Weatherproofing Retrieval for Localization with Generative AI and Geometric Consistency
CoRR(2024)
摘要
State-of-the-art visual localization approaches generally rely on a first
image retrieval step whose role is crucial. Yet, retrieval often struggles when
facing varying conditions, due to e.g. weather or time of day, with dramatic
consequences on the visual localization accuracy. In this paper, we improve
this retrieval step and tailor it to the final localization task. Among the
several changes we advocate for, we propose to synthesize variants of the
training set images, obtained from generative text-to-image models, in order to
automatically expand the training set towards a number of nameable variations
that particularly hurt visual localization. After expanding the training set,
we propose a training approach that leverages the specificities and the
underlying geometry of this mix of real and synthetic images. We experimentally
show that those changes translate into large improvements for the most
challenging visual localization datasets. Project page:
https://europe.naverlabs.com/ret4loc
更多查看译文
关键词
visual localization,image retrieval,synthetic data,domain shift,geometric consistency,long-term visual localization,ret4loc,image alteration
AI 理解论文
溯源树
样例
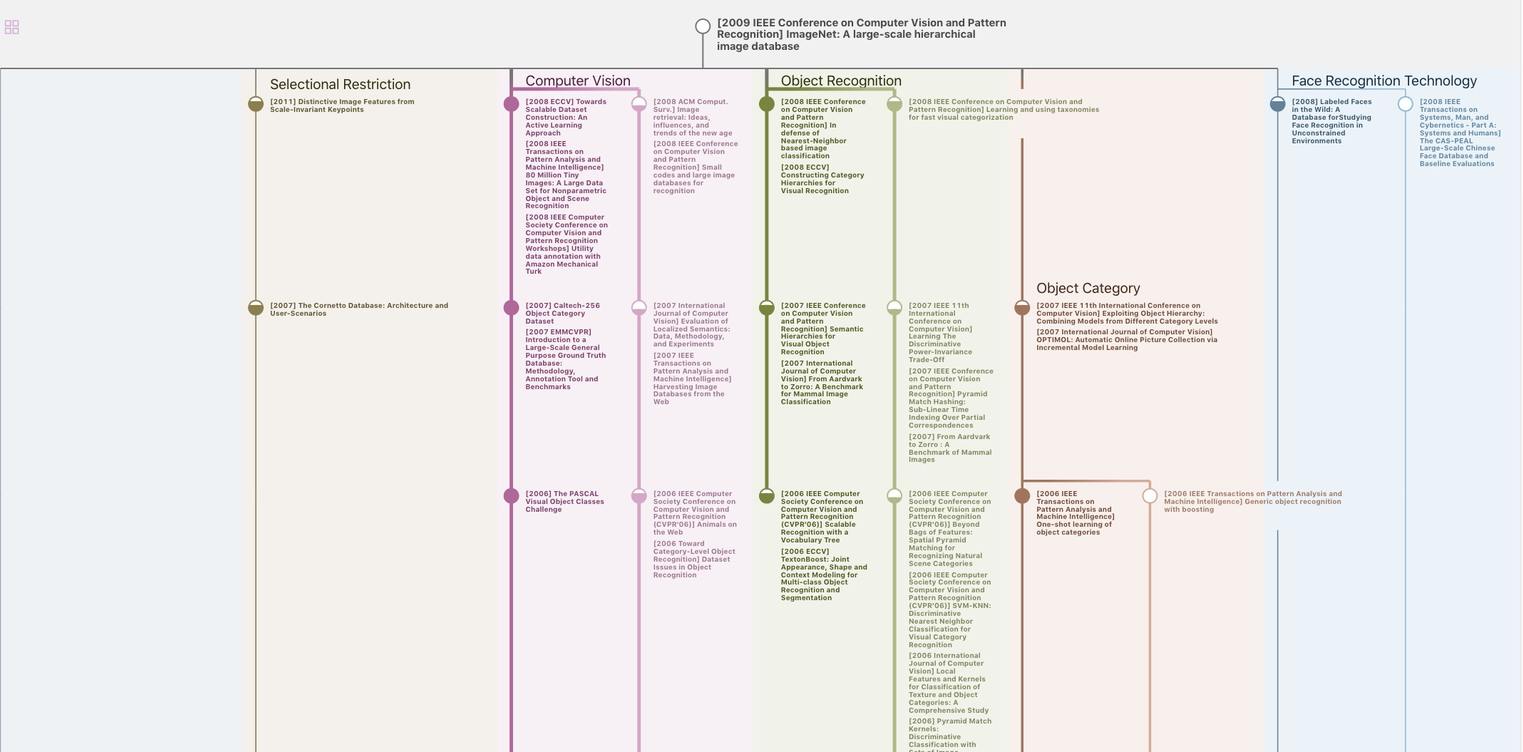
生成溯源树,研究论文发展脉络
Chat Paper
正在生成论文摘要