Multi-Masks Generation for Increasing Robustness of Dense Direct Methods.
2023 IEEE 26th International Conference on Intelligent Transportation Systems (ITSC)(2023)
摘要
In this paper, we address the problem of increasing the precision of dense direct stereo visual odometry methods. Dense methods need a dense depth map to generate warped images (virtual views) that will match with reference images if the estimated pose is good. Previous works have shown that generating the depth map by machine learning methods leads to very good odometry results. However, machine learning methods generate hallucinated depths even in areas where it is impossible to estimate the depth due to several reasons, like occlusions, homogeneous areas, etc. Generally, this produces wrong depth estimation that leads to errors in odometry estimation. To avoid this problem, we propose a new approach to generate multiple masks that will be combined to discard wrong pixels and therefore increase the accuracy of visual odometry. Our key contribution is to use the multiple masks not only in the odometry computation but also to improve the learning of the neural network for depth map generation. Experiments on several datasets show that masked dense direct stereo visual odometry provides much more accurate results than previous approaches in the literature.
更多查看译文
关键词
Visual Methods,Depth Map,Approaches In The Literature,Depth Estimation,Homogeneous Areas,Warped Image,Visual Odometry,Target Image,Max-pooling Layer,Difference Map,Pose Estimation,Camera View,Edge Area,Error Map,Percentage Of Pixels,Stereo Images,Temporal Images,Homogeneous Texture,Ground Truth Depth
AI 理解论文
溯源树
样例
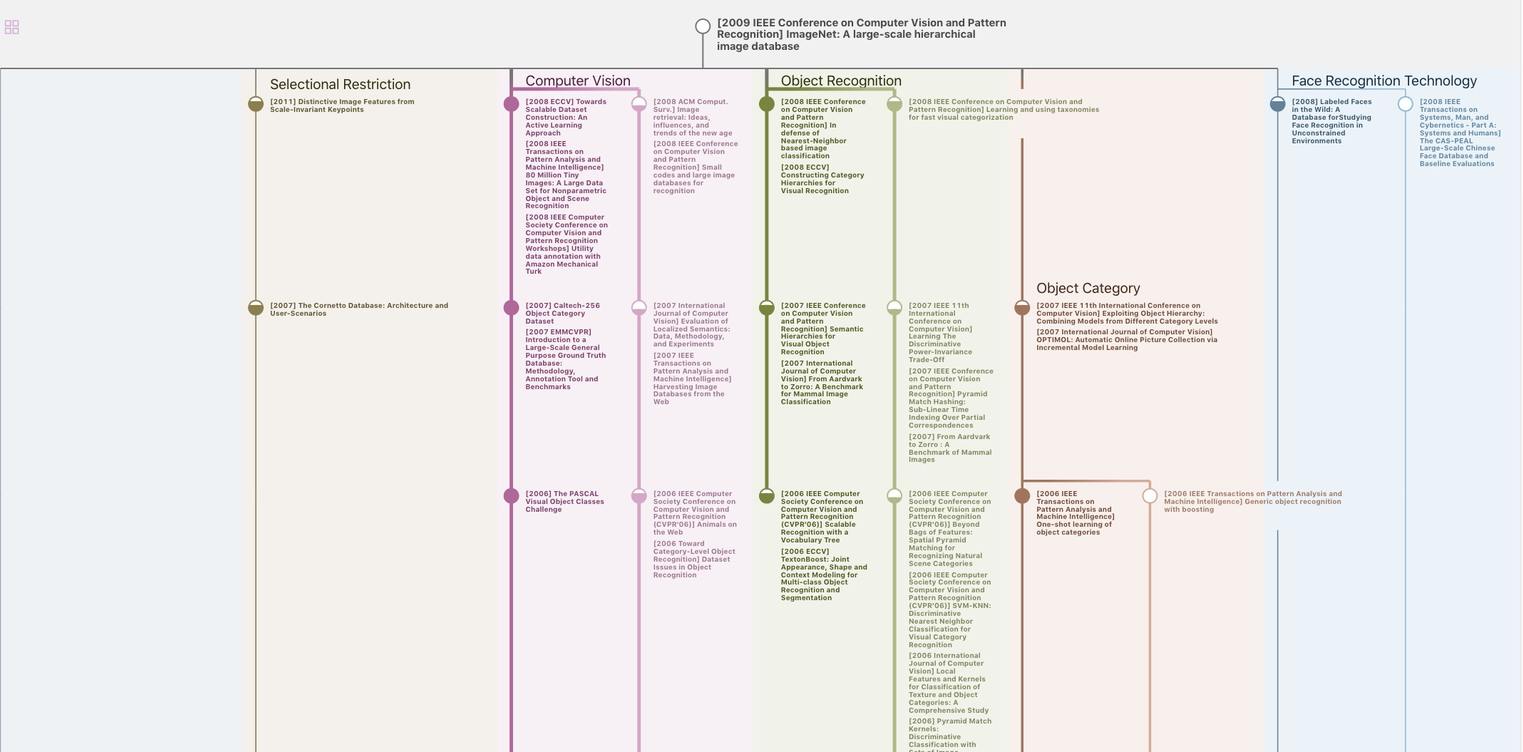
生成溯源树,研究论文发展脉络
Chat Paper
正在生成论文摘要