Real-time Incident Detection in Public Bus Systems Using Machine Learning.
2023 IEEE 26th International Conference on Intelligent Transportation Systems (ITSC)(2023)
摘要
Traffic incident detection (ID) systems use various sensors and communication technologies to identify, classify and locate traffic incidents in real time. For public bus systems, installing physical sensors such as cameras or radars is expensive. An alternative approach is to rely solely on GPS signals acquired in real-time from the bus fleet. In this work, we propose a machine learning approach for traffic incident detection trained using historical bus GPS data. The model detects incidents earlier, allowing public bus authorities and operators to mitigate the consequences of incidents. We also propose a methodology to generate incident labels from historical data based on sudden headway changes that involve multiple buses. We evaluate the model with traditional incident detection evaluation metrics: Detection Rate (DR), False Alarm Rate (FAR), and Mean Time to Detect (MTTD). The ML model achieved a DR of 79% for incidents lasting more than ten minutes, with an MTTD of 6.4 minutes and a FAR of 2.5%.
更多查看译文
关键词
Machine Learning,Public Bus,Traffic Accidents,Sudden Changes,Global Positioning System,False Alarm Rate,Headway,Traditional Metrics,Global Positioning System Data,ML Models,Global Positioning System Signal,Traffic Detection,Simulated Data,Highway,F1 Score,Daily Work,Travel Time,Average Speed,Traffic Flow,Classification Metrics,Low False Alarm Rate,Road Segments,Days In August,Urban Road,Traffic Conditions,Bus Stop,Minutes In Duration,Bus Network,Low Detection Rate
AI 理解论文
溯源树
样例
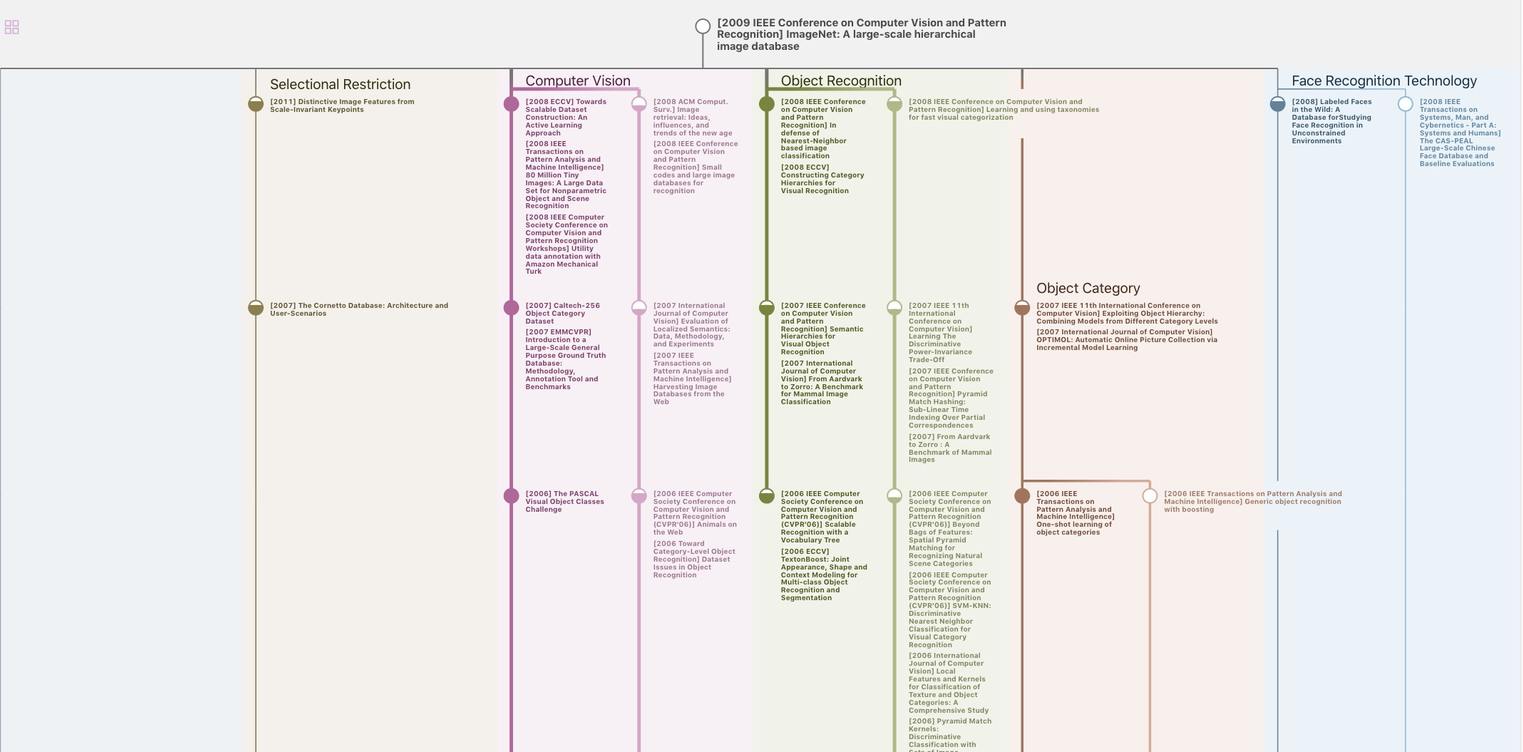
生成溯源树,研究论文发展脉络
Chat Paper
正在生成论文摘要