Online Structured Prediction with Fenchel–Young Losses and Improved Surrogate Regret for Online Multiclass Classification with Logistic Loss
CoRR(2024)
摘要
This paper studies online structured prediction with full-information
feedback. For online multiclass classification, van der Hoeven (2020) has
obtained surrogate regret bounds independent of the time horizon, or
finite, by introducing an elegant exploit-the-surrogate-gap
framework. However, this framework has been limited to multiclass
classification primarily because it relies on a classification-specific
procedure for converting estimated scores to outputs. We extend the
exploit-the-surrogate-gap framework to online structured prediction with
Fenchel–Young losses, a large family of surrogate losses including the
logistic loss for multiclass classification, obtaining finite surrogate regret
bounds in various structured prediction problems. To this end, we propose and
analyze randomized decoding, which converts estimated scores to general
structured outputs. Moreover, by applying our decoding to online multiclass
classification with the logistic loss, we obtain a surrogate regret bound of
O(B^2), where B is the ℓ_2-diameter of the domain. This bound is tight
up to logarithmic factors and improves the previous bound of O(dB^2) due to
van der Hoeven (2020) by a factor of d, the number of classes.
更多查看译文
AI 理解论文
溯源树
样例
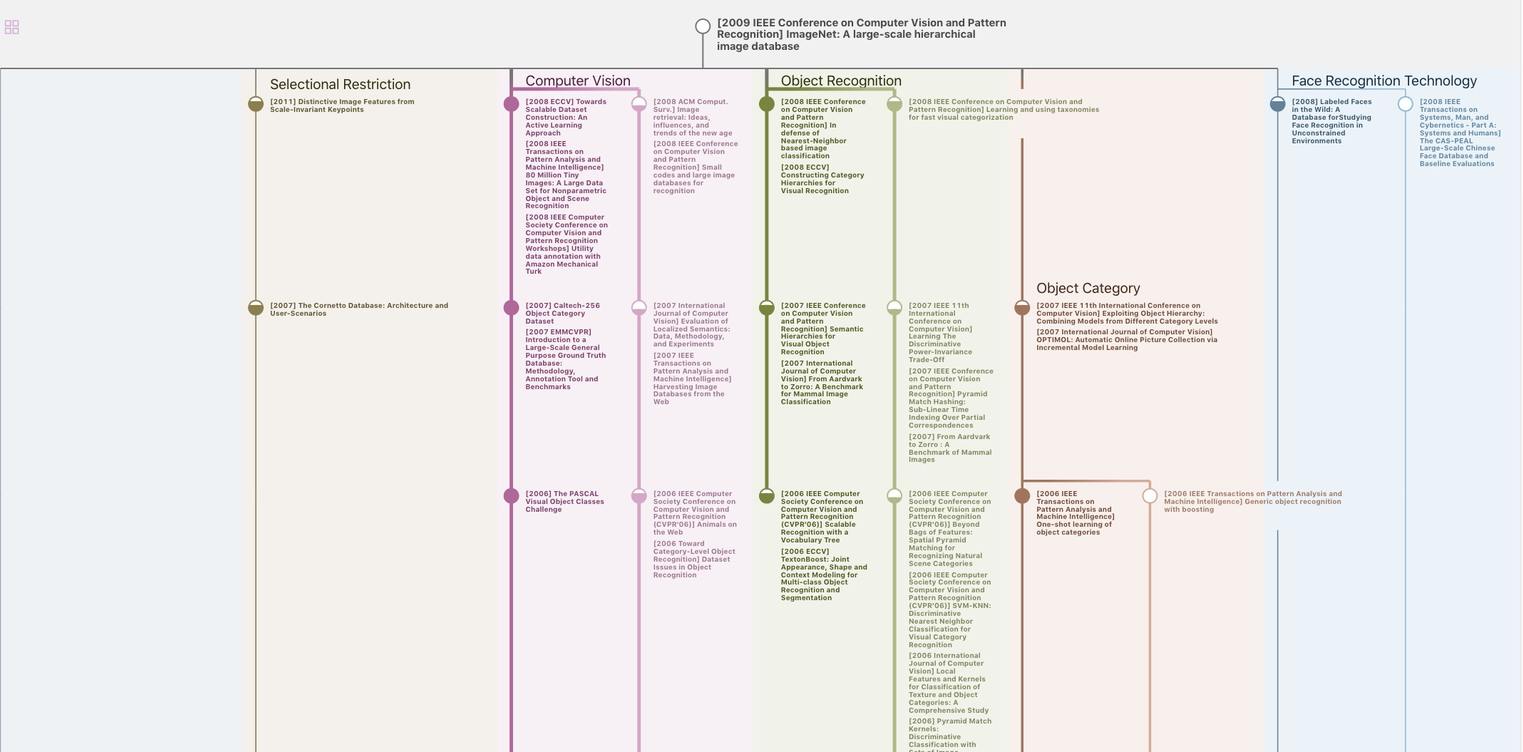
生成溯源树,研究论文发展脉络
Chat Paper
正在生成论文摘要