Exploring effect of scale dependency in LST downscaling – using convolution neural network-extreme learning machine (CNN-ELM)
Earth Science Informatics(2024)
摘要
Study of diurnal phenomena like urban head island requires land surface temperature (LST) at high spatial and temporal resolutions. Coarse spatial resolution thermal sensors have a high temporal resolution while in contrast fine spatial resolution sensors have a low temporal resolution. Hence, mathematical downscaling of coarse spatial resolution LST datasets is done for generating fine spatial and temporal resolution LST datasets. In previous studies the functional relationship between LST values and downscaling variables was assumed to be scale invariant and applied uniformly to all landcovers. The present study analyzed the scale invariance of the relationship using Convolution neural network-Extreme machine learning (CNN-ELM) algorithm. The study was done for summer, winter and spring datasets to ensure its transferability and the results were validated using Landsat retrieved LST. The study concluded that unlike as assumed in previous studies, functional relationship that exist at a coarse resolution, do not translate to finer resolutions due to scale-dependent effects, spatial heterogeneity, ecological factors, spatial autocorrelation and data noise. The relationship needs to recalibrated at finer resolution to account for these factors in local context. Secondly, when downscaling LST across different land covers, the downscaling variables should be unique to each land cover, instead of using the same downscaling variables for all land covers. The results of CNN-ELM and other traditional machine learning algorithms were also compared and it was found that CNN-ELM outperformed all the other algorithms.
更多查看译文
关键词
Land surface temperature,Convolution neural network,Extreme learning machine,Spectral indices,Down scaling,MODIS
AI 理解论文
溯源树
样例
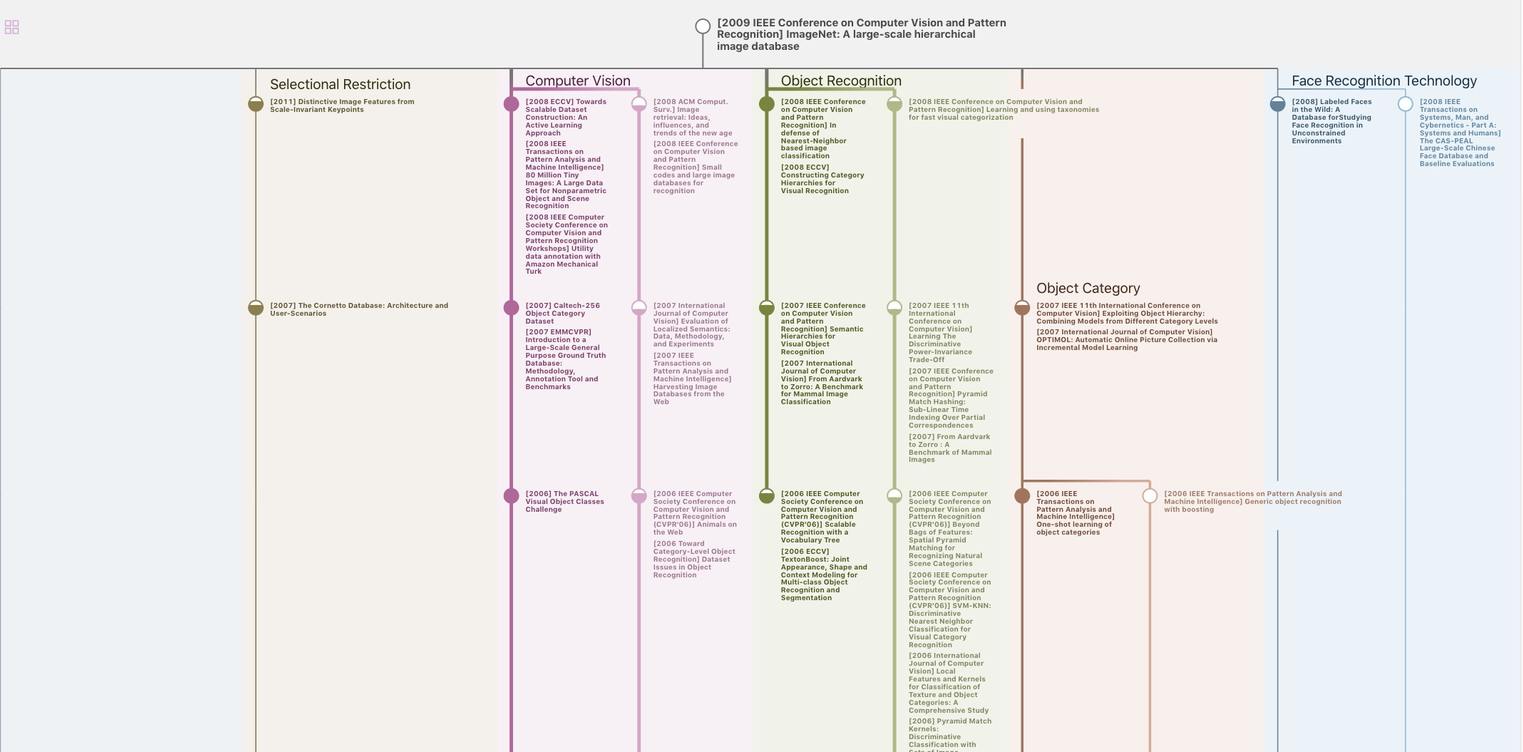
生成溯源树,研究论文发展脉络
Chat Paper
正在生成论文摘要