Time-varying Spatial Propagation of Brain Networks in fMRI data
biorxiv(2024)
摘要
Spontaneous neural activity coherently relays information across the brain. Several efforts have been made to understand how spontaneous neural activity evolves at the macro-scale level as measured by resting-state functional magnetic resonance imaging (rsfMRI). Previousstudies observe the global patterns and flow of information in rsfMRI using methods such as sliding window or temporal lags. However, to our knowledge, no studies have examined spatial propagation patterns evolving with time across multiple overlapping 4D networks. Here, we propose a novel approach to study how dynamic states of the brain networks spatially propagate and evaluate whether these propagating states contain information relevant to mental illness. We implement a lagged windowed correlation approach to capture voxel-wise networkspecific spatial propagation patterns in dynamic states. Results show systematic spatial state changes over time, which we confirmed are replicable across multiple scan sessions using human connectome project data. We observe networks varying in propagation speed; for example, the default mode network (DMN) propagates slowly and remains positively correlated to blood oxygenation level-dependent (BOLD) signal for 6-8 seconds, whereas the visual network propagates much quicker. We also show that summaries of network specific propagative patterns are linked to schizophrenia. More specifically, we find significant group differences in multiple dynamic parameters between schizophrenia patients and controls within four large-scale networks: default mode, temporal lobe, subcortical, and visual network. Individuals with schizophrenia spend more time in certain propagating states. In summary, this study introduces a promising general approach to exploring the spatial propagation in dynamic states of brain networks and their associated complexity and reveals novel insights into the neurobiology of schizophrenia.
### Competing Interest Statement
The authors have declared no competing interest.
更多查看译文
AI 理解论文
溯源树
样例
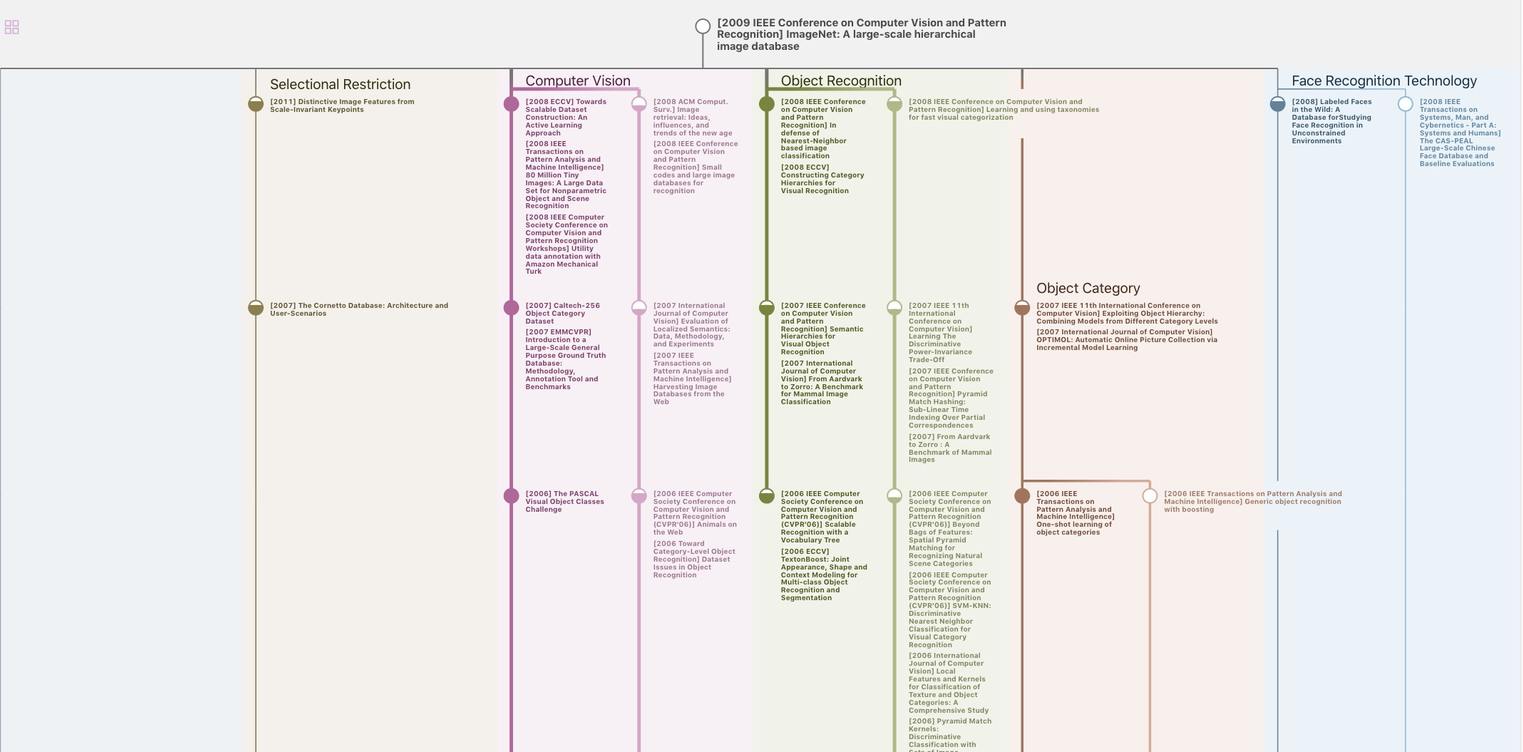
生成溯源树,研究论文发展脉络
Chat Paper
正在生成论文摘要