Interaction-Aware Maneuver Intention Prediction Using Gated Mixture-of-Experts Attention Mechanism.
2023 IEEE 26th International Conference on Intelligent Transportation Systems (ITSC)(2023)
摘要
This paper addresses the task of predicting the behavior of traffic participants, which involves complexities such as road geometry and agent interactions. To overcome these challenges, this paper presents a novel framework called AIMP (Attention-based Interaction-aware Maneuver Prediction). AIMP utilizes interaction graphs to extract intricate interaction features from traffic scenes. The framework incorporates a Gated Mixture-of-Experts Attention Mechanism, which combines information from road geometry, interaction patterns, and motion dynamics. This fusion process also considers prior maneuver intention estimations, enhancing both explainability and informativeness. Experimental results highlight a performance enhancement (approximately 2% ∼ 9% of accuracy) of the proposed AIMP framework compared to alternative fusion methods.
更多查看译文
关键词
Attention Mechanism,Predictor Of Intention,Maneuver Intention,Fusion Method,Prior Estimates,Interaction Graph,Road Geometry,Model Performance,Convolutional Neural Network,Long Short-term Memory,Recurrent Neural Network,Class Distribution,Autonomous Vehicles,Osteopontin,Feature Fusion,Motion Features,Graph Convolutional Network,Prediction Framework,Gated Recurrent Unit,Historical Trajectory,Graph Attention Network,Lane Change,Longitudinal Prediction,Fusion Operation,Target Vehicle,Occupancy Grid,Modal Features,Multilayer Perceptron,Data Augmentation,Decoder Block
AI 理解论文
溯源树
样例
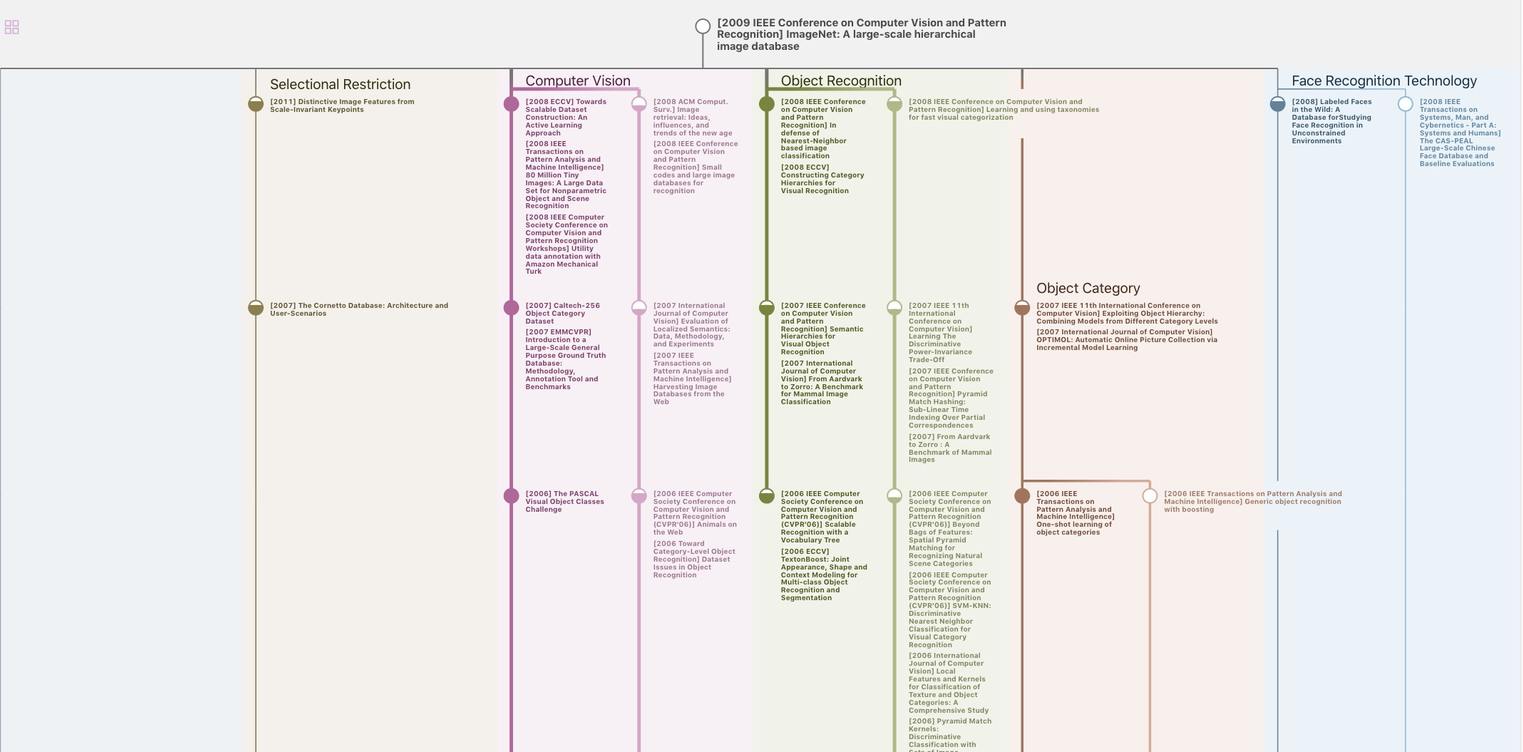
生成溯源树,研究论文发展脉络
Chat Paper
正在生成论文摘要