UDRSNet: An unsupervised deformable registration module based on image structure similarity
MEDICAL PHYSICS(2024)
摘要
BackgroundImage registration is a challenging problem in many clinical tasks, but deep learning has made significant progress in this area over the past few years. Real-time and robust registration has been made possible by supervised transformation estimation. However, the quality of registrations using this framework depends on the quality of ground truth labels such as displacement field.PurposeTo propose a simple and reliable method for registering medical images based on image structure similarity in a completely unsupervised manner.MethodsWe proposed a deep cascade unsupervised deformable registration approach to align images without reliable clinical data labels. Our basic network was composed of a displacement estimation module (ResUnet) and a deformation module (spatial transformer layers). We adopted l2-norm to regularize the deformation field instead of the traditional l1-norm regularization. Additionally, we utilized structural similarity (ssim) estimation during the training stage to enhance the structural consistency between the deformed images and the reference images.ResultsExperiments results indicated that by incorporating ssim loss, our cascaded methods not only achieved higher dice score of 0.9873, ssim score of 0.9559, normalized cross-correlation (NCC) score of 0.9950, and lower relative sum of squared difference (SSD) error of 0.0313 on CT images, but also outperformed the comparative methods on ultrasound dataset. The statistical t-test results also proved that these improvements of our method have statistical significance.ConclusionsIn this study, the promising results based on diverse evaluation metrics have demonstrated that our model is simple and effective in deformable image registration (DIR). The generalization ability of the model was also verified through experiments on liver CT images and cardiac ultrasound images.
更多查看译文
关键词
cascaded network,deformable registration,structural similarity,unsupervised learning
AI 理解论文
溯源树
样例
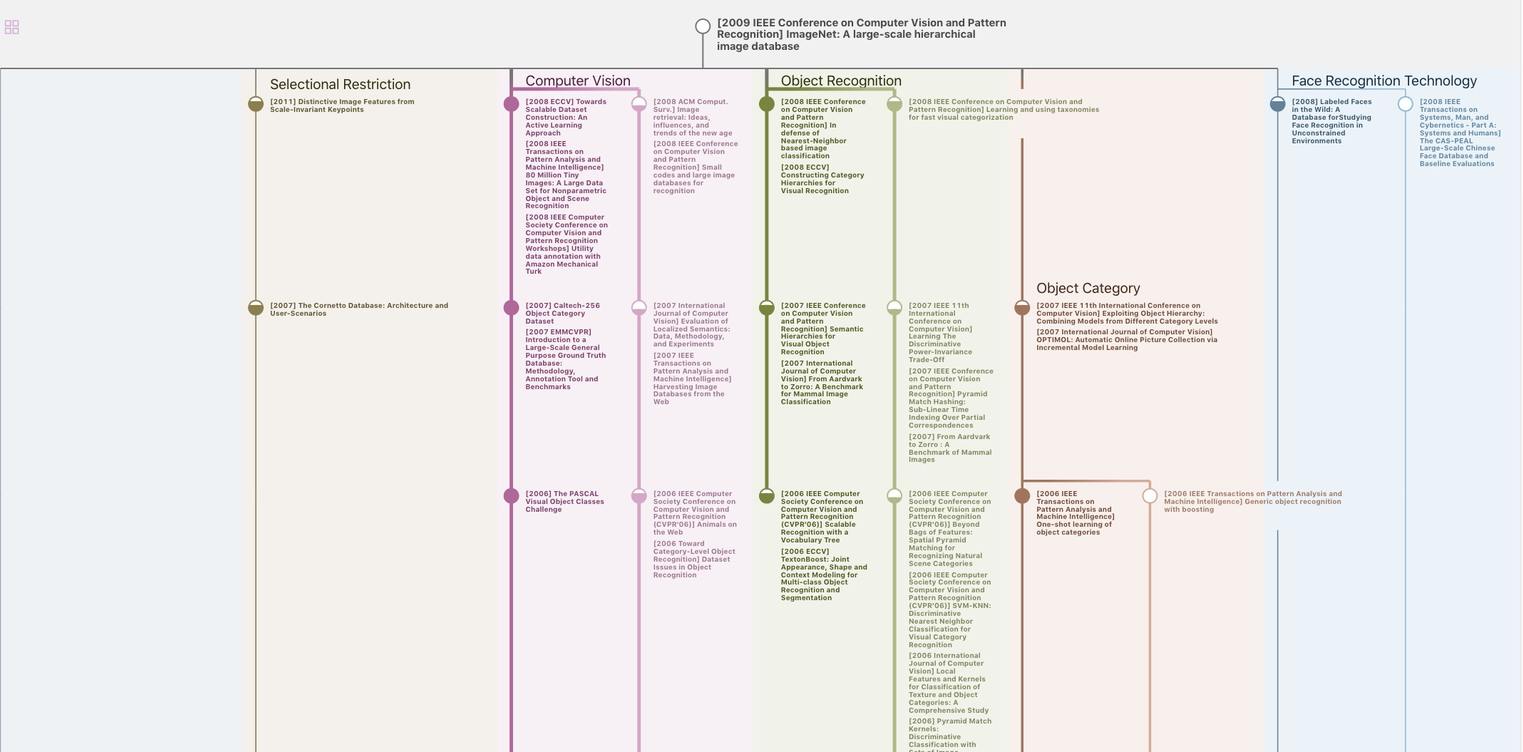
生成溯源树,研究论文发展脉络
Chat Paper
正在生成论文摘要