Learning From Drivers Patterns of Evasive Maneuvers in Case of Emergency Using Dynamic-Time-Warping-Based Clustering.
2023 IEEE 26th International Conference on Intelligent Transportation Systems (ITSC)(2023)
摘要
How to ensure safe takeovers in Level 3 automated driving has become a great concern. Among all the studies, the research question “What if drivers are not ready or fail to take over within required time?” seems to have been less emphasized. Therefore, the main objective of this research is to learn from drivers patterns of evasive maneuvers in emergent cases using dynamic-time-warping (DTW)-based k-means clustering. Forty-eight participants were recruited for a driving simulator study, and application of the algorithm resulted in three patterns of evasive maneuvers irrespective of scenarios, with Type I and III being the least and the most cautious ones. Specifically, drivers with Type I maneuvers preferred to overtake the vehicle ahead at higher speeds, lower accelerations, and quicker lane change maneuvers. Drivers with Type II maneuvers would slow down the vehicle before changing lanes, and then started to accelerate and perform the lane change. Drivers with Type III maneuvers tended to decelerate the vehicle to a low speed and kept at that speed for some time before they started to accelerate and finish the lane change. This helped to understand and design evasive maneuvers during automated driving and paved the way for the next research.
更多查看译文
关键词
Evasive Maneuvers,High Speed,K-means,Lane Change,Low Acceleration,Lane Change Maneuver,Time Series,Left Side,Centroid,Time Series Data,Cluster Centers,Effects Of Scenarios,Automated Vehicles,Speed Profile,Human Drivers,Steering Angle,Real Vehicle,Yaw Rate,Driver's License,Young Drivers,Time Series Sequence,Group Of Drivers
AI 理解论文
溯源树
样例
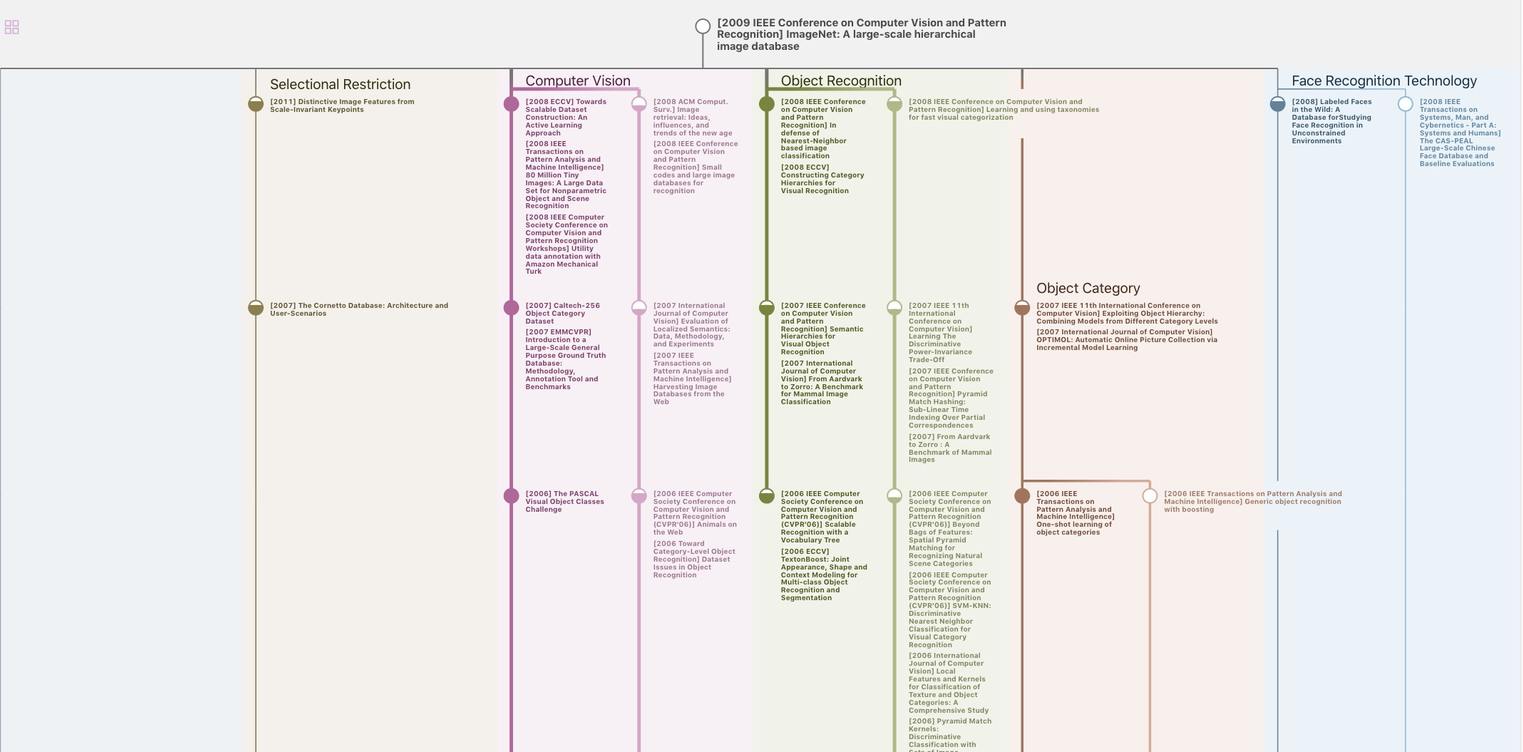
生成溯源树,研究论文发展脉络
Chat Paper
正在生成论文摘要