Intriguing Differences Between Zero-Shot and Systematic Evaluations of Vision-Language Transformer Models
CoRR(2024)
Abstract
Transformer-based models have dominated natural language processing and other
areas in the last few years due to their superior (zero-shot) performance on
benchmark datasets. However, these models are poorly understood due to their
complexity and size. While probing-based methods are widely used to understand
specific properties, the structures of the representation space are not
systematically characterized; consequently, it is unclear how such models
generalize and overgeneralize to new inputs beyond datasets. In this paper,
based on a new gradient descent optimization method, we are able to explore the
embedding space of a commonly used vision-language model. Using the Imagenette
dataset, we show that while the model achieves over 99% zero-shot
classification performance, it fails systematic evaluations completely. Using a
linear approximation, we provide a framework to explain the striking
differences. We have also obtained similar results using a different model to
support that our results are applicable to other transformer models with
continuous inputs. We also propose a robust way to detect the modified images.
MoreTranslated text
AI Read Science
Must-Reading Tree
Example
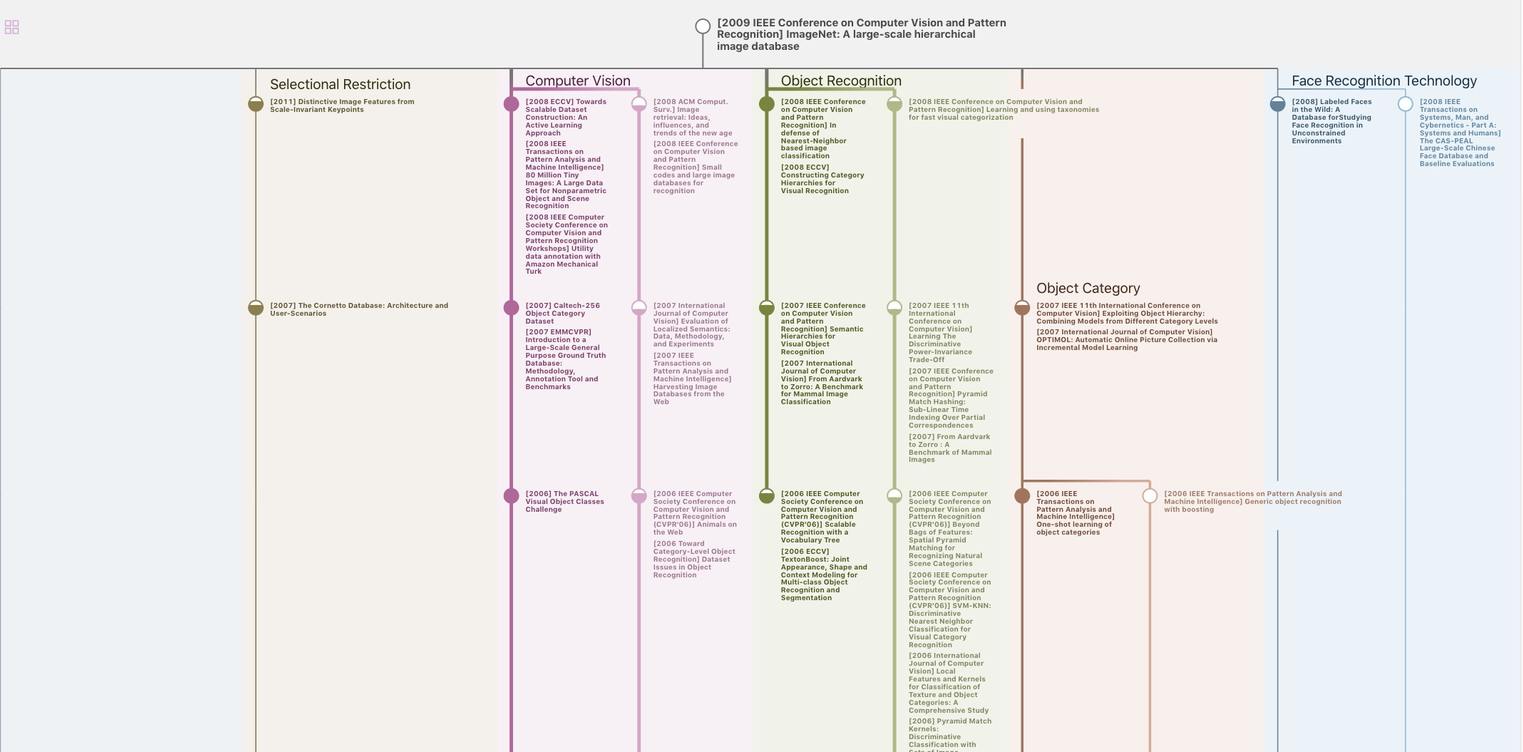
Generate MRT to find the research sequence of this paper
Chat Paper
Summary is being generated by the instructions you defined