Safety Reinforced Model Predictive Control (SRMPC): Improving MPC with Reinforcement Learning for Motion Planning in Autonomous Driving.
2023 IEEE 26th International Conference on Intelligent Transportation Systems (ITSC)(2023)
摘要
Model predictive control (MPC) is widely used for motion planning, particularly in autonomous driving. Real-time capability of the planner requires utilizing convex approximation of optimal control problems (OCPs) for the planner. However, such approximations confine the solution to a subspace, which might not contain the global optimum. To address this, we propose using safe reinforcement learning (SRL) to obtain a new and safe reference trajectory within MPC. By employing a learning-based approach, the MPC can explore solutions beyond the close neighborhood of the previous one, potentially finding global optima. We incorporate constrained reinforcement learning (CRL) to ensure safety in automated driving, using a handcrafted energy function-based safety index as the constraint objective to model safe and unsafe regions. Our approach utilizes a state-dependent Lagrangian multiplier, learned concurrently with the safe policy, to solve the CRL problem. Through experimentation in a highway scenario, we demonstrate the superiority of our approach over both MPC and SRL in terms of safety and performance measures.
更多查看译文
关键词
Autonomous Vehicles,Path Planning,Model Predictive Control,Optimization Problem,Global Optimization,Lagrange Multiplier,Optimal Control Problem,Reference Trajectory,Automated Vehicles,Safety Index,Control Variables,Time Step,Objective Function,Value Function,Cost Function,Local Optimum,Quadratic Programming,Safe Conditions,Reward Function,Markov Decision Process,Longitudinal Velocity,Nonlinear Model Predictive Control,Proximal Policy Optimization,State-value Function,Slack Variables,Reinforcement Learning Algorithm,Forward Simulation,Safe Set,Multiplier Values,Entire Training
AI 理解论文
溯源树
样例
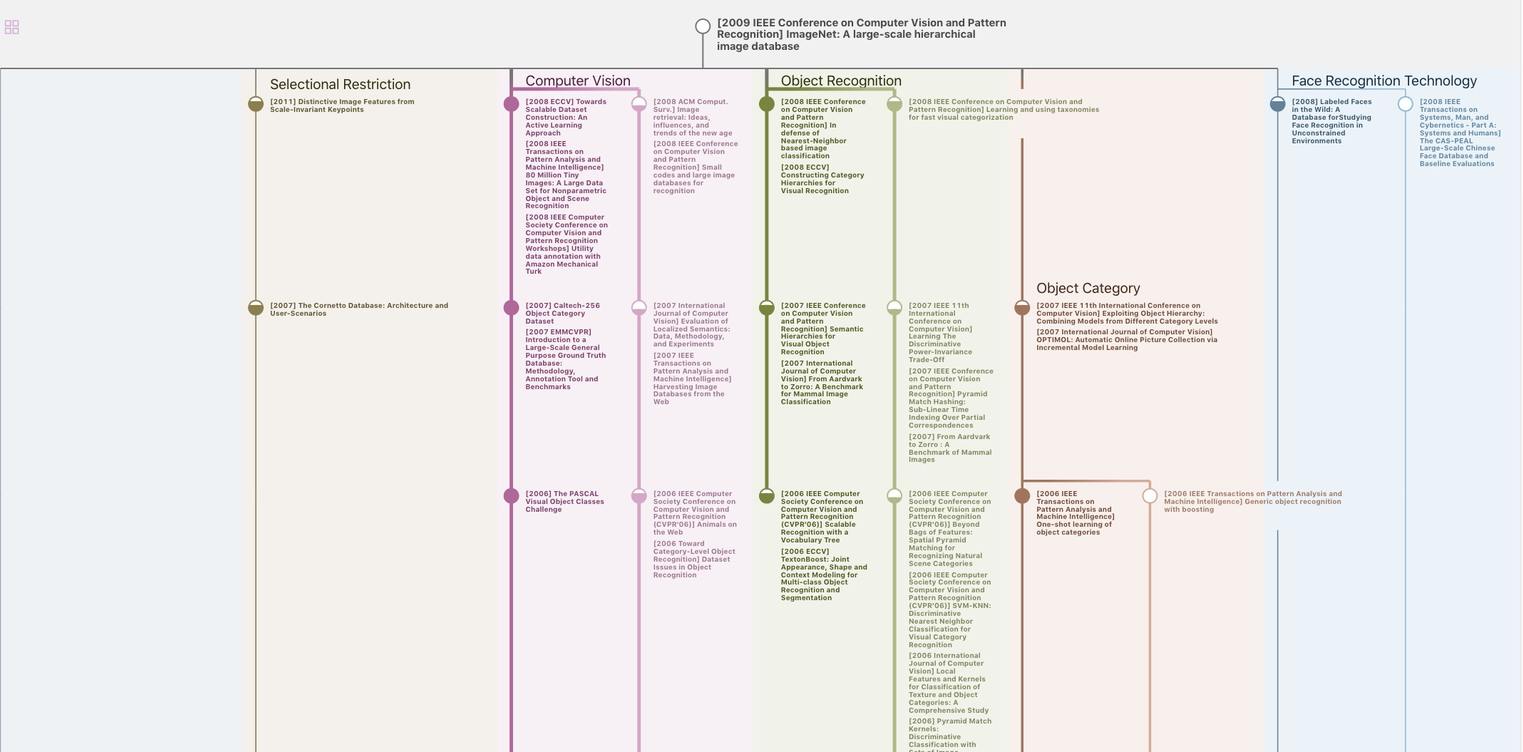
生成溯源树,研究论文发展脉络
Chat Paper
正在生成论文摘要