Maximum Entropy Inverse Reinforcement Learning Based on Frenet Frame Sampling for Human-Like Autonomous Driving.
2023 IEEE 26th International Conference on Intelligent Transportation Systems (ITSC)(2023)
摘要
Ensuring social acceptability is a key factor in the successful operation of autonomous vehicles. To achieve this, it is important to extract driving habits from expert human drivers. This paper proposes a method for training reward functions using maximum entropy inverse reinforcement learning based on Frenet frame sampling. The proposed method is applied to learn reward functions for the replication of human driver's lane-changing and velocity-keeping behaviors in multi-lane scenarios from human driving dataset, outperforming traditional methods based on Cartesian frame sampling. Converting the reward value of each sample into a probability distribution in the sample space allows for the provision of a convex space with human driving characteristics to the lower-level trajectory planner. These enhancements will help autonomous vehicles to select trajectories that align more with human driving habits. Experimental results show that the learned reward function can characterize human drivers' tendencies in multi-lane scenarios across multiple optimization metrics and exhibit strong interpretability. The proposed method effectively extract human driving behavior and habits under test conditions.
更多查看译文
关键词
Autonomous Vehicles,Maximum Entropy,Inverse Reinforcement Learning,Sample Space,Distribution In Space,Reward Function,Trajectory Planning,Human Drivers,Reward Learning,Effective Method,Least-squares,Learning Algorithms,Human Behavior,Continuous Distribution,Discrete Distribution,Traffic Light,Model Predictive Control,Numerical Algorithm,Planning Algorithm,Lane Change,Sample Trajectories,Quadratic Surface,Vehicle Behavior,Vehicle Acceleration,Maximum Entropy Principle,5th Order,Longitudinal Distance
AI 理解论文
溯源树
样例
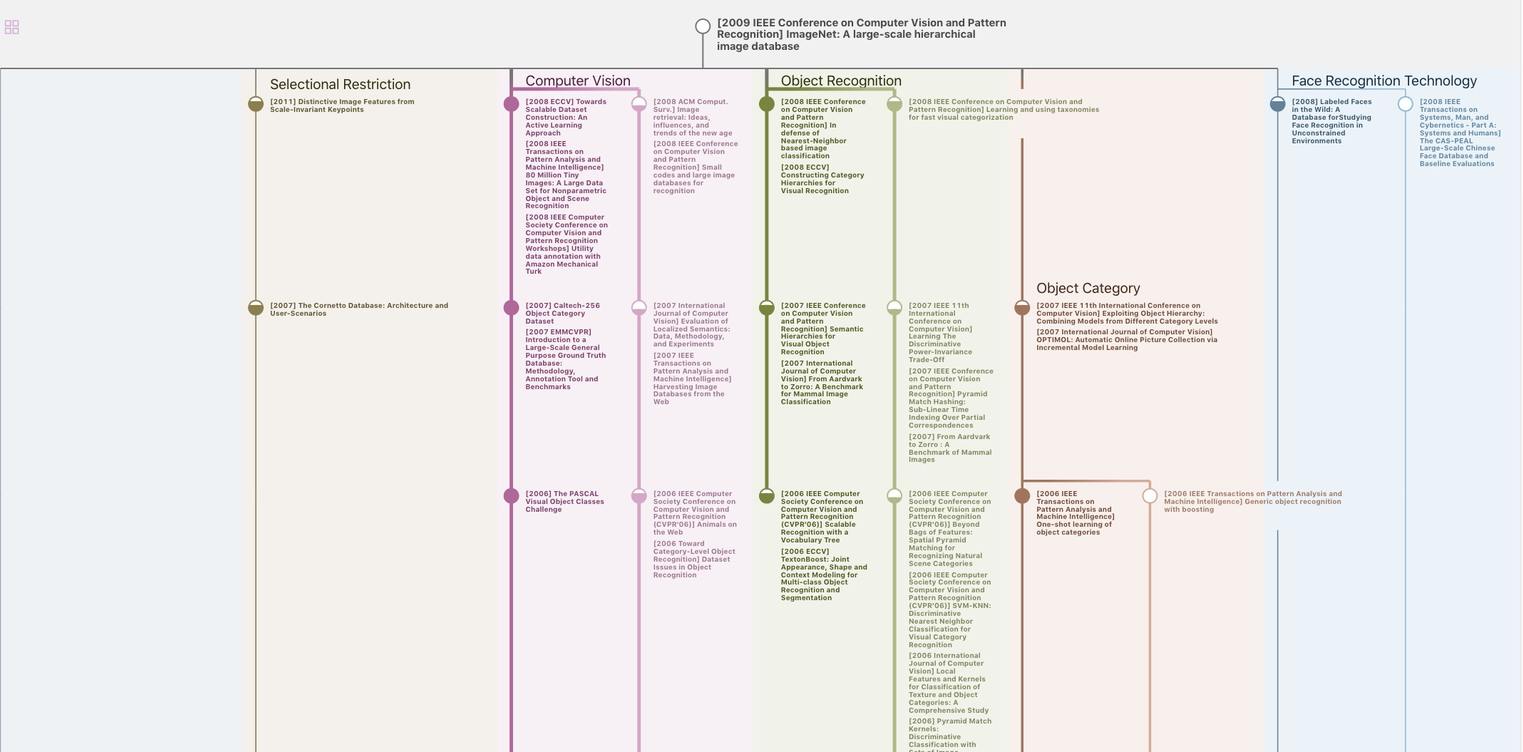
生成溯源树,研究论文发展脉络
Chat Paper
正在生成论文摘要