Detecting Low-Degree Truncation
CoRR(2024)
摘要
We consider the following basic, and very broad, statistical problem: Given a
known high-dimensional distribution D over ℝ^n and a
collection of data points in ℝ^n, distinguish between the two
possibilities that (i) the data was drawn from D, versus (ii) the data
was drawn from D|_S, i.e. from D subject to truncation by an
unknown truncation set S ⊆ℝ^n.
We study this problem in the setting where D is a high-dimensional
i.i.d. product distribution and S is an unknown degree-d polynomial
threshold function (one of the most well-studied types of Boolean-valued
function over ℝ^n). Our main results are an efficient algorithm when
D is a hypercontractive distribution, and a matching lower bound:
∙ For any constant d, we give a polynomial-time algorithm which
successfully distinguishes D from D|_S using O(n^d/2)
samples (subject to mild technical conditions on D and S);
∙ Even for the simplest case of D being the uniform
distribution over {+1, -1}^n, we show that for any constant d, any
distinguishing algorithm for degree-d polynomial threshold functions must use
Ω(n^d/2) samples.
更多查看译文
AI 理解论文
溯源树
样例
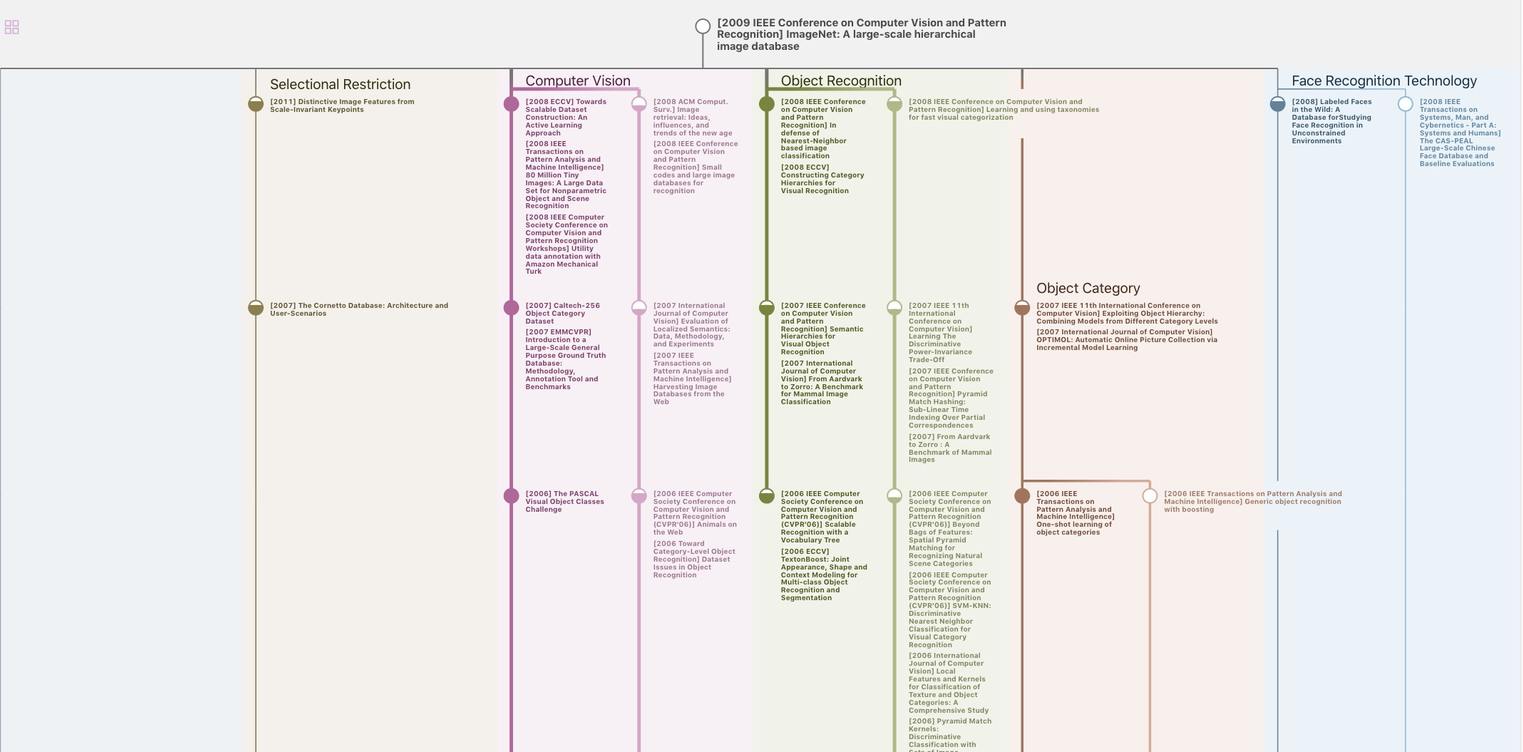
生成溯源树,研究论文发展脉络
Chat Paper
正在生成论文摘要