Nearly Optimal Regret for Decentralized Online Convex Optimization
CoRR(2024)
摘要
We investigate decentralized online convex optimization (D-OCO), in which a
set of local learners are required to minimize a sequence of global loss
functions using only local computations and communications. Previous studies
have established O(n^5/4ρ^-1/2√(T)) and O(n^3/2ρ^-1log
T) regret bounds for convex and strongly convex functions respectively, where
n is the number of local learners, ρ<1 is the spectral gap of the
communication matrix, and T is the time horizon. However, there exist large
gaps from the existing lower bounds, i.e., Ω(n√(T)) for convex
functions and Ω(n) for strongly convex functions. To fill these gaps, in
this paper, we first develop novel D-OCO algorithms that can respectively
reduce the regret bounds for convex and strongly convex functions to
Õ(nρ^-1/4√(T)) and Õ(nρ^-1/2log T). The
primary technique is to design an online accelerated gossip strategy that
enjoys a faster average consensus among local learners. Furthermore, by
carefully exploiting the spectral properties of a specific network topology, we
enhance the lower bounds for convex and strongly convex functions to
Ω(nρ^-1/4√(T)) and Ω(nρ^-1/2), respectively. These
lower bounds suggest that our algorithms are nearly optimal in terms of T,
n, and ρ.
更多查看译文
AI 理解论文
溯源树
样例
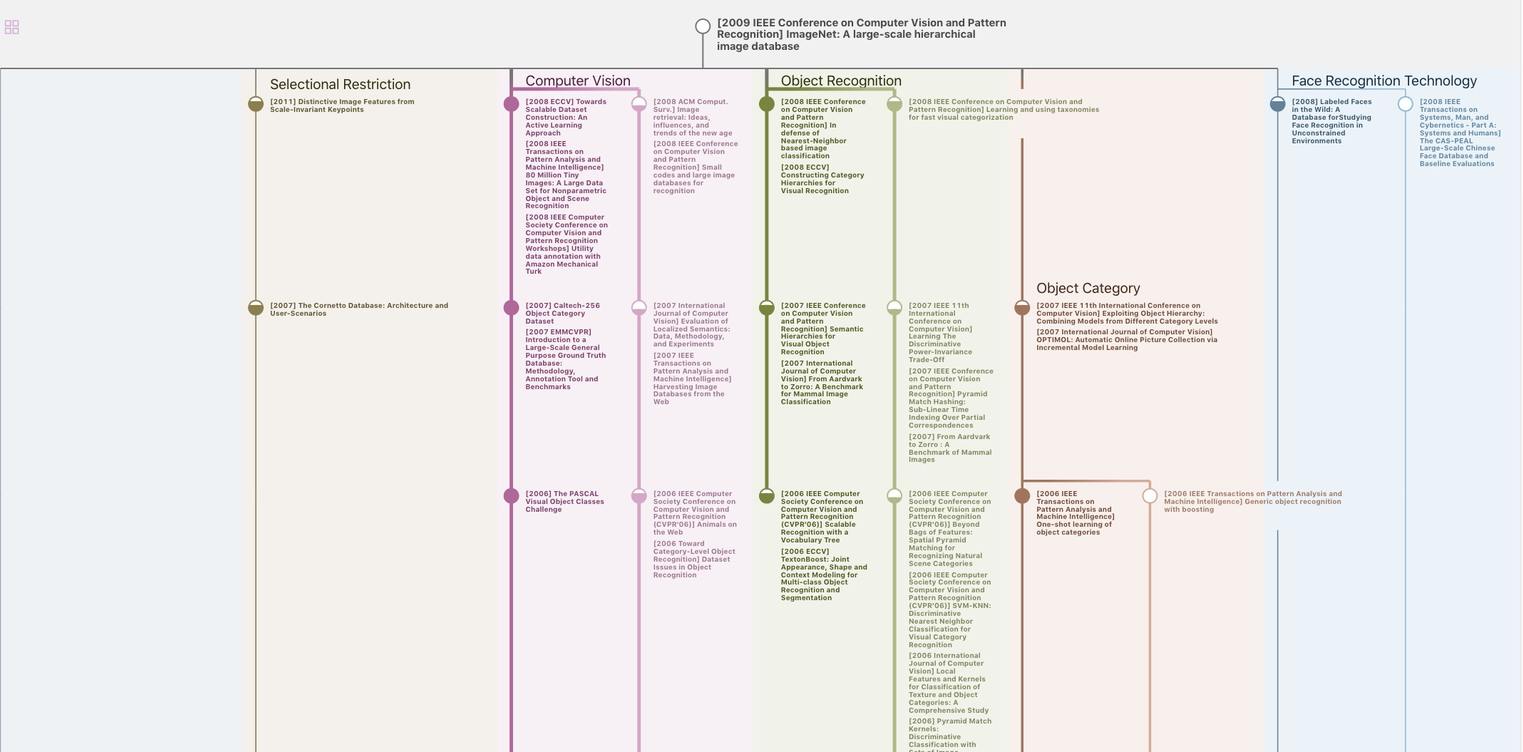
生成溯源树,研究论文发展脉络
Chat Paper
正在生成论文摘要