Delamination detection in CFRP laminates using deep transfer learning with limited experimental data
JOURNAL OF MATERIALS RESEARCH AND TECHNOLOGY-JMR&T(2024)
摘要
Carbon fiber reinforced polymer (CFRP) composites have been continuously replacing conventional metallic materials due to their excellent material properties. The orthotropic nature of CFRP composites makes them vulnerable to various types of damage. Among these, delamination stands out as the most common and severe form of damage. Therefore, deep learning based structural health monitoring (SHM) which performs autonomous health monitoring from sensor data have gained wide attention for delamination detection of CFRP composites. However, limited training data often restricts the application of these models for autonomous health monitoring. Therefore, the present research proposes convolutional neural network (CNN)-based pre-trained transfer learning method using ResNetV2 (RNV2) model to solve the data scarcity problem. The use of RNV2 model eliminated the need for developing the model from scratch and only required fine-tuning on the target composites dataset. The target dataset contained multi-class wavelet-transformed vibrational data obtained from CFRP specimens. The efficacy of the proposed approach is determined using various evaluation metrics on unseen dataset. The results of the validation demonstrated that the pre-trained RNV2 model can effectively perform SHM of CFRP composites even under limited data conditions.
更多查看译文
关键词
CFRP composites,Laminated composites,Delamination detection,Transfer learning,ResNet model,Deep learning
AI 理解论文
溯源树
样例
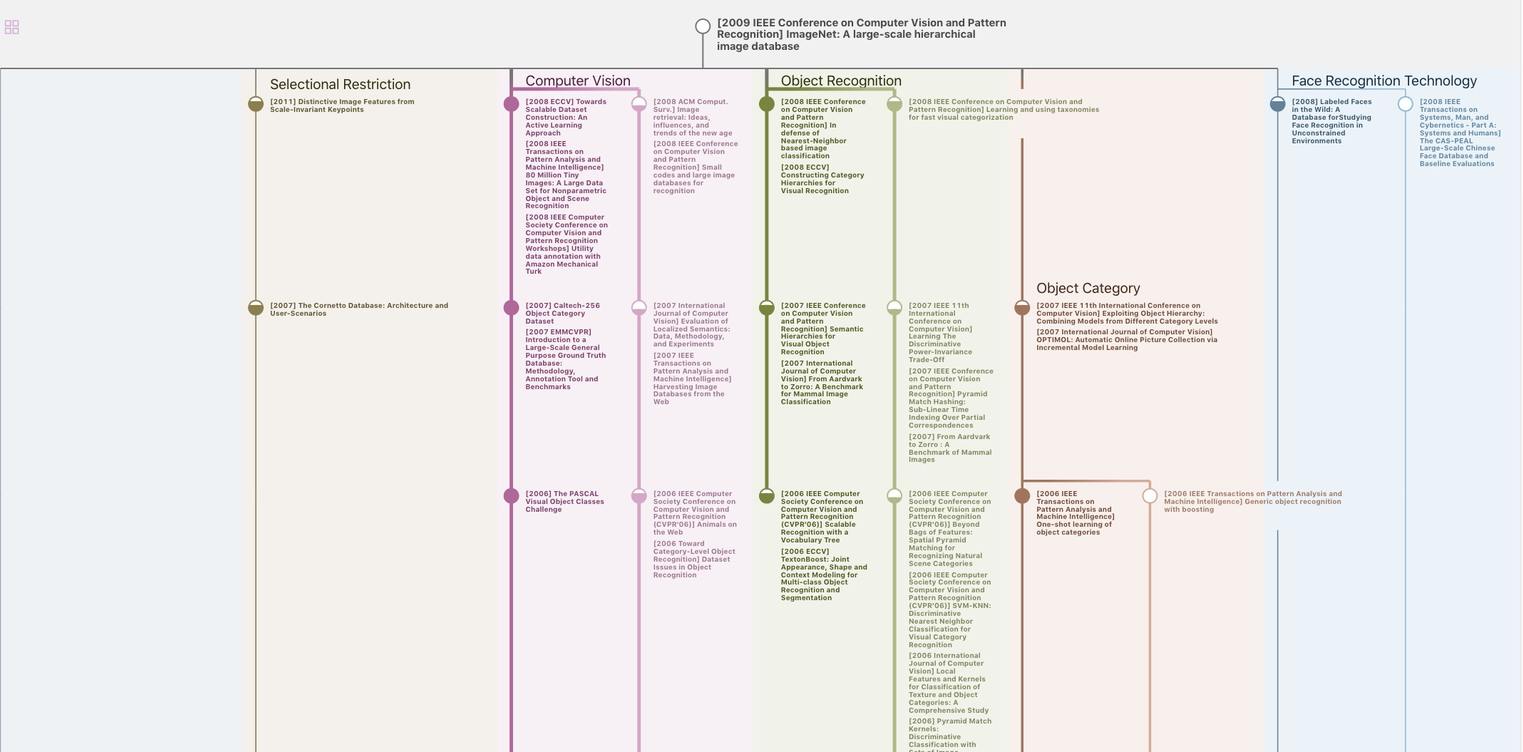
生成溯源树,研究论文发展脉络
Chat Paper
正在生成论文摘要