Demand-based Target Selection and Resource Trade for Parked-Vehicle Assisted Computing.
2023 IEEE 26th International Conference on Intelligent Transportation Systems (ITSC)(2023)
摘要
To meet the ever-increasing computing demands of smart cities, intelligent vehicles with rapid growth can be an effective supplement to the computing power network by sharing their underutilized computing resources. Considering that most of the vehicles are in the parked state, we call the computing paradigm as Parked-Vehicle Assisted Computing (PAC). However, current PAC systems ignore the parking demands of vehicles, which should consider not only the income brought by the computing resource trade but also the time cost and energy consumption of vehicles during traveling to the parking lot. In this paper, we design a trade framework and two trade models in a PAC system to encourage vehicles to rent out idle computing resources to enhance the computing power network. The costs of time and energy of vehicles during traveling and the computing energy consumption of both vehicles and Edge Servers (ESs) are considered. Based on the models, we design two optimization problems: 1) the individual trade value maximization problem to obtain personalized trade information of a vehicle, including the target parking lot, and 2) the trade allocation problem for determining trade results by maximizing the combined trade value, i.e. the maximum sum of the individual trade value of the requesting vehicles. We propose a low-complexity greedy-based Trade Allocation Rule (TAR) to obtain the trade allocation strategy. Simulation results show that the proposed algorithm outperforms other benchmark schemes in allocation time and combined trade value.
更多查看译文
关键词
Computing resource trade,parked-vehicle assisted computing,value maximization
AI 理解论文
溯源树
样例
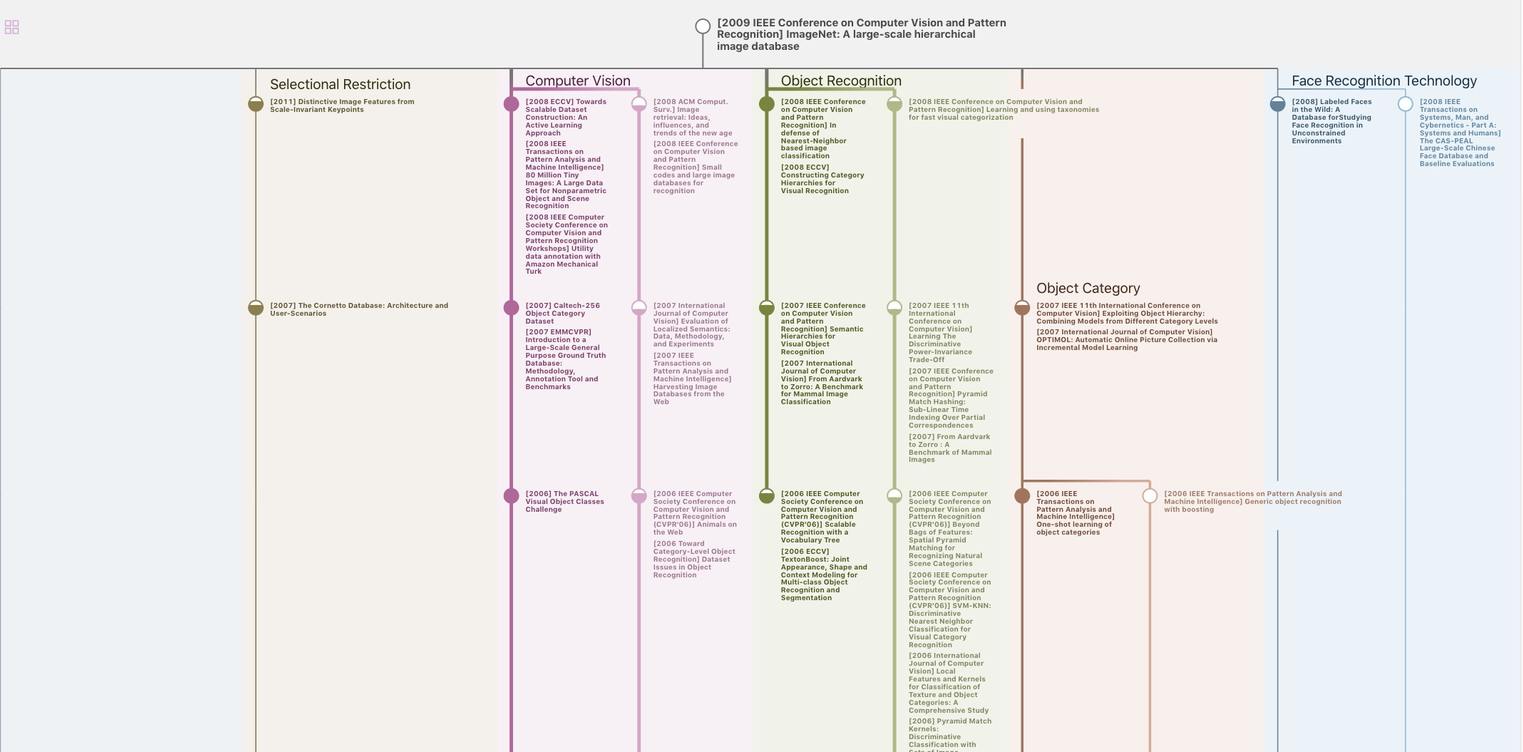
生成溯源树,研究论文发展脉络
Chat Paper
正在生成论文摘要