SolarNet- An efficient multi-model ensemble approach for Solar Power Forecasting based on Artificial Intelligence
2023 6th International Conference on Electrical Information and Communication Technology (EICT)(2023)
摘要
Solar energy has become a critical topic in the field of electrical power generation, and it is expected to remain so soon due to the world’s finite supply of fossil fuels and experts’ predictions of an energy crisis in the coming decades. Solar energy is gaining traction as a viable alternative, but solar electricity generation is heavily dependent on weather conditions, which poses certain challenges. Extensive use of solar energy is required to provide a reliable supply of electricity, and precise prediction of solar power is one solution to this problem. Accurately predicting solar power generation is critical for energy grid management and renewable energy planning. In this study, we introduce a new solar power forecasting approach called SolarNet, which utilizes deep learning techniques. The SolarNet model combines a multi-model ensemble approach that incorporates a 1D hybrid Convolutional Neural Network (Dilated Convolution), an attention-based Bidirectional LSTM, and a machine learning approach. We evaluated the performance of the SolarNet model using real-world solar power generation data and compared it to traditional forecasting methods in terms of Mean Absolute Error (MAE), Coefficient of Determination (R
2
), Root Mean Squared Error (RMSE), and Mean Absolute Percentage Error (MAPE). The results of our forecasts have been examined using several models, including feature engineering-based machine learning models, LSTM, BiLSTM, and gated recurrent units (GRU). The results demonstrate that SolarNet outperforms traditional methods in both accuracy and reliability. Our study contributes to the advancement of efficient and dependable solar power forecasting methods.
更多查看译文
关键词
ANN,BiLSTM,CNN,Hybrid Deep Learning Models,Photovoltaic Power Generation
AI 理解论文
溯源树
样例
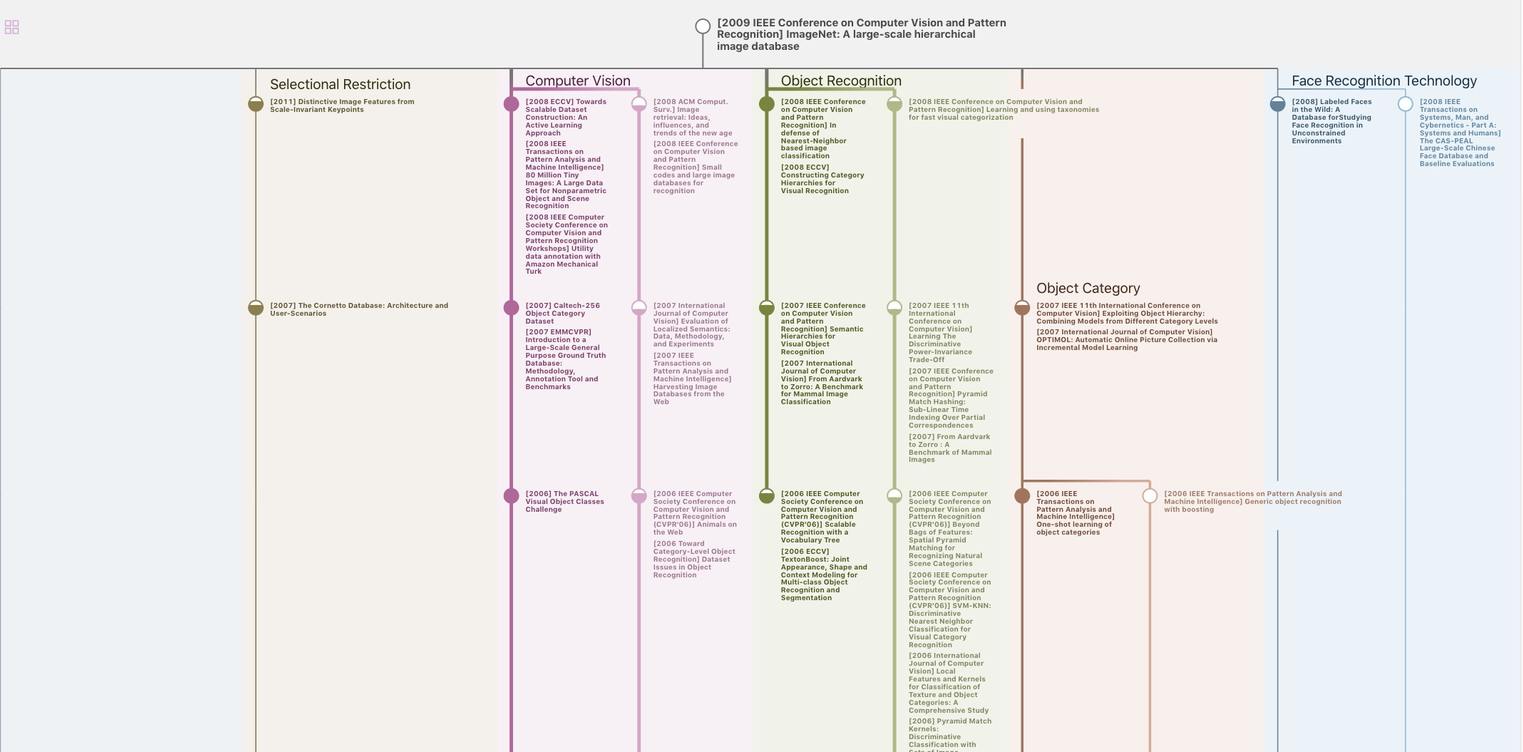
生成溯源树,研究论文发展脉络
Chat Paper
正在生成论文摘要