Game Theory-Based Simultaneous Prediction and Planning for Autonomous Vehicle Navigation in Crowded Environments.
2023 IEEE 26th International Conference on Intelligent Transportation Systems (ITSC)(2023)
摘要
Navigating crowded environments with substantial pedestrian interactions poses distinctive challenges for autonomous vehicles (AVs), primarily due to the interdependence of prediction and planning modules and the necessity for vehicles and pedestrians to cooperate to achieve mutual benefits. In this work, we introduce a novel game theory-based autonomous vehicle stack designed to address these challenges while ensuring safe and efficient navigation in such complex settings. Our primary contributions encompass the development of an efficient Nash equilibrium approximation, facilitating simultaneous prediction and planning outputs, and effectively resolving the “chicken and egg” problem inherent in traditional autonomous software stacks. Moreover, we formulate a payoff function for the game that encapsulates the interactions among agents in the environment and demonstrate that our proposed stack improves classic probabilistic predictor. Through evaluations conducted using real-world datasets, we show that the proposed stack not only enhances prediction accuracy but also improves the planning success rate while mitigating integration errors. Further results can be accessed at the following link: https://youtu.be/ctGg4-ifCYQ.
更多查看译文
关键词
Autonomous Vehicles,Crowded Environment,Simultaneous Prediction,Autonomous Vehicle Navigation,Real-world Datasets,Prediction Probability,Nash Equilibrium,Environmental Agents,Payoff Function,Chicken-and-egg,Integral Error,Safe Navigation,Efficient Approximation,Efficient Navigation,Collision,Probabilistic Model,Best Response,Path Planning,Improve Prediction Accuracy,Safe Distance,Pedestrian Trajectory,Autonomous Navigation,Future Trajectories,Planning Algorithm,Strategy Space,Navigation In Environments,Graph Attention Network,Game-theoretic Model,Information Bottleneck,Imperfect Information
AI 理解论文
溯源树
样例
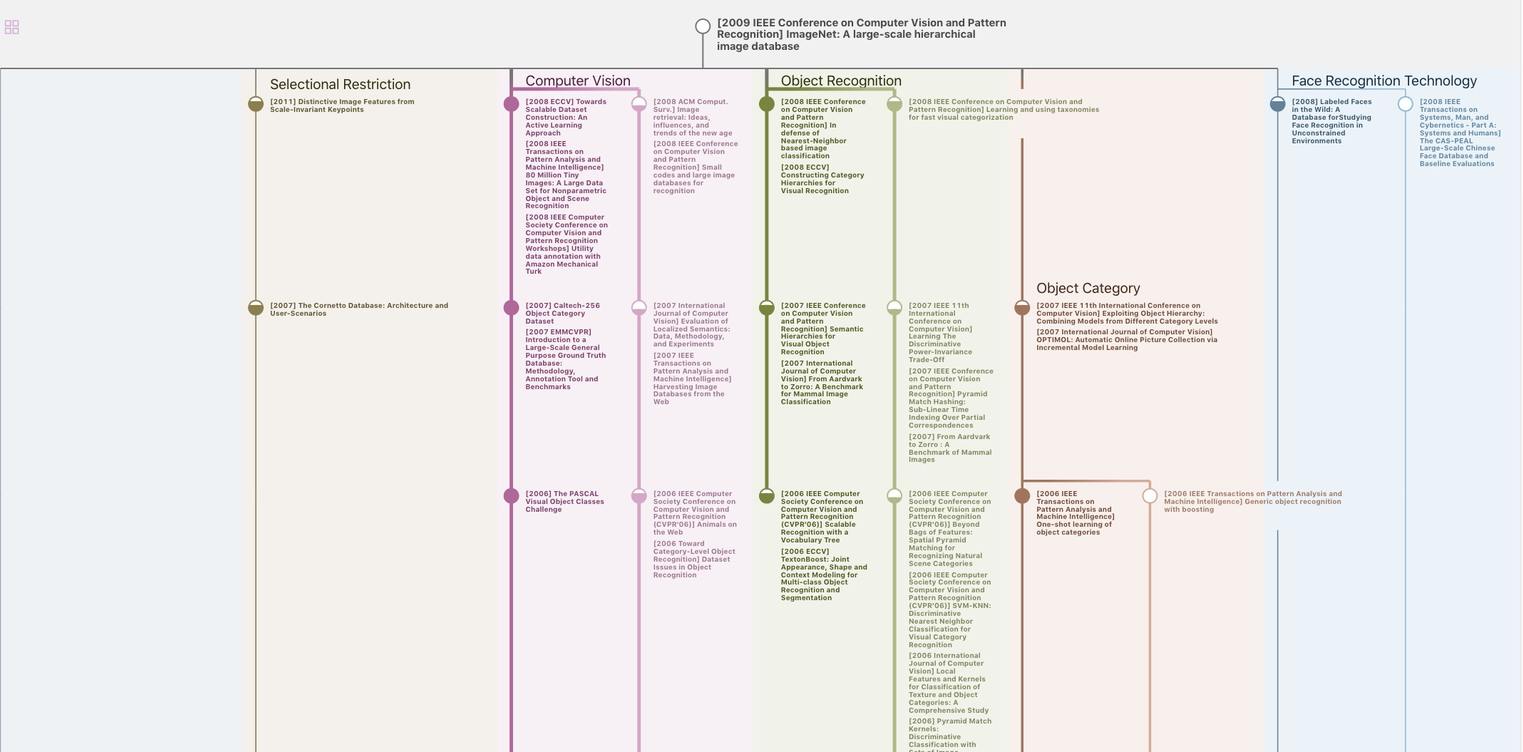
生成溯源树,研究论文发展脉络
Chat Paper
正在生成论文摘要