Robust Two-Stage Transport Data Imputation with Changepoint Detection and Tucker Decomposition.
2023 IEEE 26th International Conference on Intelligent Transportation Systems (ITSC)(2023)
摘要
Transport data is an essential resource for understanding traffic patterns and informing transport planning and policy. However, data-driven transportation research is often haunted by the prevalent issue of missing data, which can significantly impact the accuracy and reliability of data analysis. Despite extensive efforts in developing effective data imputation models, it is found that their results can deteriorate when faced with complex missing patterns and high non-stationarity of time series. To address these issues, we propose a two-stage imputation framework with changepoint detection and Tucker decomposition. Time series decomposition is embedded in the proposed framework to capture the temporal characteristics of transport time series. Experiment results demonstrate that our proposed method outperforms state-of-the-art methods in various challenging missing scenarios.
更多查看译文
关键词
transport data imputation,changepoint detection,tensor completion,Tucker decomposition
AI 理解论文
溯源树
样例
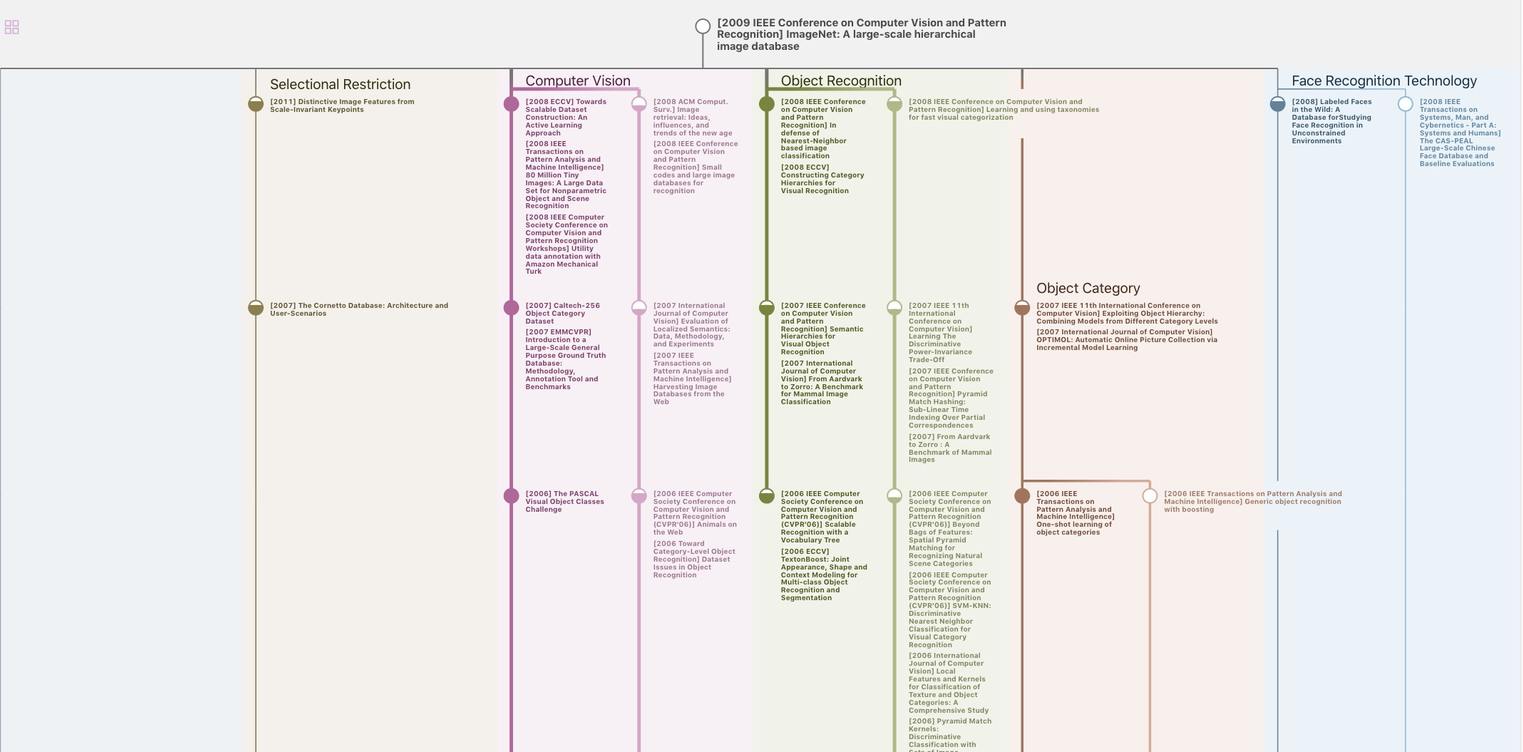
生成溯源树,研究论文发展脉络
Chat Paper
正在生成论文摘要