Prioritized Phase Split Optimization for Coordinated Traffic Signal Control in Urban Network Using Deep Reinforcement Learning.
2023 IEEE 26th International Conference on Intelligent Transportation Systems (ITSC)(2023)
Abstract
In traffic signal control (TSC), Deep Reinforcement Learning (DRL) has demonstrated its superiority to traditional approaches. However, there remain two challenges in DRL-based traffic signal control. How to cooperatively control decentralized intersection agents and how to apply TSC methods to the real world are still open. To address the aforementioned challenges, we propose a novel DRL-based strategy that optimizes the phase split for each intersection. We prioritize each intersection for signal control and adjust its phase split in accordance with its assigned priority. To be more specific, we start by optimizing the phase split of the intersection with the highest priority and then control the intersection with the following priority based on the outcome from the first intersection. In this manner, the coordinated optimization is taken into account. Additionally, we maintain the pre-defined order and definition of phases, and a fixed cycle time during the optimization process to support practical application. The proposed method yields an improvement in the network efficiency, ranging from 8–16 %, compared to the traditional method when tested in real urban network scenarios. This study also tests the impact of the control priority on the model performance.
MoreTranslated text
Key words
Light Signal,Deep Reinforcement Learning,Urban Network,Traffic Control,Traffic Signal Control,Split Phase,Network Efficiency,Order Phase,Control Priorities,Definition Phase,Average Time,Time Step,Time Delay,Travel Time,Average Speed,Multilayer Perceptron,Traffic Congestion,Traffic Flow,Discrete Set,Cycle Length,Average Delay,Average Delay Time,Multi-agent Reinforcement Learning,Traffic Demand,Traffic Conditions,Priority Order,Priority Setting,Road Section,Reward Function,Queue Length
AI Read Science
Must-Reading Tree
Example
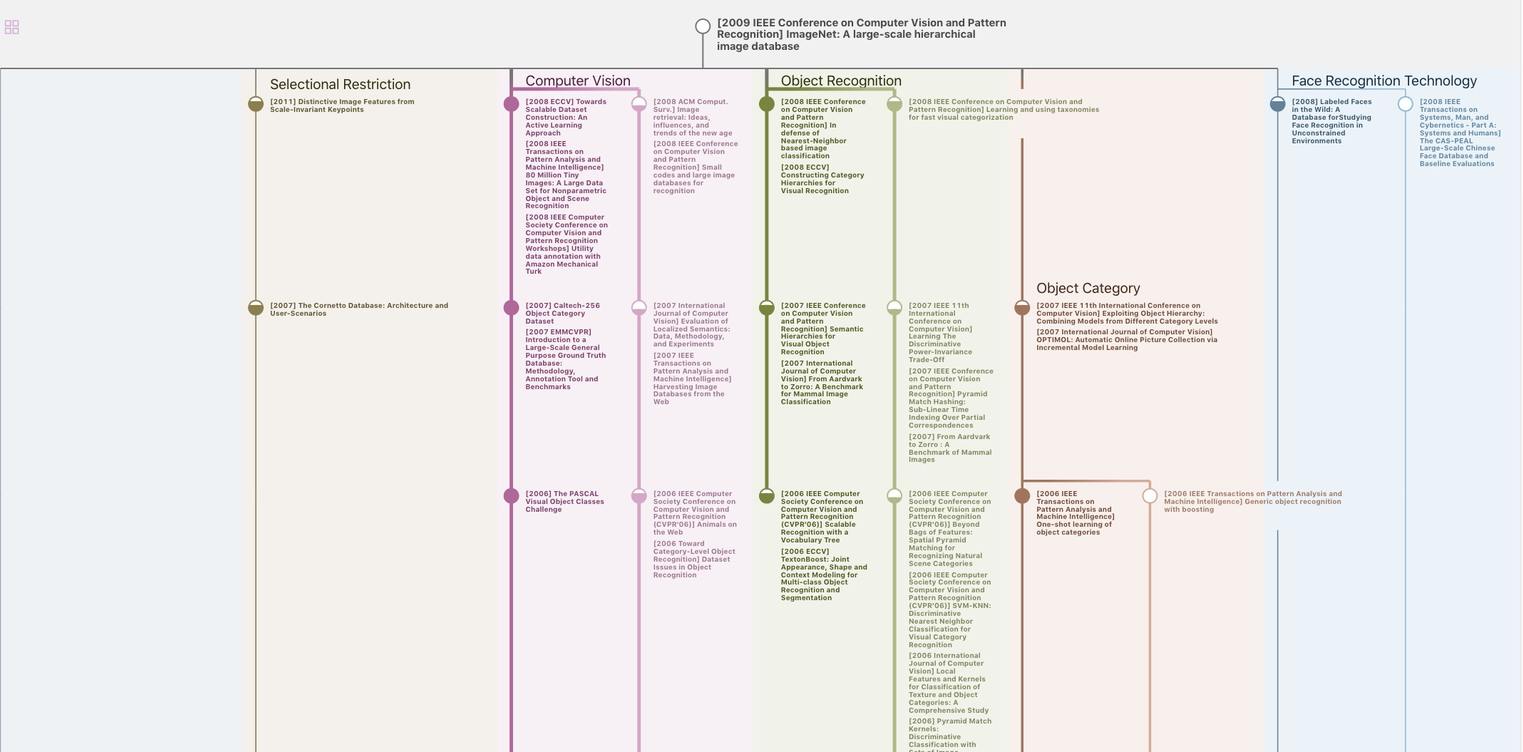
Generate MRT to find the research sequence of this paper
Chat Paper
Summary is being generated by the instructions you defined