Transfer Operators from Batches of Unpaired Points via Entropic Transport Kernels
CoRR(2024)
摘要
In this paper, we are concerned with estimating the joint probability of
random variables X and Y, given N independent observation blocks
(x^i,y^i), i=1,…,N, each of M samples
(x^i,y^i) = ((x^i_j, y^i_σ^i(j))
)_j=1^M, where σ^i denotes an unknown permutation of i.i.d.
sampled pairs (x^i_j,y_j^i), j=1,…,M. This means that the internal
ordering of the M samples within an observation block is not known. We derive
a maximum-likelihood inference functional, propose a computationally tractable
approximation and analyze their properties. In particular, we prove a
Γ-convergence result showing that we can recover the true density from
empirical approximations as the number N of blocks goes to infinity. Using
entropic optimal transport kernels, we model a class of hypothesis spaces of
density functions over which the inference functional can be minimized. This
hypothesis class is particularly suited for approximate inference of transfer
operators from data. We solve the resulting discrete minimization problem by a
modification of the EMML algorithm to take addional transition probability
constraints into account and prove the convergence of this algorithm.
Proof-of-concept examples demonstrate the potential of our method.
更多查看译文
AI 理解论文
溯源树
样例
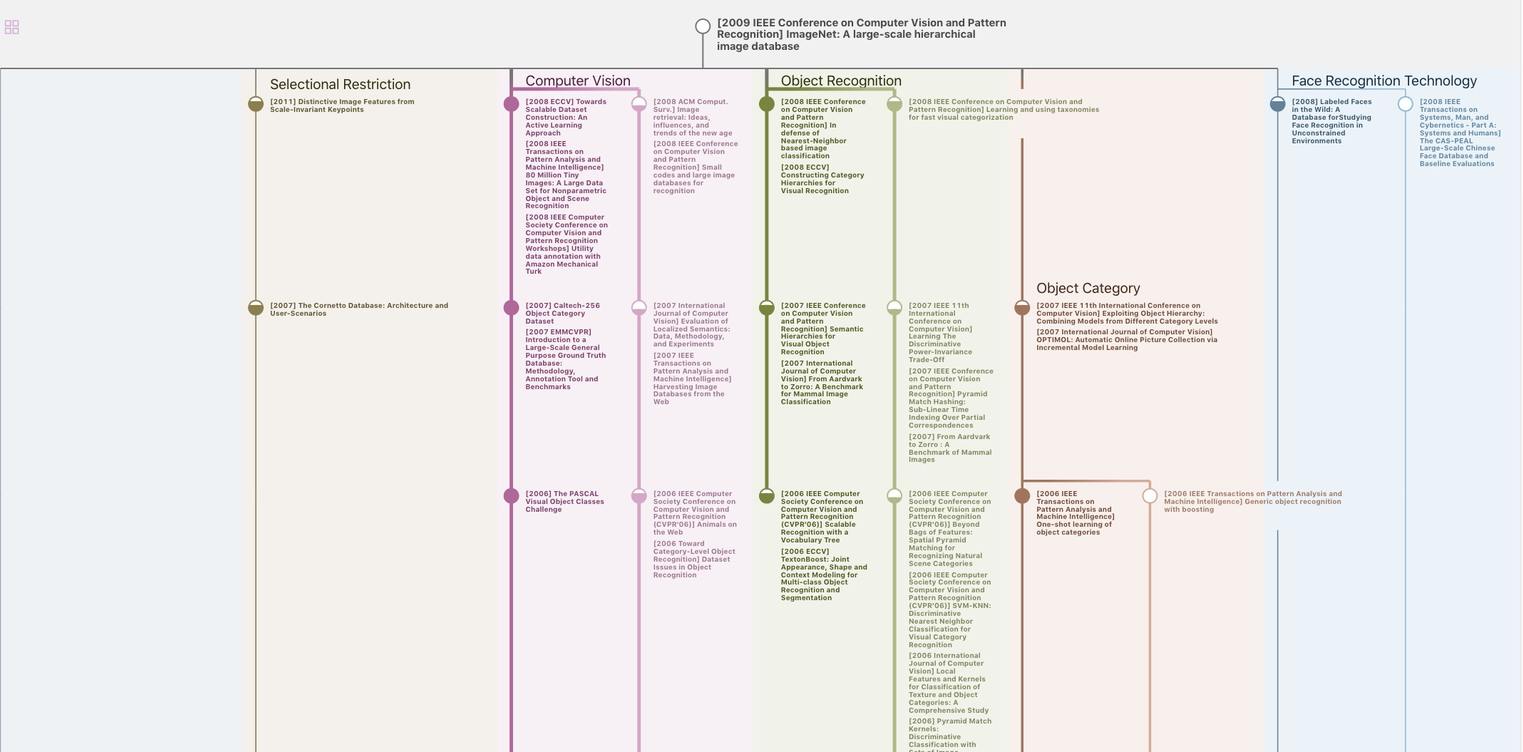
生成溯源树,研究论文发展脉络
Chat Paper
正在生成论文摘要