Predicting the Emergence of Solar Active Regions Using Machine Learning
CoRR(2024)
摘要
To create early warning capabilities for upcoming Space Weather disturbances,
we have selected a dataset of 61 emerging active regions, which allows us to
identify characteristic features in the evolution of acoustic power density to
predict continuum intensity emergence. For our study, we have utilized Doppler
shift and continuum intensity observations from the Helioseismic and Magnetic
Imager (HMI) onboard the Solar Dynamics Observatory (SDO). The local tracking
of 30.66 x 30.66-degree patches in the vicinity of active regions allowed us to
trace the evolution of active regions starting from the pre-emergence state. We
have developed a machine learning model to capture the acoustic power flux
density variations associated with upcoming magnetic flux emergence. The
trained Long Short-Term Memory (LSTM) model is able to predict 5 hours ahead
whether, in a given area of the solar surface, continuum intensity values will
decrease. The performed study allows us to investigate the potential of the
machine learning approach to predict the emergence of active regions using
acoustic power maps as input.
更多查看译文
AI 理解论文
溯源树
样例
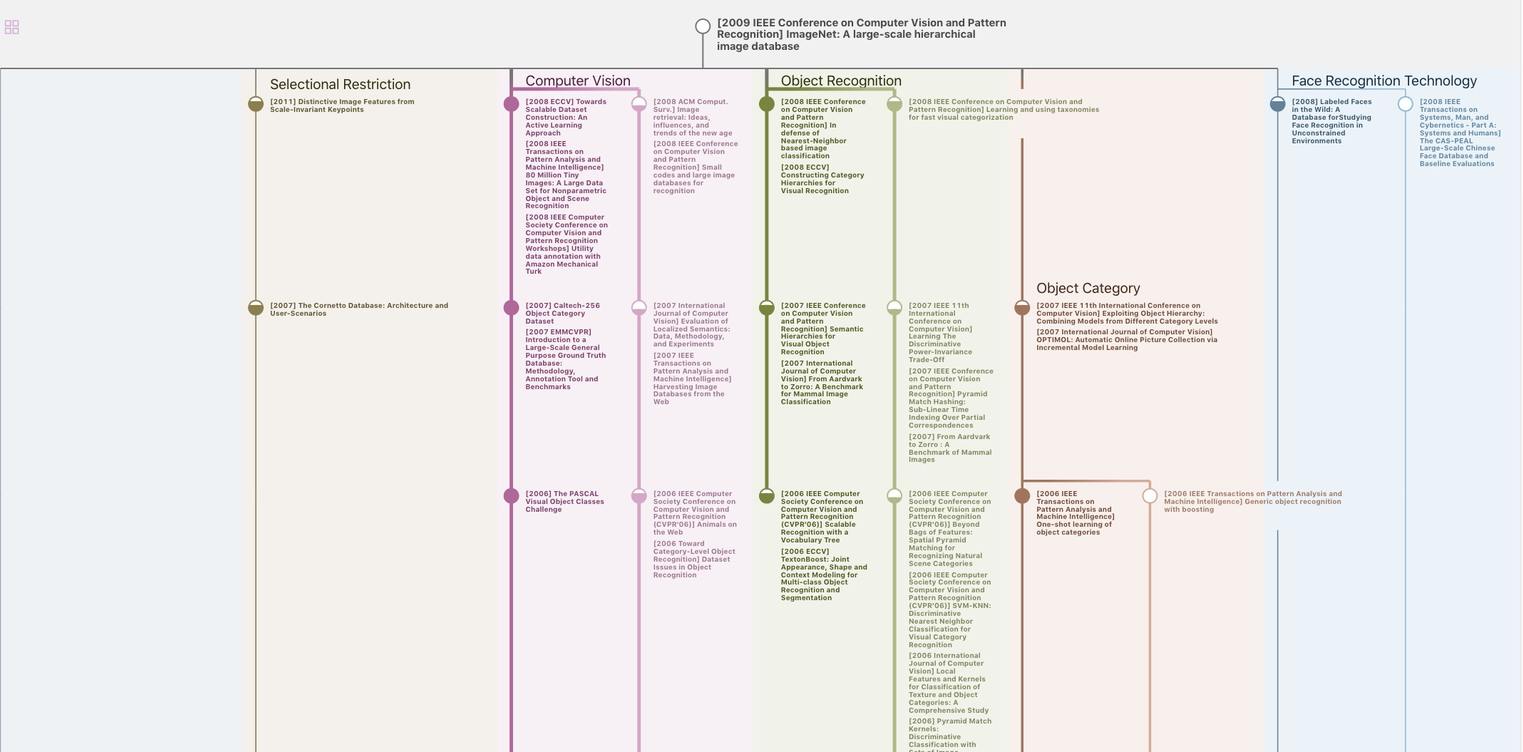
生成溯源树,研究论文发展脉络
Chat Paper
正在生成论文摘要