Exploring Federated Deep Learning for Standardising Naming Conventions in Radiotherapy Data
CoRR(2024)
摘要
Standardising structure volume names in radiotherapy (RT) data is necessary
to enable data mining and analyses, especially across multi-institutional
centres. This process is time and resource intensive, which highlights the need
for new automated and efficient approaches to handle the task. Several machine
learning-based methods have been proposed and evaluated to standardise
nomenclature. However, no studies have considered that RT patient records are
distributed across multiple data centres. This paper introduces a method that
emulates real-world environments to establish standardised nomenclature. This
is achieved by integrating decentralised real-time data and federated learning
(FL). A multimodal deep artificial neural network was proposed to standardise
RT data in federated settings. Three types of possible attributes were
extracted from the structures to train the deep learning models: tabular,
visual, and volumetric. Simulated experiments were carried out to train the
models across several scenarios including multiple data centres, input
modalities, and aggregation strategies. The models were compared against models
developed with single modalities in federated settings, in addition to models
trained in centralised settings. Categorical classification accuracy was
calculated on hold-out samples to inform the models performance. Our results
highlight the need for fusing multiple modalities when training such models,
with better performance reported with tabular-volumetric models. In addition,
we report comparable accuracy compared to models built in centralised settings.
This demonstrates the suitability of FL for handling the standardization task.
Additional ablation analyses showed that the total number of samples in the
data centres and the number of data centres highly affects the training process
and should be carefully considered when building standardisation models.
更多查看译文
AI 理解论文
溯源树
样例
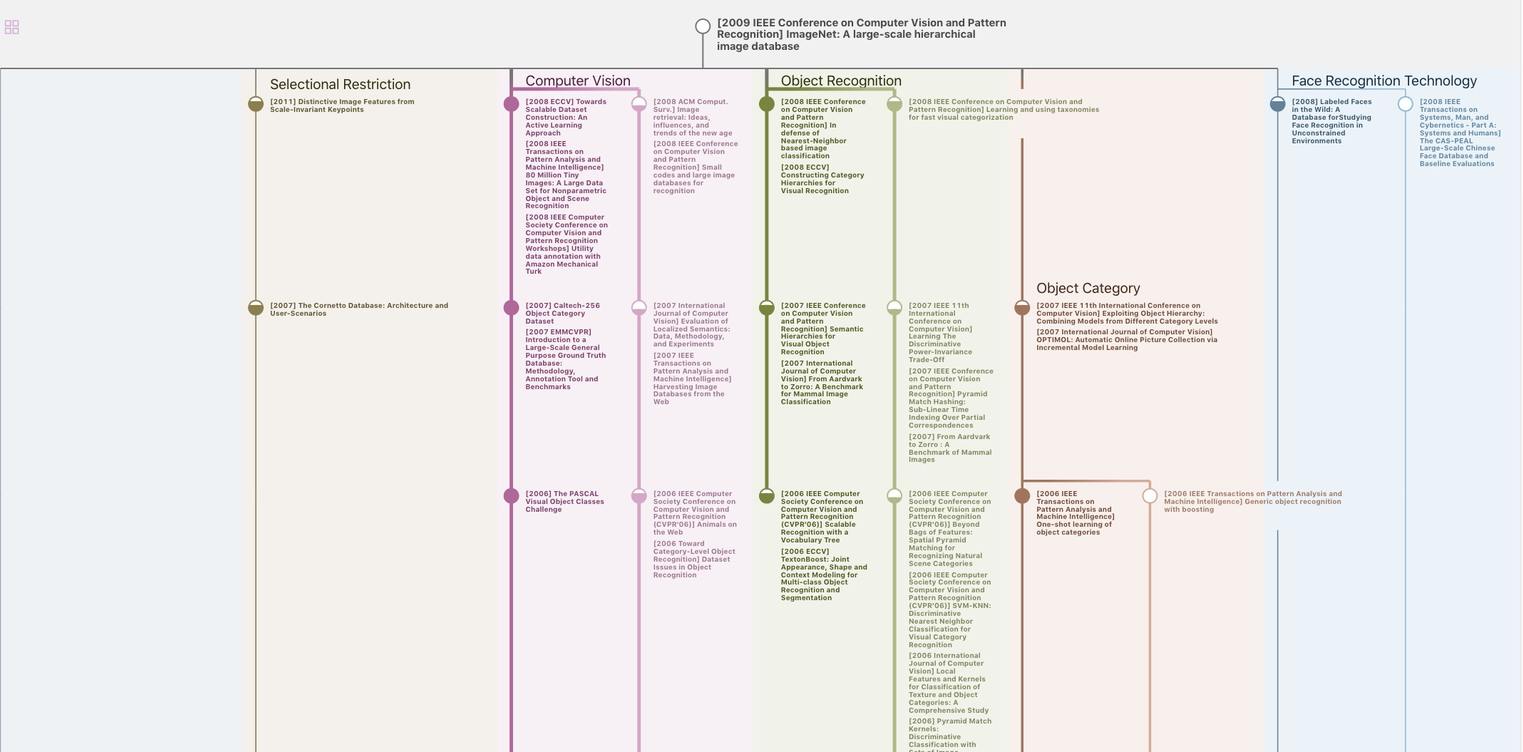
生成溯源树,研究论文发展脉络
Chat Paper
正在生成论文摘要