Lightweight Deep Learning Based Channel Estimation for Extremely Large-Scale Massive MIMO Systems
IEEE Transactions on Vehicular Technology(2024)
摘要
Extremely large-scale massive multiple-input multiple-output (XL-MIMO)
systems introduce the much higher channel dimensionality and incur the
additional near-field propagation effect, aggravating the computation load and
the difficulty to acquire the prior knowledge for channel estimation. In this
article, an XL-MIMO channel network (XLCNet) is developed to estimate the
high-dimensional channel, which is a universal solution for both the near-field
users and far-field users with different channel statistics. Furthermore, a
compressed XLCNet (C-XLCNet) is designed via weight pruning and quantization to
accelerate the model inference as well as to facilitate the model storage and
transmission. Simulation results show the performance superiority and
universality of XLCNet. Compared to XLCNet, C-XLCNet incurs the limited
performance loss while reducing the computational complexity and model size by
about 10 × and 36 ×, respectively.
更多查看译文
关键词
XL-MIMO,channel estimation,deep learning,weight pruning,weight quantization
AI 理解论文
溯源树
样例
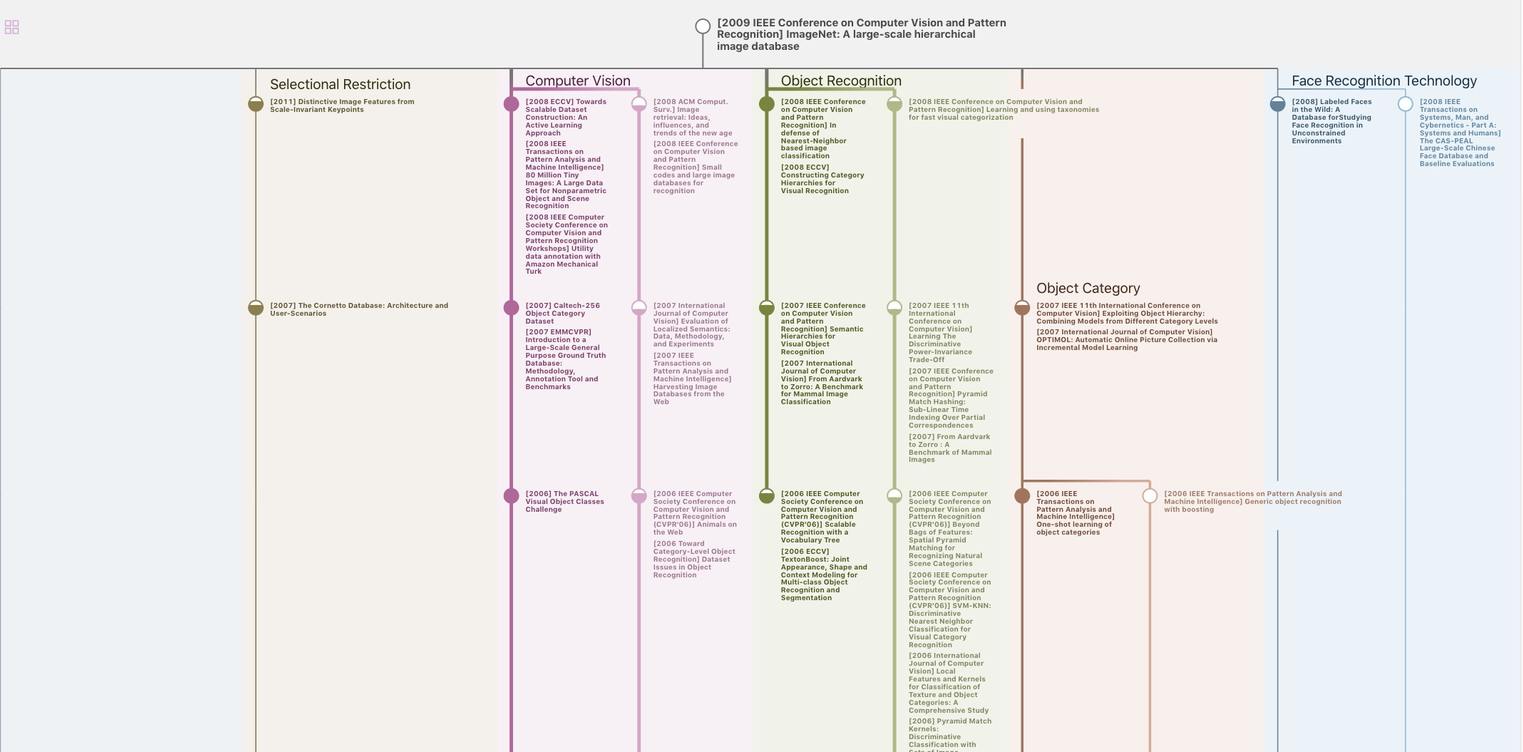
生成溯源树,研究论文发展脉络
Chat Paper
正在生成论文摘要