Deep Learning-Based, Multiclass Approach to Cancer Classification on Liquid Biopsy Data
IEEE JOURNAL OF TRANSLATIONAL ENGINEERING IN HEALTH AND MEDICINE(2024)
摘要
The field of cancer diagnostics has been revolutionized by liquid biopsies, which offer a bridge between laboratory research and clinical settings. These tests are less invasive than traditional biopsies and more convenient than routine imaging methods. Liquid biopsies allow studying of tumor-derived markers in bodily fluids, enabling the development of more precise cancer diagnostic tests for screening, disease monitoring, and therapy personalization. This study presents a multiclass approach based on deep learning to analyze and classify diseases based on blood platelet RNA. Its primary objective is to enhance cancer-type diagnosis in clinical settings by leveraging the power of deep learning combined with high-throughput sequencing of liquid biopsy. Ultimately, the study demonstrates the potential of this approach to accurately identify the patient's type of cancer. Methods: The developed method classifies patients using heatmap images, generated based on gene expression arranged according to the Kyoto Encyclopedia of Genes and Genomes pathways. The images represent samples of patients with ovarian cancer, endometrial cancer, glioblastoma, non-small cell lung cancer, and sarcoma, as well as cancer patients with brain metastasis. Results: Our deep learning-based models reached 66.51% balanced accuracy when distinguishing between those 6 sites of cancer origin and 90.5% balanced accuracy on a location-specific dataset where cancer types from close locations were grouped. The developed models were further investigated with an explainable artificial intelligence-based approach (XAI) - SHAP. They returned a set of 60 genes with the highest impact on the models' decision-making process. Conclusions: Our results show that deep-learning methods are a promising opportunity for cancer detection and could support clinicians' decision-making process in finding the solution for the black-box problem.
更多查看译文
关键词
Cancer,Medical diagnostic imaging,Liquids,Biopsy,Deep learning,RNA,Biological system modeling,liquid biopsies,tumor educated platelets (TEP),CNN-convolutional neural network,explainable AI
AI 理解论文
溯源树
样例
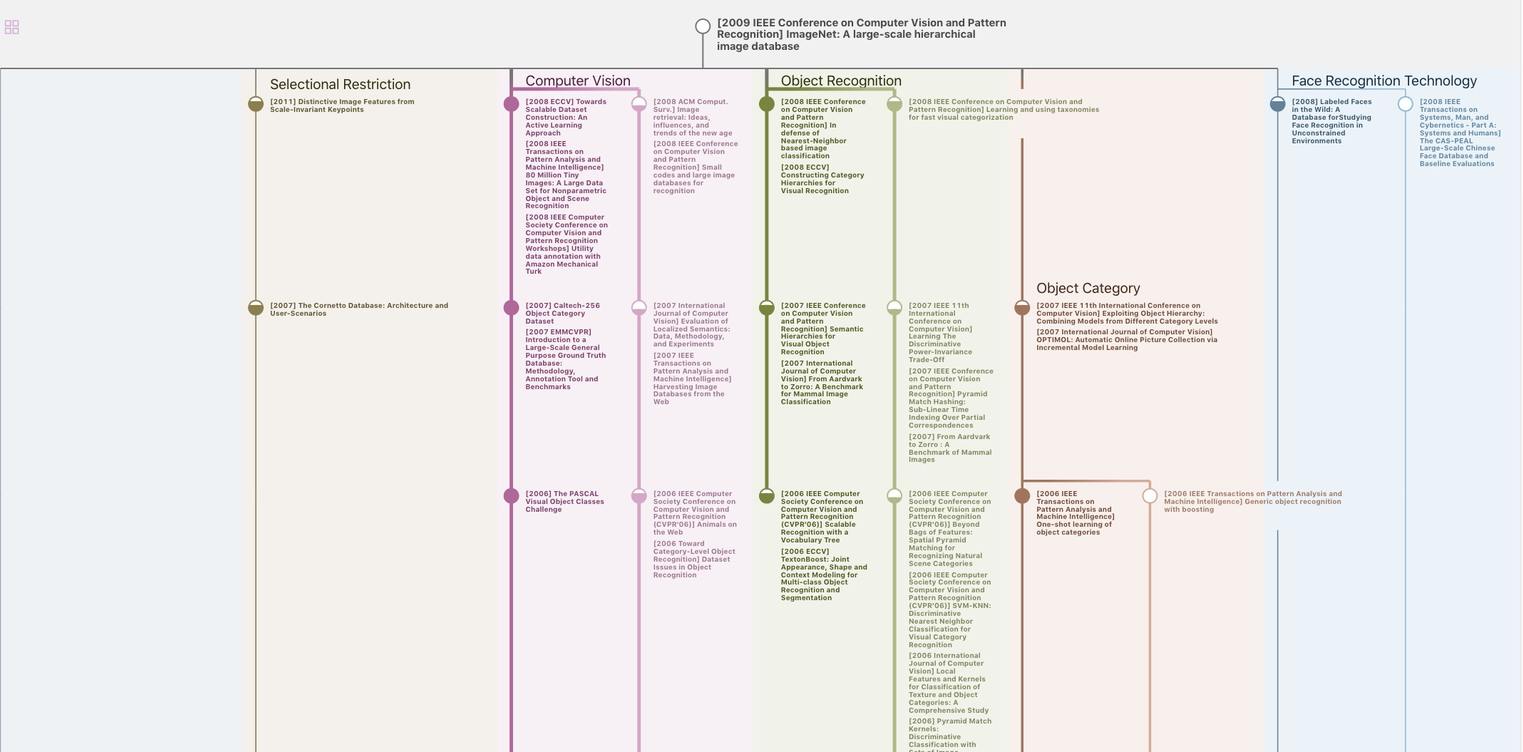
生成溯源树,研究论文发展脉络
Chat Paper
正在生成论文摘要