Colonoscopy Polyp Detection Using Bi-Directional Conv-LSTM U-Net with Densely Connected Convolution
KI - Künstliche Intelligenz(2024)
摘要
Several researchers have focused in recent years on improving the efficiency of abdominal diagnostics by segmenting colonoscopy images with machine learning techniques. Previously, colonoscopy images were manually segmented by experts in this field. This eventually became time-consuming work that was prone to human error. Advances in technology, such as increased computer power and the availability of libraries for manipulating colonoscopy images, enabled automated segmentation. In recent year, deep learning networks are using in medical segmentation field due to its versatility, high performance, high generalization capacity. Recently, new heights of effectiveness have been achieved in the process of medical image segmentation carried out by deep learning model. The process of medical image segmentation has been effectively improved by the application of deep learning models such as U-NET, RS-NET, and RS-NET++. In this study, we apply the benefits of U-Net, Bi-directional Conv-LSTM, and method of dense convolution. We applied these to the Kvasir-SEG and CVC-Clinic DB datasets and achieved the 0.92 and 0.93 dice coefficient respectively.
更多查看译文
关键词
Colonoscopy images,Machine learning,Deep learning,Medical segmentation,U-Net
AI 理解论文
溯源树
样例
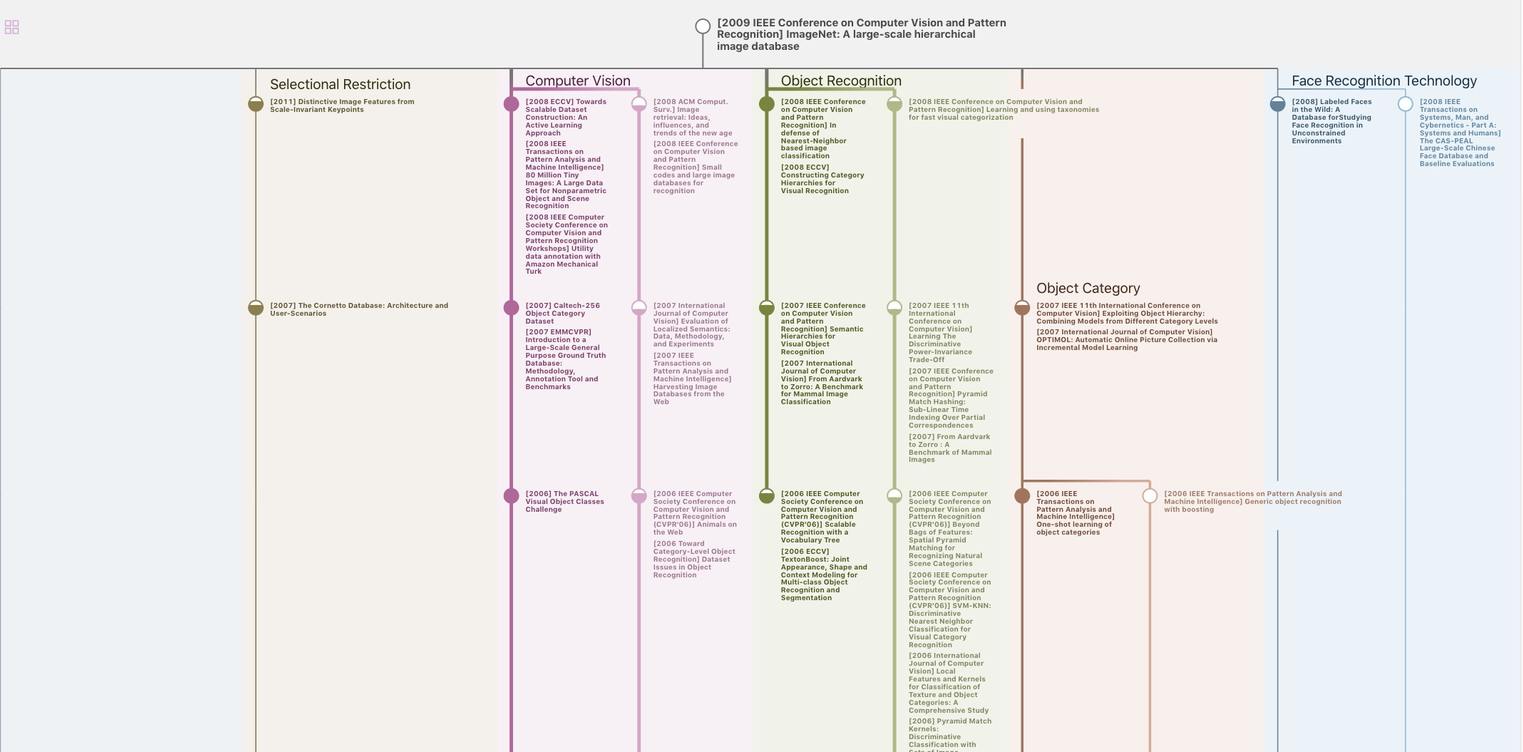
生成溯源树,研究论文发展脉络
Chat Paper
正在生成论文摘要