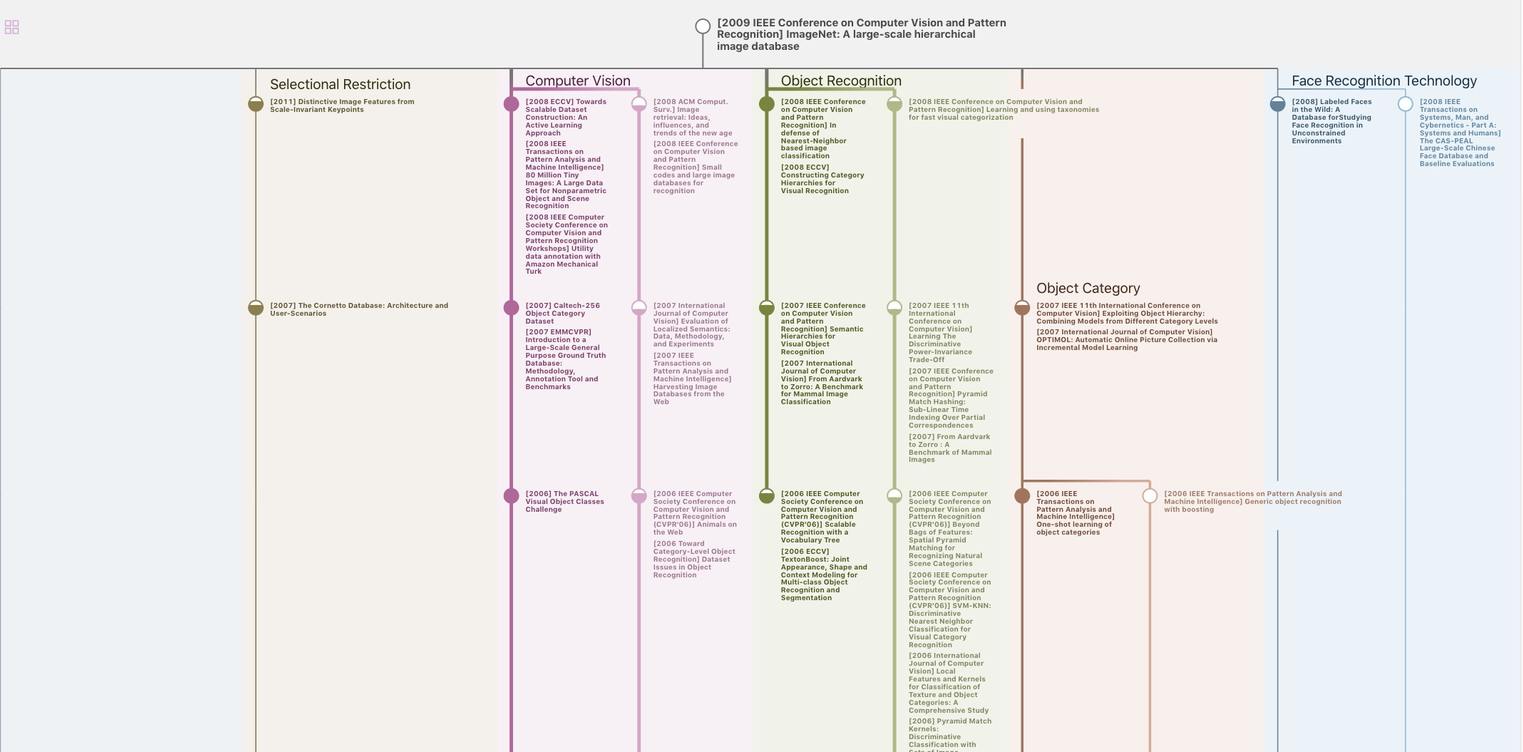
Generate MRT to find the research sequence of this paper
Chat Paper
Summary is being generated by the instructions you defined