YOLO-Substation: Inspection Target Detection in Complex Environment Based on Improved YOLOv7
2023 International Conference on Image Processing, Computer Vision and Machine Learning (ICICML)(2023)
摘要
Substations play a vital role in the field of electrical power production. In order to efficiently inspect substation equipment in complex environment using inspection robots, an target detection model based on YOLOv7, named YOLO-Substation, is proposed. To tackle the problem of feature degradation during training, a deformable convolution module is integrated into the backbone network, adapting to features of varying shapes and sizes. Furthermore, AAM is introduced to selectively emphasize crucial information from the input, improving the model’s performance in complex and dynamic backgrounds. The experiments demonstrate that the YOLO-Substation model can achieve accurate detection of inspection targets, with an mAP of 82.7%, an F1 score of 87.6%, and a recognition accuracy of 97.5%, surpassing other mainstream object detection models.
更多查看译文
关键词
component,Inspection target detection,Substation,YOLO-Substation,Deformable convolution,AAM
AI 理解论文
溯源树
样例
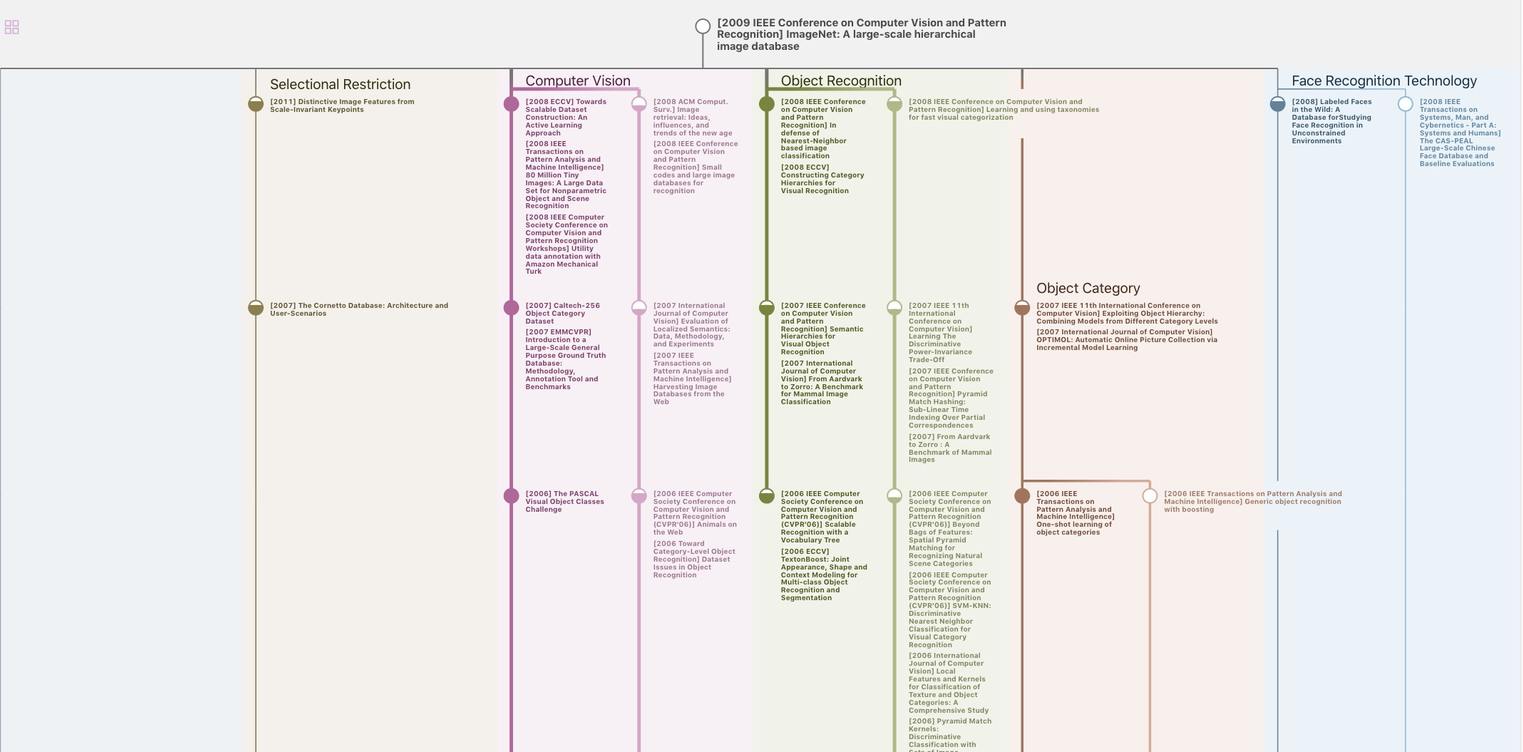
生成溯源树,研究论文发展脉络
Chat Paper
正在生成论文摘要