Comparative Study of Machine Learning Algorithm on Linguistic Distinctions over Text Related to Human Trafficking and Sexual Exploitation
2023 International Conference on Advanced Mechatronics, Intelligent Manufacture and Industrial Automation (ICAMIMIA)(2023)
摘要
Human trafficking and sexual exploitation (HTSE) are grave global issues that demand comprehensive understanding and effective countermeasures. In total, this issue—also known as modern slavery—affected an estimated 49.6 million people in 2021 and earns traffickers at least $150 billion annually, making it one of the world’s most profitable crimes. This crime can penetrate our daily life through internet advertisement, trying to trap non-tech savvy people. Automated analysis for such adverts is essential. It can be used for identifying linguistic patterns in human trafficking and sexual exploitation-related adverstisements. This research employs various machine learning algorithms (i.e., Naïve Bayes, Random Forest, Decision Trees) to classify benign and HTSE advertisements. From our experiments, Random Forest performed best, achieving a high F1 score (0.962), balancing Precision and Recall effectively. Naïve Bayes also showed promising results, while Gradient Boosting had variable F1 scores, and the Tree algorithm scored the lowest. This analysis provides insights into algorithm capabilities and limitations in addressing linguistic distinctions related to human trafficking and sexual exploitation, contributing to better detection systems. By applying these algorithms to a diverse dataset, we aim to enhance our understanding of linguistic cues in addressing these societal challenges and consider potential solutions, policy implications, and future research.
更多查看译文
关键词
Human Trafficking,Sexual Exploitation,Text Analysis,Linguistic Distinctions,Naïve Bayes,Random Forest,Trees
AI 理解论文
溯源树
样例
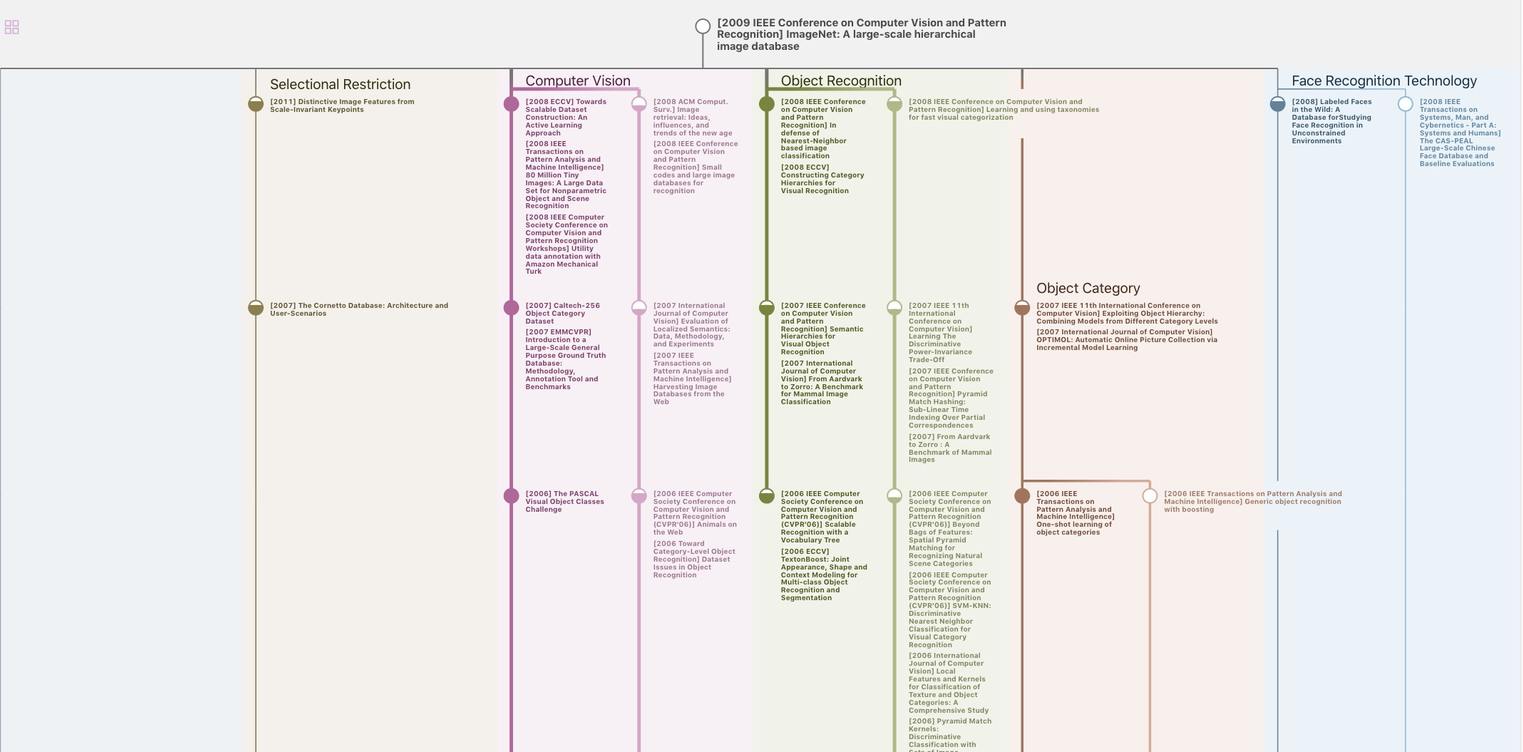
生成溯源树,研究论文发展脉络
Chat Paper
正在生成论文摘要