A Novel Approach to Regularising 1NN classifier for Improved Generalization
CoRR(2024)
摘要
In this paper, we propose a class of non-parametric classifiers, that learn
arbitrary boundaries and generalize well.
Our approach is based on a novel way to regularize 1NN classifiers using a
greedy approach. We refer to this class of classifiers as Watershed
Classifiers. 1NN classifiers are known to trivially over-fit but have very
large VC dimension, hence do not generalize well. We show that watershed
classifiers can find arbitrary boundaries on any dense enough dataset, and, at
the same time, have very small VC dimension; hence a watershed classifier leads
to good generalization.
Traditional approaches to regularize 1NN classifiers are to consider K
nearest neighbours. Neighbourhood component analysis (NCA) proposes a way to
learn representations consistent with (n-1) nearest neighbour classifier,
where n denotes the size of the dataset. In this article, we propose a loss
function which can learn representations consistent with watershed classifiers,
and show that it outperforms the NCA baseline.
更多查看译文
AI 理解论文
溯源树
样例
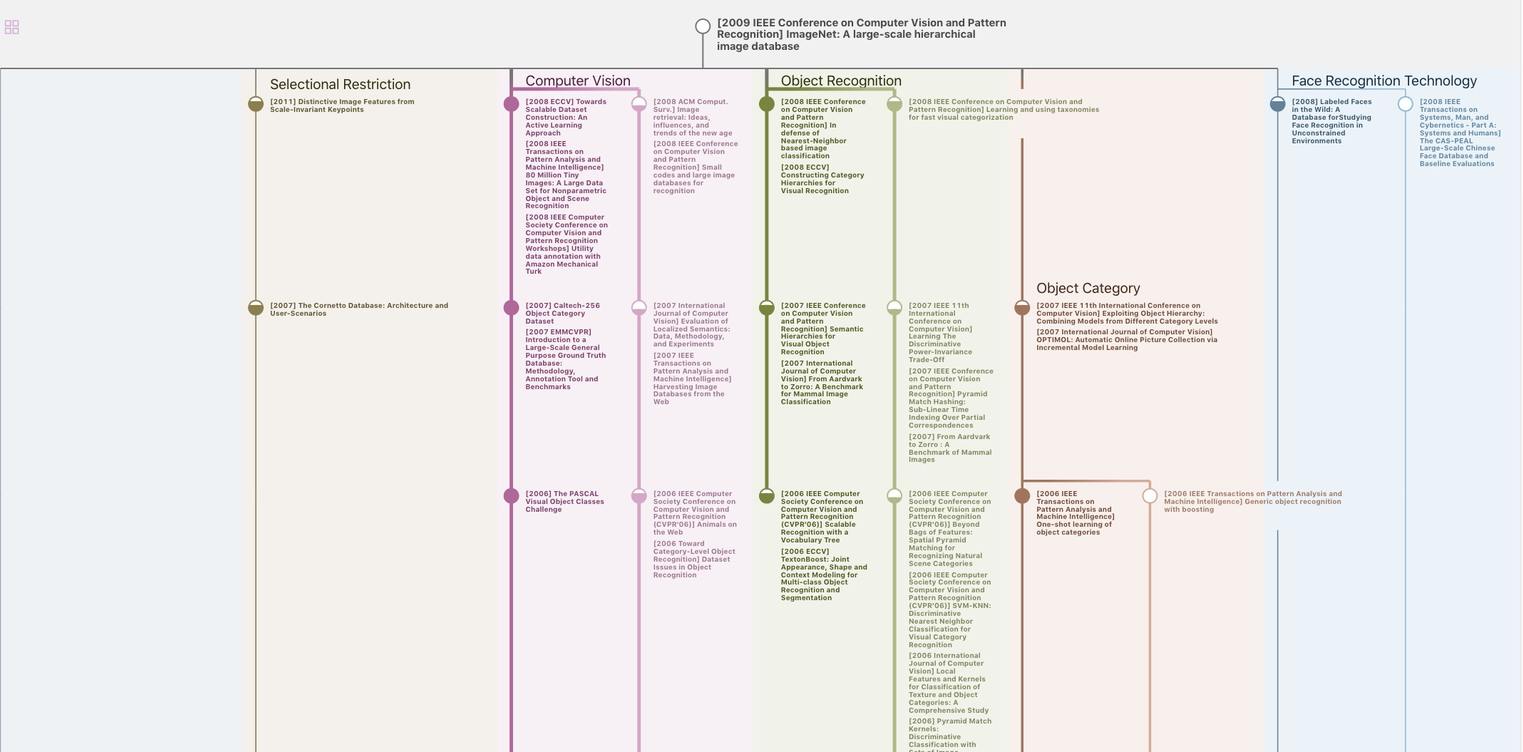
生成溯源树,研究论文发展脉络
Chat Paper
正在生成论文摘要