Improving SAR Automatic Target Recognition via Trusted Knowledge Distillation From Simulated Data
IEEE TRANSACTIONS ON GEOSCIENCE AND REMOTE SENSING(2024)
摘要
In recent years, significant research has been conducted on utilizing simulated data to support synthetic aperture radar automatic target recognition (SAR-ATR) based on deep learning (DL) techniques. By distilling the dark knowledge extracted from simulated samples, the quality of the learned representations on measured samples can be effectively enhanced. However, our study highlights an important oversight in previous works: unquestioning trust in all simulated samples inevitably introduces the part of dark knowledge that is detrimental to SAR-ATR performance. To address this issue, we introduce evidential learning to estimate the confidence degree of the model after inputting simulated samples, thereby assessing the validity of the dark knowledge to be distilled. Then, the simulated-measured knowledge distillation (KD) process will be carried out in a trusted manner. Specifically, we encourage the model to prioritize distilling the dark knowledge with higher validity while avoiding the influence of inferior knowledge through a dynamic confidence weighting (DCW) method. Additionally, we transform the standard logits-based KD loss function into a feature-based one, giving the proposed method the ability to plug-and-play. The above aspects constitute the proposed trusted simulated-measured KD method for SAR-ATR. Multiple comparative studies on the simulated and measured paired labeled experiment (SAMPLE) dataset demonstrate the effectiveness of our proposed method, which not only achieves superior performance but also maintains the desired computational complexity in the inference phase.
更多查看译文
关键词
Data models,Current measurement,Feature extraction,Pollution measurement,Adaptation models,Training,Knowledge engineering,Automatic target recognition,deep learning (DL),knowledge distillation (KD),simulated data,synthetic aperture radar (SAR)
AI 理解论文
溯源树
样例
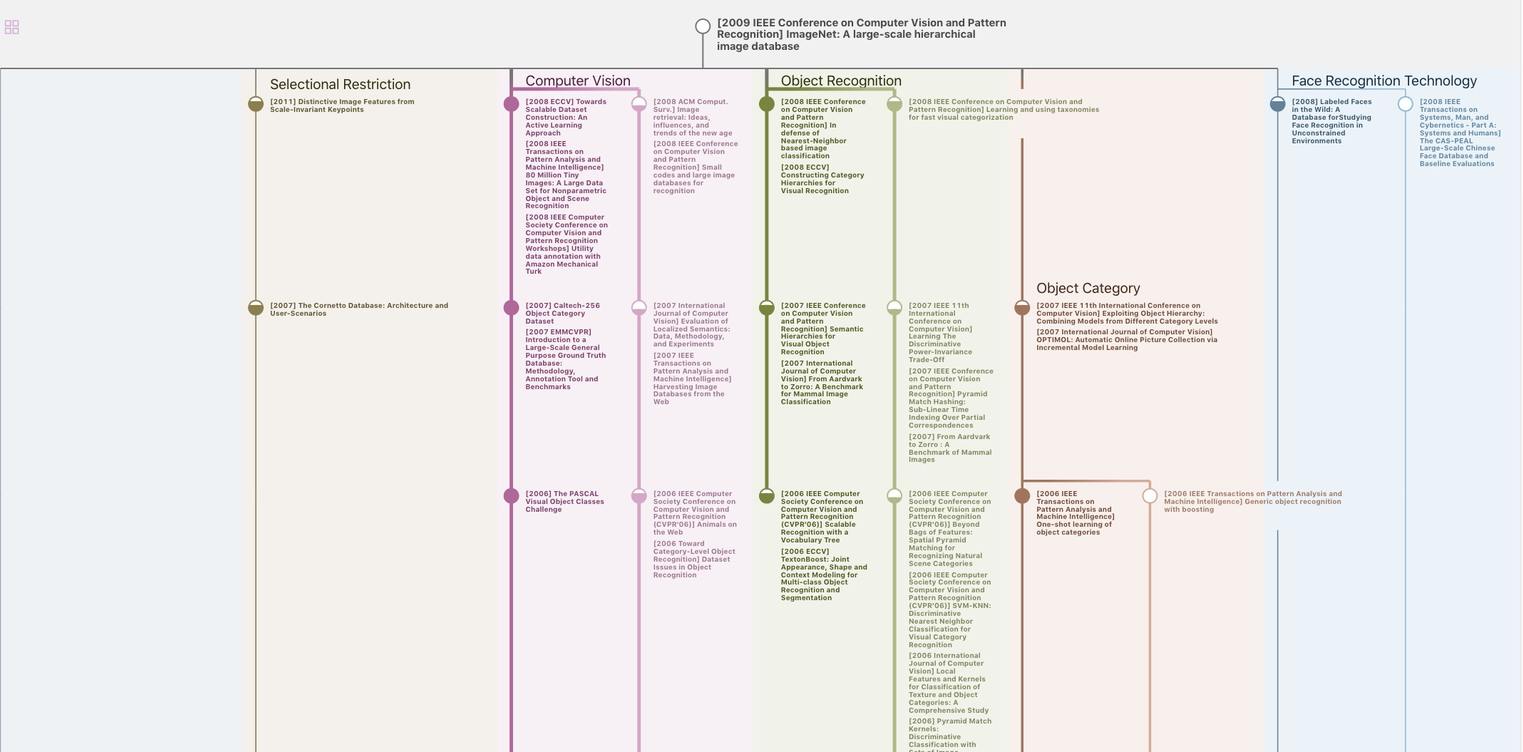
生成溯源树,研究论文发展脉络
Chat Paper
正在生成论文摘要